Estimation of efficacy of hiv nucleoside-analogue reverse transcriptase inhibitor (azt) via stochastic modeling
Proceedings of the 19th International Symposium on Mathematical Theory of Networks and Systems – MTNS 2010 • 5–9 July, 2010 • Budapest, Hungary
Estimation of efficacy of HIV nucleoside-analogue reverse transcriptase
inhibitor (AZT) via stochastic modeling
Samira Khalili, James M. Monaco, and Antonios Armaou
†,
Senior Member IEEE & Member AIChE, SIAM
Abstract— In this work, the mechanisms by which nucleoside-
In this study, a mechanistically informed model for the
analogue reverse transcriptase inhibitors (NRTIs), the most
intracellular interaction of HIV-1 and NRTIs was developed
common class of drugs used in the treatment of HIV-1, exert
which provides necessary tools to more accurately simulate
their antiviral effects are analyzed and methods in which thoseknown mechanisms could be employed to generate mathemat-
the progression of the HIV infection and its response to
ical models for drug efficacy in terms of measurable physical
treatment. The detailed explanation of the model and more
values are identified. Drug concentration is considered as a time
results can be find in [17]. The development of this type of
variant parameter which depends on the drug administration
mechanistic model can help guide future experimental inves-
time and dosage.
tigations by highlighting the key parameters that ultimatelydetermine the drug's efficacy. The efficacy was linked with
time-varying RTI triphosphate concentration from physio-
In order to describe the dynamics of the HIV infection,
logic data on intracellular triphosphate concentration max-
causative agent of AIDS, numerous mathematical models
imums and half lives, such as that collected in [15].
of varying detail have been proposed in the open liter-
II. REVERSE TRANSCRIPTION PROCESS
ature to capture different aspects of disease progression.
Currently, physically informed mathematical models have
The reverse transcription of viral RNA to DNA initiates af-
been developed for many aspects of the HIV reproductive
ter attachment of virus to host and fusion of viral components
cycle and the majority include drug efficacy as time-invariant
into the host cytoplasm. The process of reverse transcription
constants [1], [2], [3], [4]. Such mathematical models have
is not linear; up to three strands, a negative sense strand and
been employed to control disease progression and optimize
two halves of the positive sense strand, may be transcribed at
medication schedules [5], [3]. In [6], a model predictive
once. The process, as described in detail in [18] and shown in
control based method for determining optimal treatment
figure 1, includes these steps: (1) Host-provided tRNA binds
interruption of HAART was developed to schedule HIV
to the primer binding site (PBS) and (-) strand transcription
therapy. In [7] and [8], optimal medication strategies were
begins. (2) Upon reaching the 5' end of the RNA template,
scheduled for the primary stage of infection. The role of
the (-) strand is transferred to the 3' end. (3) Initiation of
mathematical modeling on the optimal control of HIV-1
the U(+) and D(+) strands begins as the (-) strand reaches
pathogenesis was reviewed in [9].
the two purine-rich primer sites. (4) After the U(+) and the
Studies such as [10], [11] use broad empirical models, as
(-) strand reach the the 5' end of their respective templates,
summarized by Greco, et. al. in [12]. These models estimate
another strand transfer occurs.This allows each to use the
the correlation between drug concentration and efficacy.
other as a template and continue transcription. (5) The ring
However, no models have attempted to reflect the class-
structure is opened as the (-) strand continues to the 5' end
specific mechanisms by which the major types of antiretro-
of the U(+) strand and the D(+) strand continues to the 5'
viral drugs exert their inhibitory effect. Nucleoside analogue
end of the (-) strand. (6) Transcription is complete once the
RT inhibitors (NRTIs) are prodrugs and they must undergo
U(-) strand displaces 100 bases of the D(+) strand to reach
three enzyme-catalyzed phosphorylations before reaching
the central termination site (CTS).
their active anabolite form. In the next step, the drugs must
After approximately 20000 reverse-transcriptase medi-
compete with natural deoxynucleotides to exert their effects.
ated nucleotide polymerization events, the complete double-
This prevents easy correlation of plasma concentrations with
stranded copy of viral DNA which is called a provirus is
instantaneous efficacy. Still, there are techniques for quickly
ready for being integrated into the host genome. During those
and directly measuring the intracellular concentration of
20000 nucleotide additions, NRTIs can exert their desired
NRTI triphosphates and natural dNTPs [13], [14], [15], [16].
NRTIs compete with natural nucleotides for addition into
S. Khalili and A. Armaou are with the Department of Chemical Engi-
the HIV reverse transcription complex. They inhibit proviral
neering, Pennsylvania State University, University Park, PA-16802
production through chain termination. However, addition of
†Corresponding author, email:
[email protected]. The author grate-
an NRTI molecule to the growing HIV genome does not
fully acknowledges the financial support of the National Science Foun-dation Career Award # CBET 06-44519 and American Chemical Society,
guarantee permanent chain termination in the intracellular
Petroleum Research Fund, grant PRF # 44300-G9.
environment. HIV reverse transcriptase enzyme can also
ISBN 978-963-311-370-7
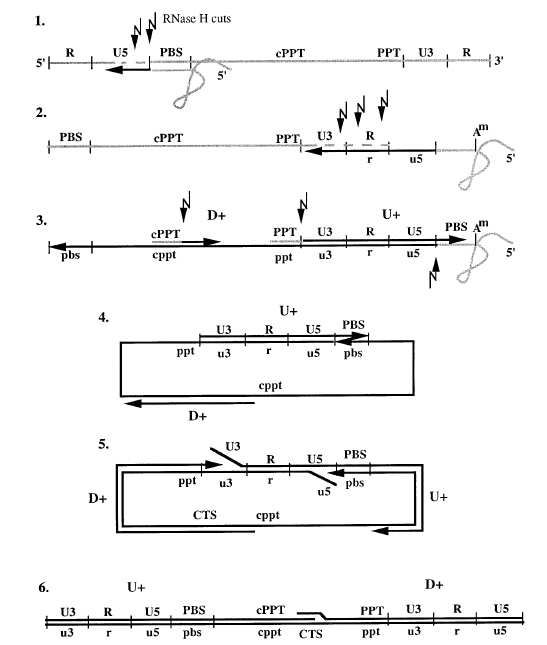
S. Khalili et al. • Estimation of Efficacy of HIV Nucleoside-Analogue Reverse Transcriptase Inhibitor (AZT) via Stochastic Modeling
the concentrations of free nucleotide and RT transcriptioncomplex. As such, the overall rates of nucleotide and NRTIaddition could be expressed as:
rate(+dN T P ) = krl(
[dN T P ][RT.DN A])
rate(+RT P ) = kirl(
[RT P ][RT.DN A])
In which KD and Ki are the dissociation constants for
the natural nucleotide and the drug, and krl and kirl are therespective rate constants for the rate limiting step. Equations1 and 2 can be used to calculate the probability of NRTItriphosphate addition:
rate(+RT P )
Fig. 1. An outline of critical reverse transcription events. Figure reproduced
rate(+RT P ) + rate(+dN T P )
from (Gotte and Li, 1999).
kirl [RTP]
catalyze the reverse reaction which results in the removal
of the terminal nucleotide.
A. Efficacy: measurement of drug effectiveness
which is equivalent to:
There are several measures used to determine the efficacy
of therapy: changes in T-cell counts, log-reductions in viral
KDkirl [RT P ]
titers, and IC
50 [11], [12], [13]. Here, the concept of efficacy
KDkirl [RT P ] + [dNT P ]
outlined by Perelson et al. [1] is used. In this definition, the
efficacy of an NRTI is the percentage by which it reduces
Since the intracellular concentration is a function of time,
the apparent rate of conversion of healthy T-cells to infected
p is time dependent as well. α, the relative affinity is then:
T-cells over the untreated case. This definition correlatesmost closely with drug IC
50, which is defined as the plasma
concentration of drug that results in a 50% reduction in the
appearance of infected cells over a set incubation period in
It can be thus concluded that the probability of NRTI
an in vitro culture.
addition can be expressed in terms of measurable physical
Addition of NRTI triphosphate occurs by the same mech-
constants. Consequently, if the concentration vs. time curves
anism as addition of deoxynucleotides. Hence, there are
for these two species may be predicted, then trends in
two possible "reactions" in the system: natural nucleotide
probability of NRTIs addition over time should be likewise
addition and NRTI addition. A good first step in determining
predictable. The effect of this probability on overall efficacy
efficacy would be to quantify the average probability that an
depends upon many additional factors, most importantly the
NRTI triphosphate is added at each "vulnerable" nucleotide
stability of the NRTI once included in the HIV genome.
in the genome. It is expected that this probability to be de-pendent upon three factors: the intracellular concentration of
B. Stability of NRTI as a chain terminator
NRTI triphosphate, the concentration of the deoxynucleotide
As mentioned earlier, RT can catalyze the reaction to
with which it competes, and the relative selectivity (α) of
remove chain-terminating NRTIs. The stability of the Ternary
the RT-Genome complex for the NRTI. The next step then is
Dead End Complex that forms varies enormously between
describing these quantities for the intracellular environment.
drugs. As reported by Isel et al. [23], HIV RT complexes
There are numerous experiments which directly measure
terminated by different NRTIs demonstrated varied results
the in vivo concentration of the triphosphate products for
when incubated in the presence of the next incoming nu-
a variety of RTIs [13], [14], [15], [19], [20], [21]. The
cleotide. Isel et al. kindly provided the data of +1 rescue
same techniques that are used to determine intracellular
(see [23], figure 3) for AZT which was studied in this work.
NRTI TP concentrations are also used to determine natural
The raw data was used to evaluate the parameters to equation
deoxynucleotide concentrations [16], [22], [21].
A(1−exp(−kextt)), where A is the amplitude of the reaction
The binding of nucleotide to the complex is a reversible
and kext is the apparent repair rate constant.
process. Since the conformational change is significantly rate
Presence of NRTIs results in the reduction in the number
limiting, the concentration of nucleotide-RT complex can be
of new cells that HIV can infect in a given period of time.
expressed in terms of its dissociation constant, as well as
This is achieved through two mechanisms:
Proceedings of the 19th International Symposium on Mathematical Theory of Networks and Systems – MTNS 2010 • 5–9 July, 2010 • Budapest, Hungary
Mechanism 1: Peripheral Blood Mononuclear Cells have
In which ²DC is the efficacy due to dead end complex
a set lifespan in vivo, and express certain natural defense
formation, p is the probability of NRTI addition each time
factors that may potentially degrade the HIV RT complex.
a vulnerable base is transcribed (expressed in equations 5
If an NRTI's TDEC is stable, then the reduction in infection
and 6) and B is the number of vulnerable bases in the
rate might be the result of a certain fraction of HIV fusions
complete genome. It is important to note that only formation
being effectively arrested during reverse transcription. The
of indefinitely stable TDECs (mechanism 1) contributes to
apparent rate of infections decreases by the percentage
the calculation of efficacy in the above equation. The effect
reduction in completed reverse transcriptions.
of time delay (mechanisms 2.I and 2.II) on efficacy needs
Mechanism 2: If TDECs are not stable, each addition
of an NRTI to the HIV genome would still represent a
There are a number of shortcomings to expression 8. As
period of holdup in the reverse transcription phase, extending
discussed earlier, the delay of reverse transcription (mech-
the time between viral fusion and active infection. When
anism 2), might be as important as permanent termina-
multiple NRTI additions occur, the holdup time may exceed
tion of the RT complex (mechanism 1) which can not
the time it would take for the host cell to die or clear the
be included in our proposed equation in a straightforward
RT complex. This, also will reduce the number of cells that
manner. Fortunately, stochastic simulation provides us with
become infected in a given period of time. We can consider
the ability of calculating the time delay associated with a
two types of time delay: time delay which exceeds the life
certain concentration as well as the permanent termination.
span of RNA in cytoplasm, which we define as mechanism
Furthermore, since addition of NRTI is a discrete event, and
2.I, and time delay which does not exceed the life span of
p(t) depends on the concentration of NRTI which changes
the RNA in cytoplasm, which we define as mechanism 2.II.
with time, stochastic simulation can provide a more accurate
In other words, the source of NRTI efficacy is likely due
estimation of the probability of NRTI addition over the
to a combination of both mechanisms (1) and (2): NRTI
transcription process. The results of stochastic simulation
induces a moderate delay in reverse transcription, increasing
will be discussed in detail in section IV.
the chance of a viral fusion failing to produce an activelyinfected T-cell while causing those that do succeed to take
III. STOCHASTIC MODEL OF REVERSE TRANSCRIPTION
longer in doing so. Such a process defies reduction to a
simple mathematical description, but is approachable via in
An investigation of the problem might persuade one to
sillico stochastic modeling of the individual events that occur
wonder if the overall delay in reverse transcription resulting
during reverse transcription.
from NRTI could be calculated simply by taking the expected
C. Estimation of NRTI efficacy
number of NRTI additions per genome and multiplying bythe expected time that each chain termination will last.
In [11], plasma time-course of drug concentration is de-
Unfortunately, As discussed in section II, the reverse tran-
scription is a nonlinear process. The inhibition of one strand
C(T ) + t (C
will not necessarily prevent the others from being elongated.
C(t) =
max − C(T ))
0 ≤ t ≤ tp
Cmax exp(−ω(t − tp))
tp < t < T
As such, the location and time when NRTI addition occurs
affects just how long it delays the entire process. The
p and T represent dosing interval (administration
proposed stochastic model accurately simulates the entire
time) and time to peak, respectively; and ω = log(2) where
process of reverse transcription on an event-by-event basis,
T1/2 is plasma half life of the drug. It was assumed that the
"building" the genome one base at a time with probabilistic
drug concentration peaks instantaneously when the dose is
addition of NRTIs followed by stochastic analysis of the
taken, i.e., tp = 0.
ultimate path to chain-terminator removal. Figure 2 shows
In this work, we have attempted to develop a more accurate
the algorithm of the simulation. Specifically this algorithm
model which considers not only changes in drug concentra-
is based on Gillespie's next reaction algorithm to the RT
tion, but also specific drug properties like the probability of
process. The "nonlinear guideline" in figure 2 eludes to
forming dead end complex. Each time the idealized NRTI
choosing which strand of the DNA the simulation is currently
is added to the growing HIV provirus, it is either removed
transcribing. It is a sequence of events which is described in
by pyrophosphorolysis or successfully forms a stable dead-
section II and graphically presented in figure 1. A random
end complex. Under cellular conditions, both phosphorolysis
sequence was chosen from the NIH HIV sequence database
and TDEC formation can be considered first-order reactions
for simulation. For each drug one million simulation runs
that compete for NRTI-terminated RT complexes. Thus, the
were used to compute the time distributions as well as
formation of a stable complex can again be calculated as a
percentage of TDEC formation.
propensity function in terms of these rates, yielding someprobability pTDEC that a dead end complex will form.
IV. RESULTS AND DISCUSSIONS
The overall probability of permanent elimination of the RT
In this section, the simulations' results are discussed.
complex, which is equivalent to efficacy in this case, can be
The reverse transcription (without considering the effect of
inhibitors) is a stochastic process. As a result, the reverse
1 − ²DC = (1 − p · pTDEC)B
transcription process acquires a time distribution rather than
S. Khalili et al. • Estimation of Efficacy of HIV Nucleoside-Analogue Reverse Transcriptase Inhibitor (AZT) via Stochastic Modeling
In Table I summary of the simulations results are pre-
sented. Investigating the one million runs for each case,four different outcomes can be identified: NRTI additionswhich lead to dead end complex formation (mechanism 1),
Based on the transcription recipe,
choose the block (A, G, C, or T)
NRTI additions which delay the process so that the overall
to be transcribed
time exceeds the life span of RNA (mechanism 2.I), NRTIadditions which delay the overall process but not enough toexceed the life span of RNA (mechanism 2.II), and finally no
Is it a vulnerable
block to the simulated
NRTI addition. Although mechanism 2.II results in delaying
the reverse transcription process, viral DNA will be produced
Copy the block and add to the chain
and eventually integrated and transcribed to generate new
Calculate "p" based on
Update concentration
virus particles. In other words, mechanism 2.II and no NRTI
Generate a random number "R1"
Move to the next block
addition can be considered as "unsuccessful inhibition" casesand mechanism 1 and 2.II as "successful inhibition" cases.
Consequently, we defined the successful inhibition or the
overall efficacy of stochastic simulation, ²si%, as the sum
of mechanism 1 (²DC%) and mechanism 2.I. ²si% is shown
Add RTI to the chain.
Generate a random number "R2"
in the last column in Table I.
Is R2 < p(TDEC)?
EFFECT OF CONCENTRATION AND HALF LIFE ON AZT INHIBITORY
Calculate the RTI dissociation
time based on exponential dist.
EFFECT FOR WILD TYPE VIRUS. THE OVERALL EFFICACY ²si% IN THE
Advance time.
Update concentration
LAST COLUMN IS THE SUM OF ²DC % AND THE PERCENT OF 2.I. NO
Move to the next block
NRTI ADDITION PERCENTAGE AND 2.II PERCENTAGE REPRESENT THE
FAILURE OF THE NRTI TO INHIBIT REVERSE TRANSCRIPTION.
Algorithm of the stochastic simulation. p and pT DEC show the
probability of NRTI addition to the chain and the probability of forming
a dead end complex, respectively. "Nonlinear guideline" refers to which
strand of the DNA is currently being considered as described in [18] andfigure 1.
a precise time: tRT = 221.14 min with variance of 5.48 min.
The distribution is shown in figure 3.
A. Effect of reverse transcription inhibitors on reverse tran-
scription time
In this study AZT which is T-analogue is simulated. The
parameters used for simulation are: α = 10, k
ext = 1.76 ×
10−3[1/s], and p
T DEC = 8.1%.
Although the value of affinity is significant, one should
note that the concentrations of drug and the natural nu-cleotide play an important role in determining the probability
It was assumed that the administration time is every eight
of inclusion of NRTI in the chain. Once included in the chain,
hours, and two different half lives: 2 and 4 hours were
it is more beneficial if 1) the extension time is long (small
investigated. As discussed before in section II-C, a longer
half life means higher p and consequently higher efficacy.
ext) and 2) the probability of forming TDEC, pT DEC is
Efficacy also increases with drug concentration as expected.
Half-life and the initial drug concentration are two very
In order to show the effect of drug administration time as
important inputs for the simulations. Because of the variety
well, at, we chose a value for maximum concentration (here
in reported half-life values in literature, various values were
Cmax = 2) and simulated six cases: adminstration time of 4
simulated: h = 1, 2, 4[hr]. The IC50 values in literature for
and 8 hours; and half lives of 1, 2, and 4 hours (the results
AZT are quite similar and the value of IC50 = 10[nM] was
are not shown here). It is interesting to note that when the
used in the simulations. It is important to emphasize here
half life is very short (comparing to at), ²si is slightly larger
that Cmax, which is the maximum drug concentration at the
than ²DC; however, when the half life is larger, the difference
time of administration, is the input for the simulation, not
between ²si and ²DC becomes significant.
the IC50. However, Cmax should be chosen considering the
It is also interesting to investigate the time distribution of
IC50. Six different ratios of Cmax to IC50 were simulated:
reverse transcription process under treatment and compare
(0.1, 0.25, 0.5, 1, 2, 4).
it with no treatment time distribution. It is important to
Proceedings of the 19th International Symposium on Mathematical Theory of Networks and Systems – MTNS 2010 • 5–9 July, 2010 • Budapest, Hungary
note that the TDEC forming cases were not considered
VI. ACKNOWLEDGMENT
in time distribution graphs. No NRTI addition cases also
Financial support from NSF, CAREER Award #CBET 06-
were not included in order to see the effect of time delays
44519, is gratefully acknowledged. The authors would like to
more vividly. One may need to consider the TDEC and
thank Dr. Isel for very helpful discussions and also providing
no NRTI addition percentages with the graphs to gain an
the experimental data necessary for this study.
overall insight of each drug. Data with
max = 0.5 were
chosen to create figure 3. Comparing the time distributionsof no treatment and AZT treatment in figure 3, it can be
[1] A. S. Perelson and P. W. Nelson, "Mathematical analysis of HIV-1
dynamics in vivo," SIAM review, vol. 41, no. 1, pp. 3–44, 1999.
observed that the AZT distribution is shifted to the right
[2] A. A. Ding and H. Wu, "Relationships between antiviral treatment
towards longer times. This is the effect of (single or multiple)
effects and biphasic viral decay rates in modeling HIV dynamics,"
NRTI inclusions into the chain and the time needed for their
Math. Biosci., vol. 160, no. 1, pp. 63–82, 1999.
[3] S. J. Snedecor,
"Comparison of three kinetic models of HIV-1
infection: Implications for optimization of treatment," J. Theor. Biol.,vol. 221, pp. 519–541, 2003.
[4] M. A. Nowak, S. Bonhoeffer, and et al, "Anti-viral drug treatment:
Dynamics of resistance in free virus and infected cell populations," J.
Theor. Biol., vol. 184, no. 2, pp. 205–216, 1997.
[5] D. Kirschner, S. Lenhart, and S. Serbin, "Optimal control of the
chemotherapy of HIV," J. Math. Biol., vol. 35, pp. 775–792, 1997.
[6] R. Zurakowski and A. R. Teel, "A model predictive control based
scheduling method for HIV therapy," J. Theor. Biol., vol. 238, pp.
exceeding lifespan
368–382, 2006.
[7] S. Khalili and A. Armaou, "Sensitivity analysis of HIV infection
response to treatment via stochastic modeling," Chem. Eng. Sci., vol.
63, pp. 1330–1341, 2008.
probability density
[8] S. Khalili and A. Armaou, "An extracellular stochastic model of early
HIV infection and the formulation of optimal treatment policy," Chem.
Eng. Sci., vol. 63, pp. 4361–4372, 2008.
[9] M. Joly and J. M. Pinto, "Role of mathematical modeling on the
optimal control of HIV-1 pathogenesis.," AICHE J., vol. 52, pp. 856–
[10] Y. X. Huang and S. L. Rosenkranz, "Modeling HIV-1 dynamics and
antiviral response with consideration of time-varying drug exposure,
Distribution of time to complete reverse transcription process
adherence, and phenotypic sesitivity," Math. BioSci., vol. 184, pp.
with no drug (solid black line) and under treatment with AZT (dashed red
165–186, 2003.
line). Only mechanisms 2.I and 2.II are considered. The dotted vertical line
[11] L. M. Wahl and M. A. Nowak, "Adherence and drug resistance:
represents the life span of RNA in cytoplasm.
predictions for therapy outcome," Proceedings of the Royal Society ofLondon Series B-Biological Sciences, vol. 267, pp. 835–843, 2003.
[12] W. R. Greco and G. Bravo, "The search for synergy - a critical review
from a response-surface perspective," Pharmacolo. Reviews, vol. 47,
pp. 331–385, 1995.
[13] J. Balzarini and P. Herdewijn,
"Differential patterns of intracel-
In conclusion, we showed that stochastic simulation can
lular metabolism of 2 , 3 -didehydro-2 , 3 -dideoxythymidine and
be employed to investigate the mechanism of inhibition of
3 -azido-2 , 3 -dideoxythymidine, 2 potent anti-human immunodefi-
the RT process. It helped us investigate the formation of
ciency virus compounds," J. Bio. Chem., vol. 264, pp. 6127–6133,1989.
TDEC as well as time delay associated with NRTI inclusion.
[14] F. Becher and A. Pruvost,
"A strategy for liquid chromatogra-
The most important challenge in widely employing this
phy/tandem mass spectrometric assays of intracellular drugs: Applica-
model is the lack of various necessary quantitative data for
tion to the validation of the triphosphorylated anabolite of antiretrovi-rals in peripheral blood mononuclear cells," J. Mass Spect., vol. 38,
different drugs. In vitro experiments could be employed to
pp. 879–890, 2003.
decipher what will take place in vivo. Due to the fact that
[15] F. Becher and R. Landman, "Monitoring of didanosine and stavu-
mutation rate of HIV is quite high and resistance to treat-
dine intracellular trisphosphorylated anabolite concentrations in HIV-infected patients," AIDS, vol. 18, pp. 181–187, 2004.
ments decreases the effect of treatment, it is also important
[16] A. Meyerhans and J. P. Vartanian, "Restriction and enhancement of
to quantify the necessary parameters of resistant strains.
human-immunodeficiency-virus type-1 replication by modulation of
Currently, quantitative data which can be used in mathe-
intracellular deoxynucleoside triphosphate pools," J. Virol., vol. 68,pp. 535–540, 1994.
matical simulations are scarce and limited to a few types
[17] S. Khalili, J. Monaco, and A. Armaou, "Development of a stochastic
of currently used drugs. Once accurate data are available,
model for the efficacy of nrtis using known mechanisms of action,"
such predictions for combinations of NRTIs will be feasible;
Journal of Theoretical Biology, vol. in press, 2010.
[18] M. Gotte and X. G. Li, "HIV-1 reverse transcription: A brief overview
we will then become able to ascertain the effectiveness of
focused on structure-function relationships among molecules involved
HAART through simulation. For future studies, we seek to
in initiation of the reaction," Arch. Biochem. & Biophys., vol. 365,
include this model in an intracellular model of HIV infection
pp. 199–210, 1999.
[19] C. Y. Solas and F. Li, "Intracellular nucleotides of 2',3'-deoxy-3'-
which initiates by attachment of a virus to the host to
thiacytidine in peripheral blood mononuclear cells of a patient infected
production and budding of new virions. Such an exclusive
with human immunodeficiency virus," Antimicrob. Agents & Chemo.,
model provides the opportunity to investigate the combined
vol. 42, pp. 2989–2995, 1998.
[20] C. V. Fletcher and E. P. Acosta, "Concentration-controlled zidovudine
effects of different classes of HIV drugs such as Protease
therapy," Clinical Pharmaco. & Therapeutics, vol. 64, pp. 331–338,
Inhibitors, Fusion Inhibitors, and Integrase Inhibitors.
S. Khalili et al. • Estimation of Efficacy of HIV Nucleoside-Analogue Reverse Transcriptase Inhibitor (AZT) via Stochastic Modeling
[21] W. Y. Gao and T. Shirasaka, "Differential phosphorylation of azi-
dothymidine, dideoxycytidine, and dideoxyinosine in resting and acti-vated peripheral blood mononuclear cells," J. of Clinical Investigation,vol. 91, pp. 2326–2333, 1993.
[22] J. D. Chi and A. Jayewardene, "A direct determination of thymidine
triphosphate concentrations without dephosphorylation in peripheralblood mononuclear cells by lc/ms/ms," AIDS, vol. 26, pp. 829–836,2001.
[23] C. Isel and C. Ehresmann, "The emergence of different resistance
mechanisms toward nucleoside inhibitors is explained by the propertiesof the wild type HIV-1 reverse transcriptase," J. Biol. Chem., vol. 276,pp. 48725–48732, 2001.
Source: http://www.conferences.hu/mtns2010/proceedings/Papers/423_291.pdf
BUREAU OF QUALITY ASSURANCE PROGRAM REPORT FOR Milton Girls Juvenile Residential Facility Gulf Coast Youth Services (Contract Provider) 5570 E. Milton Road Milton, FL 32583 Review Date(s): November 16-18, 2010 PROMOTING CONTINUOUS IMPROVEMENT AND ACCOUNTABILITY
Pharmacological Treatment of Schizophrenia by Richard H. Hall, 1998 Drugs The primary theory of schizophrenia at the neurotransmitter level, the dopamine hypothesis, was formed as the result of an accidental, yet dramatic event in the history of the treatment of schizophrenia. In the 1940s a French surgeon, Henri Laborit, began using a drug in an effort to reduce post operative shock in his patients. Laborit found that the drug reduced patients' anxiety without impairing cognitive functioning. This finding lead French drug companies to explore similar drugs, and as a result Chlorpromazine was developed. This drug was tested on a variety of mental disorders and did not seem to have much affect on disorders such as depression, but had dramatic effects on schizophrenia. As a result the "drug revolution" in the treatment of schizophrenia was born, and the result was profound. Schizophrenic patients who had been "out of touch" from consensus reality for years, were suddenly able to function effectively in society. As we'll discuss below, the effects do not occur for all schizophrenics, and there were side effects. Nevertheless, the introduction of drugs like chlorpromazine increased practitioners' ability to treat schizophrenia more dramatically than anything before, or anything that has come sense. Like all anti-schizophrenic drugs that have since been introduced, chlorpromazine was a dopamine (DA) antagonist. Since the introduction of chlorpromazine, anti-schizophrenic drugs have improved. Primarily they have become more specific in their effect, and, as a result, the side effects have decreased. One of the first widely used drugs introduced after Chlorpromazine is called Haloperidol (that goes by the commercial name, Haldol), and it is also a dopamine antagonist. However, it has it's primary effect on one principal type of dopamine receptor, DA2 receptors. Recently another class