Annualreport2014.dvi
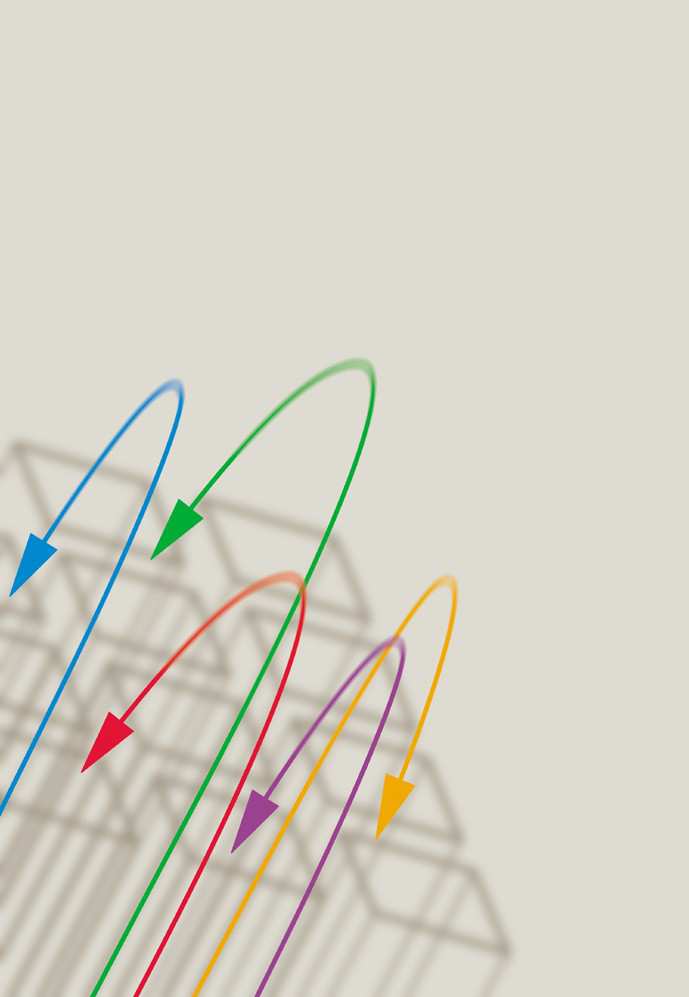
April 2013 – March 2014
IAP-Network StUDyS
Developing crucial Statistical methods for Understanding
major complex Dynamic Systems in natural, biomedical
and social sciences
ordinator: Ir ene Gijbels, KUL-1
Phase VII, Contract P7/06
1 General information and list of abbreviations
Main achievements per work package . . . . . . . . . . .
Most important network activities . . . . . . . . . . . .
3 Description of the research completed
Work package 1: The study of associations and dependencies in complexsystems . . . . . . . . . . . . . . . . . . .
Modeling of complex dependencies through copulas . . . . .
Flexible regression models . . . . . . . . . . . . 10
Qualitative constraints and goodness-of-fit testing . . . . . 12
Interactions with other work packages . . . . . . . . . 12
Work package 2: The study of different dynamics in complex systems
State space modeling, Bayesian shrinkage, and sparse and robustrepresentations . . . . . . . . . . . . . . . 13
Quantile-based spectral analysis . . . . . . . . . . 14
Further contributions to the study of dynamics in complex systems . 15
Interactions with other work packages . . . . . . . . . 16
Work package 3: Multivariate modeling and hierarchically structured data . 163.3.1
Hierarchical modeling of endpoints of different data types . . . 16
Joint modeling of several endpoints . . . . . . . . . . 17
Spatial correlated events . . . . . . . . . . . . . 18
Multivariate longitudinal profiles, allowing for incomplete data . . 18
Goodness-of-fit tests for hierarchical models . . . . . . . 18
Modeling of multivariate survival data . . . . . . . . . 18
Interactions with other work packages . . . . . . . . . 19
Work package 4: Dynamics of a stochastic system and the impact of non-observed characteristics . . . . . . . . . . . . . . . 203.4.1
Boundary estimation problems . . . . . . . . . . . 20
Deconvolution and inverse problems . . . . . . . . . 21
Homogeneity, heterogeneity and endogeneity . . . . . . . 22
Interactions with other work packages . . . . . . . . . 23
Work package 5: Variable and model selection and the study of (ultra-)highdimensional data . . . . . . . . . . . . . . . . . 243.5.1
Developing efficient and fast techniques for variable selection in ahigh dimensional setting . . . . . . . . . . . . . 24
Developing efficient variable selection methods in flexible regressionmodels, including robust selection procedures . . . . . . . 25
Developing methods for combining multiple sorts of informationfrom the same system . . . . . . . . . . . . . 26
Developing model selection methods for graphical models . . . 26
Constructing new model complexity measures . . . . . . . 26
Developing methods for statistical inference for high-dimensionalrandom matrices using LE Cam's approach . . . . . . . 27
Interactions with other packages . . . . . . . . . . 27
Meta Work package. The developed statistical methods in full use . . . 273.6.1
Understanding the dynamic aspects of affective disorders . . . 27
Predicting dynamic behavior of economics . . . . . . . . 28
Infectious disease epidemiology . . . . . . . . . . . 28
Quality and safety in food production . . . . . . . . . 29
Interactions with other work packages . . . . . . . . . 31
4 Network Activities
Organization of the network: administrative meeting . . . . . . . 33
Collaborations, working groups and seminars . . . . . . . . . 344.4.1
Training of young researchers: PhDs and postdocs . . . . . . . 384.6.1
Guidance of young researchers . . . . . . . . . . . 38
Training network for young researchers . . . . . . . . . 40
Prizes or special recognition obtained by network members . . . . . 41
List of publications per team . . . . . . . . . . . . . 445.1.1
Katholieke Universiteit Leuven, KUL-1 . . . . . . . . 44
Katholieke Universiteit Leuven, KUL-2 . . . . . . . . 56
Universit´e catholique de Louvain, UCL . . . . . . . . . 59
Universit´e libre de Bruxelles, ULB . . . . . . . . . . 83
Charles University at Prague, CU . . . . . . . . . . 87
Rijkskuniversiteit Groningen, RUG . . . . . . . . . . 88
5.1.10 Universidad de Santiago de Compostela, USC . . . . . . . 905.1.11 London School of Hygiene and Tropical Medicine, LSHTM . . . 92
List of co-publications . . . . . . . . . . . . . . . 935.2.1
Refereed publications (published). . . . . . . . . . 93
Refereed publications (in press, accepted for publication). . . . 97
Books & Book Chapters (in press). . . . . . . . . . 97
General information and list of abbreviations
The network consists of 7 Belgian partners and 4 international partners. We list in Table1 the names of the partners of the network, with their institution and research unit.
Katholieke Universiteit Leuven, Statistics
Katholieke Universiteit Leuven, Quantitative Psychology and Individual Differences
Universit´e catholique de Louvain,Institut de statistique, biostatistique et sciences actuarielles
Universiteit Gent, Biometrics and Statistics
Universiteit Hasselt, Center for Statistics
Universit´e libre de Bruxelles, ECARES and Department of Mathematics
Universit´e de Li ege, Statistique
Charles University at Prague, Statistics
Rijksuniversiteit Groningen, Social Science Statistics
Universidad de Santiago de Compostela, Statistics
London School of Hygiene and Tropical Medicine, Medical Statistics
Table 1: Belgian and international partners of the network.
The research project is built up around five work packages (WPs), and one meta work
package (MWP). Table 2 gives the main contributors to each work package and indicatesper package the partner that is coordinating the work. Obviously each partner is invitedto contribute to each work package, and actual contributions (from small to major) toeach work package come from all partners together.
Main contributing partners
WP1: The study of associations and dependencies
KUL-1, UCL, ULB, ULG∗, CU, USC
in complex systems
WP2: The study of different dynamics in complex systems
KUL-1, KUL-2, ULB∗, CU, RUG
WP3: Multivariate modeling
KUL-1, UCL, UG, UH∗, ULG, USC
and hierarchically structured data
WP4: Dynamics of a stochastic system and the impact
KUL-1, KUL-2, UCL∗, UG, RUG
of non-observed characteristics
WP5: Variable and model selection and the study
KUL-1, KUL-2, UG∗, UH, ULB, ULG
of (ultra-)high dimensional data
Meta WP: The developed statistical methods in full use
all partners (KUL-1∗)
Table 2: Main contributors per work package, and coordinating partner per work package(indicated with a ∗).
In Section 3 we report on the research results obtained, in the various work packages,during the report period. In this section we briefly summarize per work package the mainachievements. We also briefly comment on the most important network activities duringthe past year.
Main achievements per work package
Main achievements for WP1:
Copulas were further studied to investigate the association between variables. It in-cludes nonparametric and flexible estimation of conditional association, spatial and taildependence. Applications in finance and in environment studies with e.g. directionaldata were also proposed. Flexible regression models for potentially censored data weredeveloped. It includes among others the estimation of conditional quantiles, modelswith varying regression coefficients, survival models where an unknown proportion ofthe population is cured, functional analysis of variance, or the analysis of directionaldata. Goodness-of-fit tests on various aspects of these models and on the properties ofthe underlying data were also established.
Main achievements for WP2:
Progress has been made this year on all topics that are considered in this work package,with particularly significant advances on the themes that are also considered in Workpackage 5. For instance, there have been many contributions in the forecasting of high-dimensional data (through Bayesian shrinkage, sparse methods, or the development ofone-sided filters), with applications that are mainly in economics. Strong results havealso been obtained in functional data analysis (FDA), e.g., for data-driven selection oftuning parameters in functional linear regression. This is a crucial problem in FDAsince the number of principal components that are kept in the analysis (depending onthe sample size) has there a crucial impact on the performances of statistical procedures.
Main achievements for WP3:
Progress has been made to deal with correlations in complex hierarchical data, as wellas with the problem of integrating the marginal likelihood. Several contributions weredelivered to the study of spatial correlated events, in particular in a number of appli-cations. Advancements have been made to study the effect of misspecification of therandom-effects distribution in hierarchical models. Major steps in the modeling of sur-vival data were taken by the use of copulas to model dependencies for example betweensurvival and censoring times, or between data grouped in clusters. Furthermore, frailtymodels allowing for more flexibility (such as, for example, changes in time) have beenused in statistical analysis.
Main achievements for WP4:
In frontier estimation a major advancement has been in the study on conditional fron-tiers and efficiency scores, allowing to deal with unobserved heterogeneity.
theoretical results (e.g. minimax theory) have been established in inverse regressionproblems. Different modeling approaches for statistical inference in case of heterogene-ity have been investigated: semi-parametric transformation models, accelerated failuretime models, nonparametric instrumental regression models, modeling of latent vari-ables in mediation analysis, describing the various sources of variability in a mixedmodel setting, among others.
Main achievements for WP5:
New state–of–the–art variable and model selection procedures in a high dimensionaldata context are presented in this work package. Such procedures are highly relevantand much required in bioinformatics and biostatistics; the usefulness of the presentedmethods in these fields is demonstrated. Similarly, adequate and practical solutions tothe testing problems occuring in such high dimensional data have been put forward.
In developing such techniques, ample attention is given to the model assumptions. Onthe one hand, robust modeling and/or nonparametric solutions are provided. On theother hand, new measures are proposed to assess the goodness of fit of the selectedmodels/variables.
Main achievements for MWP:
In application areas, the methodologies developed in WPs 1—5 are used to provide an-swers to specific research questions. This led to two significant insights in the dynamicalaspects of affective disorders, as well as to newly gained insights in the regulation ofmaladaptive anger. Important advances have been made in the accuracy of economicforecasts, in the development of adequate statistical models for describing infectiousdisease epidemiology, and in an efficient study of food quality control. Furthermore,the developed methods have been put in full use in a number of other applicationsin nursing sciences, sport sciences, ecology and the statistical analysis of astronomicalobservations.
Most important network activities
The past year has been a special year, since it covers most of the year 2013, which was theInternational Year of Statistics. This special occasion acted as a catalyst on the researchactivities and events in the statistical community. Many meetings were organized bynetwork partners, within Belgium but also abroad. Several short courses and a wealthof statistical seminars were offered in the network. Moreover, two honorary doctorateswere delivered with promoters from the network. In addition, the interuniversity platform
for training of young researchers, financed via the Flemish government, got into an evenhigher acceleration with the development of new interuniversity training courses, and withsetting up an initiative for a future PhD researchers event jointly with colleagues from theFrench-speaking universities.
Description of the research completed
In the subsections below we describe the progress that has been made in the variouswork packages. The reporting in each work package is divided mostly according to themain objectives formulated in the original proposal. For convenience we therefore list (initalics) the major objectives exactly as formulated in the original proposal. Since researchis continuously evolving some slight shifts in emphasis may result. For each work package,we also indicate interactions with research results in other work packages. The referencesmentioned in the text can be found at the end of this report (for published or acceptedpapers, books or chapters in books) and on the web site for submitted papers (see theitem ‘Technical papers').
Work package 1: The study of associations and dependencies incomplex systems
Main goal: Measuring associations between characteristics (of scalar type, functionaltype, .) of a stochastic system can enter at various levels (in a time evolution, in tailsof distributions, .) This work package studies the statistical modeling of associationsand dependencies in complex stochastic systems, including testing for specific associationstructures.
Major objectives:
1.1. Modeling of complex dependencies through copulas.
(1) developing methods for modeling dependencies between real-valued variables and
(2) developing and study tests for testing for specific dependency (copula) struc-
(3) developing methods for constrained copula estimation, and doing inference based
(4) studying the use of conditional copula estimators in vine copula models to deal
with high-dimensional data;
(5) developing methods for conditional copula estimation for censored observations;
(6) developing nonparametric inference methods for extremal dependence within
random vectors, with emphasis on the high-dimensional case;
(7) developing dimension-reduction techniques for analyzing extremal dependence.
1.2. Flexible regression models.
The general aim is to study the associations between a vector of responses and a
vector of explanatory variables in regression by means of flexible regression models.
More precisely, we plan to :
(8) do inference in such flexible regression models, by using frequentist and Bayesian
(9) adapt regression methods that are developed for completely observed data, to the
censored data case;
(10) develop statistical methods for estimating quantiles in advanced flexible regres-
sion models.
1.3. Qualitative constraints and goodness-of-fit testing.
Here we study the associations or dependencies in a collection of variables. Thespecific objectives:
(11) developing tests for testing that two groups are mutually independent;
(12) testing that a pair of variables are independent conditionally upon the other
We next describe the obtained research results.
Modeling of complex dependencies through copulas
Conditional copulas
Researchers from KUL-1, UH and CU joined forces to develop bootstrap procedures forstatistical inference for conditional copula's, see Omelka et al. (2013). Veraverbeke etal. (2014) improve upon available nonparametric estimators for conditional distributionfunctions. The proposed procedure can also be used in other contexts, for example, whencensoring occurs. The link between conditional copula and conditional distribution func-tion then also allows to us this technique to estimate a conditional copula under theso-called simplifying assumption (such as in vine pair-copula constructions), see Gijbelset al. (2013).
Flexible estimation of conditional copula was also performed at ULG for conditionallyexchangeable random variables (Lambert, 2014). The starting point is a spline approxi-mation to the generator of an Archimedean copula. Changes in the dependence structurewith a covariate x are modelled by flexible regression of the spline coefficients on x.
Copulas: estimation and tests for specific dependence structures
The research work on testing for specific dependence structures and estimation under qual-itative constraints has been continued. It involves testing for tail monotonicity, for positivequadrant dependency and for stochastic monotonicity, among others, see e.g. Gijbels andSznajder (2013a,b).
Rayner et al. (2013) introduced an efficient algorithm for the construction of polynomialsthat are orthonormal on bivariate density functions: it can be used to build goodness-of-fittests for copulas.
For multivariate Gaussian copula models with unknown margins and structured correla-tion matrices, a rank-based, semiparametrically efficient estimator is proposed in Segerset al. (2013) for the Euclidean copula parameter.
Copulas and extremal dependences
In Einmahl et al. (2014), tail dependence models for distributions attracted to a max-stable law are fitted using observations above a high threshold. To cope with spatial,high-dimensional data, a rank-based M-estimator is proposed relying on bivariate marginsonly. A data-driven weight matrix is used to minimize the asymptotic variance. Ananalysis of wind speed data from the Netherlands illustrates the method.
Copulas and applications
A review on different approaches for statistical inference when modelling dependenciesthrough copulas was provided in Gijbels et al. (2014). In the PhD research work of KlausHerrmann (KUL-1) one of the aims is to investigate the distribution (and consequentlyquantiles and expectations) of a sum of a finite number of dependent components. Thedependence between the components is modelled through a copula, and applications infinance are looked at.
Copula-based measures of association between two random vectors are suggested in Grotheet al. (2013) The measures are applied to characterize strength and direction of associationof northern and southern European bond markets during the recent Euro crisis as well asassociation of stock markets with bond markets.
Statistical modelling of directional variables has also been addressed with contributions todensity estimation for directional–linear data. Garc´ıa–Portugu´es et al. (2013a) studies therelation between wind direction and SO2 concentration through circular–linear densities,with a copula approach. In addition, a kernel density estimator (Garc´ıa–Portugu´es et al.,2013b) and bandwidth selectors (Garc´ıa–Portugu´es, 2013) were introduced.
Copulas were also used by Braeken et al. (2013) in structural equation models to specifydependencies between error variables in personality questionnaires.
Flexible regression models
Inference in flexible regression models
Techniques of approximations with P-splines have been studied intensively in the networkin previous years. It is one of the basic ingredient in the study of grouped regularizationmethods for additive varying coefficient models in Antoniadis et al. (2014). Conditionalquantile estimation in varying coefficient models was tackled in Andriyana et al. (2014)and testing procedures for qualitative features of the varying coefficients were developedin Ahkim and Verhasselt (2014). The doctoral research of Yudhie Andriyana furtherfocuses on dealing with heteroscedasticity when estimating conditional quantiles. P-splines
were also used in the modeling of survival data gathered on subjects sampled from apopulation where an unknown proportion of them is not susceptible to the monitoredevent: Bremhorst and Lambert (2014), in a collaboration between UCL and ULG, extendthe promotion time model with covariates influencing simultaneously the probability ofbeing cured and the latent survival distribution. Identification issues are carefully studiedand discussed. P-spline models involve the selection of penalty parameters. Frasso andEilers (2014) developed an innovative procedure based on L-curves and V-curves. It iscomputationally efficient and robust, in particular when the data are serially correlated(with standard methods tending to over-smooth the data).
Wavelets and kernel methods were also used in various contexts. Autin et al. (2013, 2014)studied flexible estimation by wavelets in multidimensional settings. Pardo-Fern´andez etal. (2014) test for the equality of K regression curves in a fully nonparametric context: itis based on the comparison of empirical estimators of the characteristic functions of theregression residuals in the K sub-populations. Nonparametric tools were also developedto explore directional (environmental) data (Oliveira et al., 2013, 2014).
Adapting regression methods that are developed for completely observed data,to the censored data case
In Lopez et al. (2013), a nonparametric single-index regression model is considered in whichthe response is subject to right censoring with completely observed covariates. With po-tentially interval censored responses, Lambert (2013) proposed a nonparametric additivemodel for the location and the dispersion of a continuous variable with an arbitrary smoothconditional distribution.
Estimation of quantiles in advanced flexible regression models
Many univariate robust estimators are based on quantiles. The variance and hence themean squared error of some quantile-based estimators can be reduced by using smoothingtechniques, as was shown in Hubert et al. (2013).
Noh et al. (2013c) use the characterization of the joint distribution of variables by
their marginal distributions and the underlying copula to estimate conditional quantilessemi-parametrically. Mammen et al. (2013) compare nonparametric and parametric fitsin regression quantile models to test parametric specifications.
Just as there is a strong connection between quantiles and depth in location models
(Hallin et al., 2014), regression depth and regression quantiles are intimately related. Inthe regression context, Paindaveine and Van Bever (2013) introduce a local regressiondepth concept that can cope with multimodal distributions.
Qualitative constraints and goodness-of-fit testing
A novel approach to testing for homogeneity of dispersions, not relying on parametricassumptions and based on the means of within-group distances, was developed in a jointresearch by the KUL-1 and CU partners (Gijbels and Omelka, 2013).
Estimation of generalized additive models with functional covariates is tackled by Febrero–Bande and Gonz´
alez–Manteiga (2013). Garc´ıa–Portugu´es et al. (2013) introduce a testing
procedure for the functional linear model with scalar response. Gonz´alez–Manteiga andCrujeiras (2013) provide an overview on goodness–of–fit tests for regression models. Newproposals for testing regression methods are also provided by Boente et al. (2013), for ageneralized partially linear model, and by Ojeda et al. (2013), in the presence of selection–biased data. A test for assessing independence between a directional and a linear variablehas been proposed by Garc´ıa–Portugu´es et al. (2014). Goodness–of–Fit tests for directionaldensities (Boente et al., 2014) and a test for directional–linear independence (Garc´ıa–Portugu´es et al., 2013a) were also developed.
Interactions with other work packages
In all other work packages the study of relationships between variables (of different) natureis among the core tasks. The techniques developed in this work package therefore servedirectly as a key input for the other work packages, where often the focus is on differentaspects, such as complexity and high-dimensionality of the data (link to WP5), completeversus partial observational schemes (links to WP3 and WP4), the time dynamical aspectof the dependence structure (link to WP2), to name some crucial elements.
Work package 2: The study of different dynamics in complex sys-tems
Main goal: A stochastic system can exhibit different dynamics (time dynamics, spatialdynamics, .). This work package is concerned with efficiently modeling these differentlayers of dynamics, and with the development of statistical methodology for these complexdynamic systems.
Major objectives:
(1) developing methods for the joint modeling of many exchangeable stochastic systems
that show sizeable variation in their dynamics;
(2) developing methods for the joint modeling of many correlated stochastic systems;
(3) developing efficient statistical methods for a broad range of complex dynamic systems,
involving e.g. serially correlated functional data;
(4) developing sparse and robust estimators of models for high dimensional time series,
in particular for large dimensional vector autoregressive models.
We next describe the obtained research results.
State space modeling, Bayesian shrinkage, and sparse and robust rep-resentations
As last year, most emphasis in this part of the project was put on the analysis andprediction of high-dimensional time series, a field in which factor model methods so far havebeen the most efficient approach. A general form of dynamic factor models, containingall others as particular cases, was introduced in a paper in 2000 by Forni and co-authors,but their definitions were based on a spectral approach. Hallin and Lippi (2013) ratheradopted a time-domain approach, showing that the general dynamic factor model, contraryto other factor models, follows from a general representation result, and therefore doesnot really place restrictions on the high-dimensional process under study. A weak featureof general dynamic factor model methods, however, is that they classically involve two-sided filters resulting from the factorization of spectral density matrices: they are not wellsuited, therefore, in forecasting problems. Forni et al. (2014) improved on this by showinghow that two-sidedness issue can be handled by exploiting a specific property of reducedrank stochastic processes.
Still for the forecasting of high-dimensional times series, vector autoregressions (VARs)
are flexible and useful time series models. In high dimensions, however, their dense pa-rameterization leads to unstable inference and inaccurate forecasts. Informative priors canthen be used to shrink the richly parameterized VAR towards a parsimonious benchmark.
Giannone, Lenza and Primiceri (2014) studied the optimal choice of informativeness ofthese priors, which were treated as additional parameters. This reduces the importance ofsubjective priori choices and provides good out-of-sample forecasting performances. Fiecasand von Sachs (2013) adopted a similar approach and proposed shrinking a nonparamet-ric spectral estimator (smoothed periodogram) towards a diagonal shrinkage target whichconstitutes a compromise between a fully parametric (VAR) fit and a fully non-structuredregularising identity matrix. Still with a high-dimensional forecasting objective in mind,Conflitti et al. (2013) studied the problem of optimally combining individual forecasts ofgross domestic product (GDP) and inflation and proposed algorithms for computing theoptimal weights.
Beyond forecasting, another natural approach to analyze high-dimensional time series
is to introduce sparsity assumptions. Further progress was made there, in particular forcointegrated time series. Nonstationary time series are called cointegrated if a linear com-bination of them is stationary; sparse cointegration then means that only few coefficients inthat linear combination are non-zero. Cointegration theory relies heavily on canonical cor-relation analysis (CCA). Wilms and Croux (2013) developed a sparse approach to CCA,that forms the basis of sparse cointegration analysis. More broadly, a comprehensivereview and investigation of statistical process monitoring methods for high-dimensionaltime-series was presented in De Ketelaere et al. (2013) and Rato et al. (2014).
Finally, De Roover et al. (2014) contributed to the joint modeling of many exchange-
able stochastic systems by developing a novel switching principal component analysis
methodology to detect in multivariate time series data phases of consecutive time pointswith similar means and/or covariation structures. On the modeling of correlated stochas-tic systems, Verdonck and Tuerlinckx (2014) developed an analytically tractable multipleattractor network model of information accumulation for speeded two-choice decision mak-ing. This model accounts for a broad range of known psychophysical phenomena.
Functional data analysis
A crucial problem in most functional data analysis (FDA) procedures is the tuning of thedimension parameter. In functional linear models, for instance, one should determine howfast (compared to the sample size) can the dimension of the estimated linear operatorgrow to be consistent for the (infinite-dimensional) population operator. In this context,H¨
ormann and Kidzi´
nski (2014) proposed a data-driven dimension selection that avoids
the usual (unrealistic) assumptions. Moreover, minimax estimation of a linear functionalevaluated at the slope also relies on the optimal choice of a tuning parameter. Johannesand Schenk (2013) proposed a data-driven selection procedure of this tuning parameterand showed that the resulting fully data-driven estimator still achieves minimax optimalrates of convergence.
For functional time series data, most statistical procedures work in the time domain.
ormann et al. (2014) rather adopted the frequency domain approach to study opti-
mal dimension reduction for such data. This is achieved by so-called dynamic functionalprincipal components (DFPCs); these coincide with standard FPCs for i.i.d. functionaldata but are considerably more effective as a tool for dimension reduction when serialdependence is present. As a side product, a rigorous mathematical framework for spectraldensity operators along with an asymptotic theory for corresponding lag-window estima-tors were provided. Still in a setting of functional time series data, Aue, Dubart Norinhoand H¨
ormann (2014) introduced a new dimension and model selection criterion in or-
der to simultaneously tune the dimension and order of the model. This resulted into anautomatic forecasting of functional time series.
Other significant contributions to FDA were developed. In particular, Slaets et al.
(2013) constructed software for flexibly warping functional data in a Bayesian way. Claeskenset al.(2014) proposed a new depth function for multivariate functional data, that allowsto order such data, identify a central curve, and measure dispersion of the curves. Ajoint PhD research is currently supervised by the research partners at KUL-1 and CU forstudying probabilistic and statistical properties of depths, also in this FDA setup.
Quantile-based spectral analysis
Quantile- and copula-related spectral concepts recently have been considered. Dette etal. (2014) introduced a rank-based cross-periodogram involving Koenker and Bassett'scelebrated check function, and established its pointwise asymptotic properties. Kley et al.
(2014) provided an asymptotic analysis of a slightly different class of smoothed rank-based
cross-periodograms associated with the copula spectral density kernels from Dette et al.
(2014). They showed that, for a general class of (possibly non-linear) processes, properlyscaled and centered smoothed versions of those cross-periodograms, indexed by couples ofquantile levels, converge weakly to Gaussian processes. This leads to asymptotic confidenceintervals for copula spectral density kernels. It also provides asymptotic distributions(under serial dependence) for a new class of rank-based spectral methods involving theFourier transforms of rank-based serial statistics. Skowronek et al. (2014) provided a localstationary extension of Dette et al. (2014), which was applied to financial time series.
Copulas and ranks were also considered in a (multivariate) serial context in Kojadinovic
et al. (2013), for the problem of detecting changes in cross-sectional dependence structures.
They introduced a test based on a recently studied variant of the sequential empiricalcopula process. Ranks there are computed with respect to relevant subsamples, withbeneficial consequences for the sensitivity of the test.
Further contributions to the study of dynamics in complex systems
Extreme values (EVs) are often considered in time series. In this line of research, B¨
and Segers (2013) casted the classical EV method of annual maxima into an asymptoticframework involving a triangular array of block maxima. For absolutely regular stationarysequences, the empirical copula of the sample of vectors of block maxima was shown tobe a consistent and asymptotically normal estimator for the limiting EV copula. EVscan also be analyzed nonparametrically by estimating tail processes; Drees et al. (2014)adopted this approach for stationary, regularly varying Markov chains. Similarly, EVs ofa multivariate Markov chain with regulary varying stationary marginal distribution andasymptotically linear behavior were considered in Janssen and Segers (2014).
Modeling the dynamics in a dependence structure between time series is of particular
interest, and a semiparametric approach using local polynomial approximation was pre-sented in Gijbels, Herrmann and Sznajder (2014). The use of the proposed dynamicalmodelling approach is demonstrated in the analysis and forecast of wind speed data. For-est fire data was analyzed in Fuentes-Santos et al. (2013), by considering their spatialstructure with spatial point pattern analysis and inference techniques recently developedin the Spatstat package of R.
Finally, Jaeger and Lambert (2013) proposed a Bayesian ODE-penalized B-spline ap-
proach that enables to make inference in models specified by systems of linear ODEs whenthe error distribution is assumed Gaussian or adequately described by a mixture of Gaus-sians (Jaeger and Lambert, 2014). It is based on an approximation of the solution throughB-spline basis functions and on a penalty term related to the ODE that needs to be solved.
Similar ideas were used by Frasso et al. (2013) in frequentist and Bayesian settings to dealwith multi-dimensional dynamics described by PDEs. Efficient tools to make inference insystems ruled by nonlinear ODEs were also developed in Frasso et al. (2014).
Interactions with other work packages
All contributions above related to high-dimensional time series data (particularly thoserelying on shrinkage methods or sparsity assumptions) also naturally link to WP5. Sim-ilarly, the numerous papers above dealing with multivariate processes all model the de-pendence between the univariate processes involved, hence are naturally related to WP1where the development of general methods for investigating dependency structures (amongothers via copulas) is among the main objectives.
Work package 3: Multivariate modeling and hierarchically struc-tured data
Main goal: The aim of this work package is two-fold: analyzing hierarchically structureddata, and using hierarchical modeling with the aim to unravel the dynamics of the under-lying stochastic system. Survival data, for example, are often structured hierarchically,and exhibit complex association structures, possibly changing in time or space. Hierarchi-cal non-linear spatial and/or temporal modeling of species in the environment (describinge.g. the biological behavior of animals) is an important modeling tool.
Major objectives:
(1) developing statistical methods in hierarchical modeling for various endpoints of differ-
ent data types (e.g. observations grouped in clusters, hierarchically structured data);
(2) developing statistical methods for joint (dynamic) modeling of several endpoints;
(3) developing statistical methods for spatial correlated time events, taking into account
other spatial information;
(4) studying statistical methods for multivariate longitudinal profiles, allowing for incom-
plete data;
(5) developing goodness-of-fit tests for hierarchical models;
(6) studying the modeling of multivariate survival data via copula modeling, frailty mod-
eling and transformation modeling techniques, including a comparative study.
We next describe the obtained research results.
Hierarchical modeling of endpoints of different data types
In many applications, hierarchical modeling is used to study the complex underlying pro-cess of different endpoints. While hierarchical methods for normally distributed data arewell understood, there is a lot of ungoing research for other types of outcomes. Buildingon earlier work for non-Gaussian data, Generarlized Linear Mixed Model (GLMM) toolshave been built, extended, and refined for complex hierarchical data, exhibiting correla-tion and/or overdispersion (Efendi and Molenberghs, 2013; Efendi, Molenberghs and Iddi,2014; Ivanova et al., 2014). Van der Elst et al. (2013) focused in particular on the ap-plication of such methods in a psychometric context. An additional phenomenon that is
important with count data and requires further study is zero-inflation (Iddi and Molen-berghs, 2013; Kassahun et al., 2014). A difficulty with these models is the evaluation ofa marginal likelihood integrating out the random effects. As an alternative, these modelshave been looked at from a Bayesian perspective (Ghebretinsae et al., 2013; Aregay etal., 2013, 2014). Molas et al. (2013) considered H-likelihood inference where the explicitevaluation of the integral is avoided.
A problem in hierarchical data is the occurrence of negative correlation, because this
jeopardizes the typical hierarchical interpretation. Loeys and Molenberghs (2014) ad-dresses this in the context of psychology. Molenberghs et al. (2013) and Kenward andMolenberghs (2014) study various ways of formulating hierarchical models and their rela-tive advantages and disadvantages in terms of interpretable marginal functions and easy-to-use marginal parameters.
Item response theory (IRT) models can also be viewed as a particular set of GLMM
and Nonlinear Mixed Models (NLMM), but with some particular features. San Mart´ınet al. (in press) contributed to a better understanding of the (lack of) identifiability ofa particular IRT model, whereas Magis (in press) and Magis and De Boeck (in press)contributed to various estimation and testing issues. Ip et al. (2013) extensively studiedthe problem of fitting unidimensional item response models to data that have a strongdimension in addition to a few minor nuisance dimensions. From their part, Kadengye etal. (in press) developed a novel mixture IRT model that is especially suitable to study thetime dynamics of learning processes (along with individual differences therein).
Taverne and Lambert (2014) develop the inflated discrete beta regression model to
deal with discrete choices on Likert scales in surveys. The mean and the dispersion ofrates are jointly regressed on covariates using an underlying beta distribution with theacknowledgement that the profiles of some respondents invariably lead them to makeone specific choice. A novel approach to differential item functioning was considered byintroducing lasso penalization to the model fitting process and shrinking the item-groupinteraction parameters (Magis et al., 2014). The comparison of Bayesian and weightedlikelihood estimators of proficiency in polytomous item response theory models was furtherdiscussed (Magis, 2014b). Moreover, a formula for the asymptotic standard error of a broadclass of robust estimators of proficiency was derived (Magis, 2014a).
Joint modeling of several endpoints
Different contributions have been made in the topic of joint modeling of several endpoints.
Joint models for hierarchical data, possibly including time-to-event components, havebeen developed in Njeru Njagi et al. (2013, 2014) and Efendi et al. (2013). Molas etal. (2013) extended H-likelihood inference to multivariate GLMMs. Ceulemans et al.
(2013) proposed a robust version of multilevel simultaneous component analysis whichcan withstand the effects of outliers when analysing multivariate data with more than onelevel.
Spatial correlated events
Several applications with spatial correlated events where been investigated. (1) The spatio-temporal dynamics of Bluetongue virus were investigated by Faes et al. (2013). Ensoy etal. (2014) explored the effect of cattle movements on the spread in a dynamic model andinvestigate the spatio-temporal prediction of disease spread. (2) Dental caries experiencesare spatially references, since an active lesion on one surface may impact the decay processof the neighboring surfaces. Mutsvari et al. (2013) investigated the use of a spatiallyreferenced multilevel autologistic model, correcting for misclassification. (3) Breast cancerrisk is believed to be associated with several reproductive factors, such as early menarcheand late menopause. Duarte et al. (2014) used Structured Additive Regression models toexplore spatial and temporal correlations with a wide range of covariates. (4) Yehenew etal. (2013) studied the impact of hydro-electic dams on malaria incidence and demonstratethe equivalence between the frailty model and mixed Poisson regression models.
Multivariate longitudinal profiles, allowing for incomplete data
Incomplete data are inherent to longitudinal studies. Geva et al. (2013) gave an overviewof how missing data is generally treated in publications. New guidance on preventingand treating missing data are fostered by Mallinckrodt et al. (2013a,b). Novel strategiesfor handling missing data are given by Bartlett et al. (2014) for the situation of missingcovariate data, by Geerdens et al. (2013) for multivariate long-term follow-up data andby Donneau et al. (2013) for non-monotone missing ordinal data in longitudinal settings.
Clustering of multivariate longitudinal profiles, possibly subject to missing data, were
investigated. Bruckers et al. (2013, submitted) propose a cluster algorithm for multivari-ate longitudinal data. They also used techniques from functional data analysis, missingdata analysis and ensemble clustering to reveal groups of similar patients when facinghigh-dimensional multivariate data with missing observations (Bruckers et al. 2014a,b,submitted).
Goodness-of-fit tests for hierarchical models
Verbeke and Molenberghs (2013) presents model assessment tools for hierarchical datathat are modeled using random effects. A gradient-based method to study goodness-of-fitissues associated with correct and misspecification of the random-effects distribution inmixed models was developed (Verbeke and Molenberghs, 2014). Varewyck et al. (2014)investigate the impact of power on the use of random effect models as an alternative tofixed effect models, when the fixed effect models suffer from convergence problems.
Modeling of multivariate survival data
Clustered survival data are often analysed using frailty models. Cetiny¨
bert (2014) propose a semiparametric Bayesian shared frailty model to analyze correlated
interval-censored survival data. Flexible forms are considered jointly for the baselinehazard and the frailty distribution. Loeys et al. (2014) consider semi-parametric pro-portional hazards model with crossed random effects in the context of visual recognitiontasks. Munda et al. (2013) propose to relax the assumption that the frailty terms actsmultiplicatively on the hazard rate and consider frailty models with time-varying frailties.
Munda and Legrand (2013a,b) investigate the effect of misspecification of the frailty modeland propose a new diagnostic plot to guide the choice of the frailty distribution.
Also copula models are commonly used in this context, and several novel contributions
have been made. In Sujica and Van Keilegom (2013) a nonparametric location-scale modelis considered, in which the response is subject to random right censoring and censoringdepends on the response via a known conditional copula function. In Segers and Uytten-daele (2013) a rank-based method is developed to estimate the tree structure of a nestedArchimedean copula. Prenen et al. (2013) extended the Archimedean copula methodologyto model multivariate survival data grouped in clusters of variable size. And Rotolo etal. (2013) propose a simulation procedure based on a copula model allowing to introducedependence between times of different transitions and between those of grouped subjects.
Other flexible models for censored data have been developed as well. Heuchenne and
Laurent (2014a,b) and U˜
na-Alvarez et al. (2013) developed a method based on imputa-
tion techniques to estimate nonparametrically the conditional variance in a location-scaleregression model when the data are possibly right-censored and left truncated. Tala-makrouni et al. (2014) extended the method of parametrically guided non-parametricregression to the censored data case using an unbiased transformation of the data anda local linear fit. Yang et al. (2014) propose estimators of the coefficient functions forthe varying coefficient model in the case where different coefficient functions depend ondifferent covariates and the response is subject to random right censoring. Buyze andGoetghebeur (2013) studied properties and power of the cross-over design a non-recurrentright-censored survival time. Braekers and Grouwels (2013) proposed a semi-parametricCoxs regression model for zero-inflated left-censored time to event data.
Interactions with other work packages
Several of the mentioned research is linked with research in other work packages. As anexample, the research on multivariate survival data is linked with WP1, for example viathe concepts of frailties and copulas. Several papers also deal with the study of spatio-temporal dynamics of a process, and thus relate to research in WP2. The developedmethods have been investigated in different contexts, such as infectious diseases, in psy-chometrics, in bioinformatics, and in clinical trials. As such, there are clear interactionswith the MWP.
Work package 4: Dynamics of a stochastic system and the impactof non-observed characteristics
Main goal: For complex systems it is often impossible to have observations on all impor-tant variables. In micro-econometrics for example, the price of a good in a country mayalso depend on the welfare of the people living in that country, and the latter is difficult tomeasure. This work package studies how to deal with non-observed (latent) characteristicsin complex dynamic systems.
Major objectives:
4.1. Boundary estimation problems.
This problem arises for example in economics when estimating a production or a costfrontier function, which can be viewed as the boundary of the support of multivariaterandom variables. The main objectives:
(1) developing non- and semi-parametric methods for estimating boundaries or
frontiers, allowing for noisy data (robust estimators and stochastic frontier mod-els);
(2) developing non- and semi-parametric methods for estimating frontiers in the
presence of heterogeneous factors (observable and/or unobservable);
(3) developing non- and semi-parametric methods for estimating frontiers when
panel data are available;
4.2. Deconvolution and inverse problems.
In (ill-posed) inverse problems one often assumes that the transformation that linksthe observed signal to the signal of interest, is known. This assumption is howeverrather unrealistic. Our objectives:
(4) quantifying the influence of this noise on optimal convergence rates in a mini-
max sense;
(5) developing fully data-driven estimation procedures in this context;
(6) testing hypothesis regarding the unknown signal;
(7) developing Bayesian nonparametric estimation procedures.
4.3. Homogeneity, heterogeneity and endogeneity.
Identification and nonparametric estimation of the structural function in nonpara-metric instrumental regression with endogenous explanatory variables is a challeng-ing task. The major objectives:
(8) constructing fully data-driven estimation procedures;
(9) developing hypothesis tests and Bayesian nonparametric estimators, optimal in
a minimax sense.
We next describe the obtained research results.
Boundary estimation problems
In Cuevas et al. (2014) and Pateiro-L´
opez and Rodr´ıguez Casal (2013) the estimation of
sets and its application in image analysis are considered. The authors study in particular
shape reconstruction of a point cloud as well as the estimation of a medial axis and innerparallel body. Moreover, in order to handle data clouds in three dimensions, Lafarge etal. (2014) have implemented an R package.
The analysis of productivity of firms is intensively studied in the economics literature.
Simar and Wilson (2013) summarizes recent developments and perspectives for inferenceand estimation problems in nonparametric frontier models. A commonly used approachin this context is the estimation of productivity frontiers assuming typically outputs ob-served with some homogeneous measurement error. Simar, Vanhems and Van Keilegom(2013) extend the concepts of conditional frontiers and conditional efficiency scores to thecase of unobserved heterogeneity. The authors propose and analyze a model where theheterogeneity variable is linked to a particular input (or output). It is defined as thepart of the input (or the output), independent from some instrumental variable througha non separable nonparametric model. A particular attention is drawn to endogeneityissues involved in this model. In situations where firms face heterogeneous environmentalconditions the measurement of the impact of environmental factors given a nonparametricproduction model is studied in Bˇ
adin et al. (2013). In case their efficiency can be captured
by a conditional efficiency Florens et al. (2014) use a flexible nonparametric location-scalemodel to eliminate the dependence of inputs/outputs on these factors. These pre-whitenedinputs/outputs define the optimal frontier function and a pure measure of efficiency morereliable to produce rankings, since the influence of external factors has been eliminated.
Deconvolution and inverse problems
In Autin, Freyermuth and von Sachs (2014), in the context of denoising curves by tree-structured wavelet thresholding, the authors show the superiority of using block-thresholdingwithin the maxiset approach compared to classical unstructured wavelet threshold esti-mators. Considering a functional linear regression model, leading naturally to a linearinverse problem with noisy operator, Johannes and Schenk (2013) derive a minimax the-ory for the nonparametric estimation of a linear functional evaluated at the unknown slopefunction. A plug-in estimator which is based on a dimension reduction techniques and ad-ditional thresholding is proposed. Strong points of the newly derived theory consists inits applicability to a wide range of possible functional regressors, slope parameters andlinear functionals covering in particular point-wise estimation as well as the estimationof weighted averages of the parameter. Considering a Gaussian inverse regression modelthe influence of observational noise in the operator is studied in Johannes and Schwarz(2013) from a minimax-theory point of view. The authors develop a fully data-drivenestimation procedure combining model selection and Lepskis method which can attainminimax-optimal rates. An extension of the aforementioned results to inverse problemswith a nonlinear operator is a statistical demanding challenge. An iterative estimation ofthe solution of a nonlinear operator equation in the presence of noise in the operator isstudied in Dunker et al. (2014).
Considering a Gaussian inverse regression model with known linear operator Johannes
et al. (2014) link the frequentist minimax-theory to a nonparametric Bayesian approach.
The proposed nonparametric Bayesian estimation procedure allows to incorporate thefully data-driven choice of the smoothing parameter in a natural way. Moreover, it isshown that the rates of the concentration of the posterior distribution coincide with theminimax-rates established in Johannes and Schwarz (2013).
Homogeneity, heterogeneity and endogeneity
The identification in a semi-parametric transformation model, in which the regressionfunction has an additive nonparametric structure and the transformation of the responsebelongs to some parametric family, with an additional presence of endogeneity in theexplanatory variables is studied in Vanhems and Van Keilegom (2013). Moreover, theauthors propose a profile likelihood estimation method for the transformation and studyits theoretical properties.
Tiwari and Heuchenne (2013) estimated an accelerated failure time model for the time
between two transitions (for example, unemployment and employment). This model de-pends linearly on both exogenous and endogenous covariables and takes heterogeneityinto account. Further, Tiwari (2013) also considered a control function approach to es-timate a parametric single index model, where unlike other control function approaches,all regressors are correlated with the unobserved heterogeneity and a subset of them areendogenous.
Considering a nonparametric instrumental regression model Breunig and Johannes
(2013) develop a minimax theory for the nonparametric estimation of linear functionalof the structural function. A nonparametric estimation procedure is present which isminimax-optimal up to a constant over a wider range of classes for the structural function.
Furthermore, a fully data-driven estimation procedure is constructed which can attainminimax optimal rates of convergence. Assuming the independence of the instrument andthe regression error the nonparametric estimation of the structural function leads naturallyto a nonlinear operator equation. Iterative estimation procedures considering linear andnonlinear operator equations with noisy operator in nonparametric instrumental regressionare studied in Johannes et al. (2013) and Duncker et al. (2014), respectively.
Estimation procedures for panel data with large dimensions n, T , and general forms
of unobservable heterogeneous effects, are discussed in Bada and Liebl (2014). They givea description of their R-package phtt. The package also provides a wide range of dimen-sionality criteria in order to estimate the number of the unobserved factors simultaneouslywith the remaining model parameters.
Another source of endogeneity is the presence of censored or hierarchical data. Molen-
berghs and Lesaffre (2013), Mallinckrodt et al. (2014), Song et al. (2013), Grobler et al.
(2014), and Geva et al. (2014) contribute towards the dissemination of proper missing-data methodology. Some focus on clinical trials in general, others on specific areas, such
as geriatrics, etc. Donneau et al. (2014) investigates relative performance of missing-datamethodology for incomplete clinical trials.
Loeys et al. (2013) consider flexible imputation-based strategies for mediation analysis
based on natural direct and indirect effects. Loeys et al. (in press) and Moerkerke et al. (inpress) consider the handling of latent variables in mediation analysis and VanderWeele andVansteelandt (2014), and VanderWeele, Vansteelandt and Robins (2014) develop strategiesfor handling multiple mediators. Vansteelandt et al. (2014) and Vansteelandt and Daniel(2014) study the impact of model misspecification in covariate-adjusted effect estimatorsand develop estimators with improved robustness against model misspecification based onpropensity scores.
Understanding the various sources of variability in hierarchical settings often comes
down to testing hypotheses about variance components in mixed models. Standard testingprocedures do not apply due to hypotheses on the boundary of the parameter space.
Drikvandi et al. (2013) have proposed methods to do so without parametric assumptionsabout the unobserved latent variables in the models.
Data on tooth emergence in children are usually gathered in an epidemiological study
only at regular intervals. Therefore, the exact emergence time is not known but theinterval of emergence is. In order to understand the dynamics in tooth emergence, Cecereet al. (2013) proposed a new exploratory and graphical method which is an extensionof the principal component analysis biplots, but allowing for interval-censoring. Anotherexample where interval-censoring frequently occurs is in the modeling of of antimicrobialresistance data, for which Jaspers et al. (2014a,b) used semi-parametric mixture models.
In clinical trials, it is frequently of interest to estimate the time between the onset of
two events (e.g. duration of response in oncology). Dejardin and Lesaffre (2013) reviewexisting approaches and discuss their limitations in case that subjects are assessed at fixedvisits but the initial event and the terminating event occur in between visits and, henceare doubly interval censored. Furthermore, they propose a stochastic EM algorithm thatovercomes the problems in the existing approaches. They show by simulations the finitesample properties of their approach.
Interactions with other work packages
The use of shape constraints and flexible regression models for the estimation of sets,boundaries and frontiers leads to common interests with WP1. The study of regres-sion models with functional covariates is an other link with WP1. Dimension reductionmethods and smoothing parameter selection schemes are shared with WP5. Finally, thepresence of homogeneity, heterogeneity and endogeneity due to censored and hierarchicaldata is a link with WP3.
Work package 5: Variable and model selection and the study of(ultra-)high dimensional data
Main goal: The number of observed characteristics (variables) can be large to high toultra-high, when compared to the number of individuals (subjects) for which these vari-ables are measured. Methods that automatically can select the important characteristics(possibly of different nature) in complex systems need to be developed, also for situationsfor which the model adopted specifies that the number of characteristics grows very fast(even at a polynomial rate) with the number of subjects. Even for a set of selected char-acteristics, several models may be plausible, and model selection comes into play.
Major objectives:
(1) developing efficient and fast techniques for variable selection in a high dimensional
(2) developing efficient variable selection methods in flexible regression models, including
robust selection procedures;
(3) developing methods for combining multiple sorts of information from the same system:
defining objective functions, constructing algorithms, determining optimal weightsfor different data blocks;
(4) developing model selection methods for graphical models;
(5) constructing new model complexity measures; and developing model selection proce-
dures taking into account qualitative data patterns;
(6) developing method for statistical inference for high-dimensional random matrices using
Le Cam's approach.
We next describe the obtained research results.
Developing efficient and fast techniques for variable selection in a highdimensional setting
Variable selection problems occur often in a bioinformatics setting. Sikorska, Lesaffre,Groenen, and Eilers (2013) showed how in genome-wide association studies a huge numberof SNPs can be tested for their association with disease in a computationally efficient wayusing matrix operations in pure R code. In a similar setting, Sikorska, Rivadeneira et al.
(2013) proposed a conditional two-step approach to explore the longitudinal relationshipbetween the trait and the SNP. When some prior knowledge on a certain important setof variables is available, a natural assessment on the relative importance of the otherpredictors can be based on their conditional contributions to the response given the knownset of variables. Barut et al. (2013) proposed such a conditional screening technique ingeneralized linear models. A variable selection technique in the specific case of mixturecure models was developed by Dirick et al. (2013).
In a clinical setting, Varewyck et al. (2014) evaluated the quality of hospital care in
settings with a large number of hospitals and a large set of baseline patient characteristics
using the Firth corrected fixed effects regression models. It was shown how this frequentistbias correction on the scale of the estimating equations leads to stable convergence as wellas better power in realistic hospital settings.
In the context of personalized medicine, Doove et al. (in press) contributed an in-
depth review of a family of five recursive partitioning methods that have been recentlydeveloped for the selection of subgroups in randomized clinical trials. Dusseldorp and VanMechelen (2014) proposed a novel member of this family that focuses on so-called qualita-tive treatment-subgroup interactions (i.e., for some subgroups of persons one treatment isbetter than the other while for other subgroups the reverse is true), which are of particularrelevance for optimal treatment assignment.
Developing efficient variable selection methods in flexible regressionmodels, including robust selection procedures
Flexible regression models based on P-splines in the context of variable selection methodswere reviewed by Gijbels, Verhasselt and Vrinssen (2014). Generalized additive models(GAMs) were developed for discovering quantitative traits using bulk segregant analysiswith next generation sequencing (Claesen et al. 2013). The methods were applied andfurther refined for finding major and minor QTLs that contribute to thermo-tolerance inyeast (Yang et al. 2013). Instead of polynomial interpolation, Jansen (2013) and Jansen(2014a,b) developed a scheme for sparse multiscale representation of data on irregularpoint sets, using statistical smoothing.
An important research topic in this section is dealing with robust techniques. Croux
et al. (2013) and Oellerer et al. (2014) proposed and studied the robustness of the sparseleast trimmed squares estimator and other penalized robust regression methods by com-puting the influence function. The asymptotic biasedness of the estimators makes thecalculations non standard. Furthermore, Oellerer et al. (2013) proposed a robust andsparse regression estimator, called the shooting S, that can cope with componentwiseoutliers in the explanatory variables. Van Aelst (2014) developed a robust procedure toestimate the center of high dimensional data by reducing the effect of both contaminatedcells and structural outliers. Tharmaratnam and Claeskens (2013) studied variable selec-tion methods using robust M, S and MM estimators. Focused model selection for quantileregression was the topic of Behl et al. (2014), with an example on minimum effective dosedetermination. An overview of model selection methods was given in the encyclopediacontribution by Claeskens and Jansen (2014).
Another robust procedure was developed by Gijbels and Vrinssen (2013) for a multiple
regression model based on a specific adaptive lasso method. Sabbe et al. (2013) proposeda lasso logistic regression method in combination with an EM algorithm so as to allowfor a correct model selection in the presence of a missing data at random mechanism. Anapplication to a study on the development of dysphagia following radiotherapy for headand neck cancer was published by De Ruyck et al. (2013).
In a on nonparametric setting, De Neve et al. (2013b) adapted the Wilcoxon-Mann-
Whitney test for variable selection in a high dimensional context and they demonstratedthe method for RT-qPCR experiments. The method was also implemented as an R package(De Neve et al., 2014). Similar high dimensional hypotheses testing problem occur inepigenomics. Mensaert et al. (2014) gave an overview of the available data analysesmethods available today. Van Aelst and Willems (2013) developed routines and R softwareto estimate to perform robust inference and model selection based on the nonparametricbootstrap.
Developing methods for combining multiple sorts of information fromthe same system
Ballings' (2014) PhD dissertation focused on ensemble methods. Special attention goes tomodel fusion, an analogue variant of model selection. De Roover, Ceulemans et al. (2013)contributed a valuable clusterwise extension of the family of simultaneous component anal-ysis (SCA) methods (Ceulemans, Hubert and Rousseeuw, 2013), which implies a clusteringof the data blocks along with an SCA model per cluster. The same principle of cluster-wise extension was also successfully applied by Wilderjans and Ceulemans (2013) and DeRoover, Timmerman, Van Mechelen and Ceulemans (2013) to the PARAFAC model formultiway data. Somewhat relatedly, also a novel clusterwise SCA variant with commonand cluster-specific components was proposed by De Roover, Timmerman, Mesquita andCeulemans (2013). Van Deun et al. (2013) further made an in-depth theoretical andempirical comparison of no less than five different methods to identify common and/ordistinctive mechanisms underlying multiblock data.
Developing model selection methods for graphical models
Pircalabelu, Claeskens and Waldorp (2013) developed model selection methods for graph-ical models (see also Pircalabelu, Claeskens, Jahfari and Waldorp (2014) and Pircalabelu,Claeskens and Waldorp (2014)).
Constructing new model complexity measures
Information criteria for model selection for copula models were addressed by Geerdens etal. (2014) and a further overview of lack-of-fit tests and diagnostics for multilevel modelswas given in Claeskens (2013).
Multivariate relative dispersion measures (i.e. generalizations of the coefficient of vari-
ation to the multivariate setting) were investigated by Aerts et al. (2014a, 2014b) bymeans of influence functions.
Noh et al. (2013a, 2013b) developed methods based on the coefficient of determination
to quantile regression models to assess model misspecification.
Developing methods for statistical inference for high-dimensional ran-dom matrices using LE Cam's approach
Testing for sphericity is one of the central problems in inference for high-dimensional data.
While most contributions consist in providing testing procedures that are asymptoticallyvalid under high-dimensional asymptotics, the power and efficiency properties of such testsremain unexplored. Onatski et al. (2013, 2014) provided the first Le Cam approach tothis problem, by investigating local asymptotics under the so-called spiked alternatives, inthe n/p → c case.
Interactions with other packages
The work on blind deconvolution under positivity constraints, relates to WP4. All worklisted in Section 3.5.2 is also relevant for WP4. The study of high-dimensional covariancematrices as discussed in Section 3.5.5 is of immediate relevance for WP2, where the aspectis overall more on the time dynamics. Some of the research in this package is based onsurvival analysis, which has a natural link with WP3.
Meta Work package. The developed statistical methods in full use
Main goal: The developed statistical methods in full use. In this meta work package weaim, through continuous interactions with the other work packages, at answering specificquestions in focused application areas, in particular in econometrics, biomedical sciences,human and natural sciences. These questions on the one hand stand for the motivationof the research questions and on the other hand serve at demonstrating the impact of theplanned research on application areas.
Major objectives:
(1) Understanding the dynamic aspects of affective disorders;
(2) Predicting dynamic behavior of economics;
(3) Infectious disease epidemiology;
(4) Quality and safety in food production;
(5) Data integration in systems biology.
We next describe the obtained research results.
Understanding the dynamic aspects of affective disorders
The research contributed to two new and significant insights into the study of depression.
Firstly, making use of vector autoregressive models, Pe et al. (in press) showed that theemotion network of people suffering from Major Depressive Disorder is more dense (i.e.,displays a higher level of coherence over time). Secondly, making use of a dynamic modelbased on Lotka-Volterra equations, van de Leemput et al. (2014) revealed that mood
systems may have tipping points, with mood dynamics in the proximity of these tippingpoints becoming subject to a phenomenon called critical slowing down; this implies animportant early warning signal for the onset of depression.
A second noteworthy achievement pertains to the time dynamics of maladaptive anger
regulation: Using a tailor-made non-standard clustering methodology, Heylen et al. (inpress) were able to show how maladaptive regulation relates to both the amplitude andthe shape of anger intensity profiles across time.
Other significant results include new insights into the time-dynamic relation between
valence and arousal (as obtained by means of a flexible nonparametric regression method-ology by Kuppens et al., 2013) and the successful development by Bulteel et al. (2014) ofa tool for the study of intensive, within-person data on emotional responding. The lattertool, which is based on local robust PCA and outlier detection methods, allows the userto identify the timing and nature of latent changes in the mean level and covariation ofmultiple affective response channels.
Predicting dynamic behavior of economics
D'Agostino et al. (2013) assesses whether explicitly modeling structural change increasesthe accuracy of macroeconomic forecasts. It produces real time out-of-sample forecasts forinflation, the unemployment rate and the interest rate using a Time-Varying CoefficientsVAR with Stochastic Volatility (TV-VAR) for the US. The model generates accuratepredictions for the three variables. In particular for inflation the TV-VAR outperforms,in terms of mean square forecast error, all the competing models: fixed coefficients VARs,Time-Varying ARs and the naive random walk model. These results are also shown tohold over the most recent period in which it has been hard to forecast inflation.
Infectious disease epidemiology
Within the area of infectious disease epidemiology focus has been on: (1) The developmentand use of flexible regression models (link with WP1) to study prescriber determinants ofantibiotics (Blommaert et al., 2013), temporal patterns of influenza like illness (Bollaertset al.,2013; Vandendijck et al., 2013) and evolutions in co-payment for common medicationin Belgium (Fraeyman et al., 2013); (2) The development and use of (flexible) hierarchicalmodels (link with WP3) to study antibiotic use in Belgium (Minalu Ayele et al., 2013)and maternal mortality in Mozambique (Loquiha et al., 2013); (3) The development anduse of various statistical methods to tackle different research questions in infectious diseaseepidemiology (see e.g. Andraud et al., 2013; Castro-Sanchez et al., 2013; Potter and Hens,2013; Van Kerckhove et al., 2013).
Aregay et al. (2014) develop prediction methods in the setting of long-term vaccination
The use of state of the art and/or newly developed statistical techniques might lead
to new insights in the disease dynamics of malaria. More specifically, Yewhalaw et al.
(2013) investigated the effect of a hydro-electric dam in Ethiopia on malaria incidence. Itwas demonstrated that the dam itself does not impact on malaria incidence: during thedry season, transmission and mosquito abundance is low anyway, whereas in the rainyseason the mosquito abundance is so high that the dam does not have an impact as extrabreeding ground for the mosquitos. Furthermore, Yehenew et al. (2013) demonstratedthat different sampling schemes having equal power can be used. For the current study,more than 2000 children were followed up on a weekly basis, which could eventually leadto community fatigue. Alternatively, more children could be followed on a monthly basisinstead, leading to similar power under simple model conditions.
Quality and safety in food production
Rodr´ıguez et al. (in press) showed, by means of suitably applied multiway dimensionreduction procedures, how a fast and efficient food quality control could be achieved usingelectronic noses.
In food industry, the quality of meat produced for human consumption very much
depends on conditions under which animals were kept and/or transported. Studies in thiscontext are often highly hierarchical since measurements are taken at various levels (unitlevel, batch level, .), and this should be accounted for in the analysis. Permentier et al.
(2013) showed a relation between diet and carcass measures at slaughter of crossbreds.
Other applications
Applications in finance and managementHambuckers and Heuchenne (2014) model financial time series with heavy-tailed distri-butions: they compare sinh-arcsinh and generalized hyperbolic distributions computedon stock indices. Their model assumes a multiplicative heteroscedastic structure with anonparametrically estimated conditional variance.
In the study of control charts and their applications in management science, Faraz et
al. (2013) improved the power of variable ratio sampling control charts by using run ruleswhile Faraz et al. (2014) proposed a Shewhart-style control charting strategy (namedbundling) which describes how to create and average related time variables to allow fora reduction in the number of charts needed for monitoring delivery chain systems. Next,Celano et al. (2013) developed a control chart that monitors the social quality loss tofinal customers. The advantage of this chart is that processes are monitored according tospecification and upper control limits that are derived from the total price of a productin a market, which allows for a targeting cost philosophy. Seif et al. (2014a) evaluatedthe performance of the multiple variable sampling intervals scheme. Seif et al. (2014b)investigated the consequences of non-normality on adaptive sampling schemes enabling tomonitor processes for example based on heavy tails distributions.
Applications in social sciences and health sciencesVan der Elst et al. (2014) examines the use of various statistical methods towards theanalysis of repeated cognition data.
The study of antimicrobial resistance has become one of the main public health burdens
of the last decades, and monitoring the development and spread of non-wild-type isolateshas therefore gained increased interest. Monitoring is performed, based on the minimuminhibitory concentration (MIC) values, which are collected through the application of di-lution experiments. For a given antimicrobial, it is common practice to dichotomize theobtained MIC distribution according to a cut-off value, in order to distinguish betweensusceptible wild-type isolates and non-wild-type isolates exhibiting reduced susceptibilityto the substance. However, this approach hampers the ability to further study the char-acteristics of the non-wild type component of the distribution as information on the MICdistribution above the cut-off value is lost. As an alternative, new methods based on mix-ture models are presented, allowing the estimation of the full continuous MIC distribution,thereby taking all available information into account. In current research these modelsare extended to the multivariate setting of multi- and co-resistance, and to trend-modelsto examine possible time trends.
Minalu et al. (2013) use for change-points in mixed models to model antibiotic use.
To optimize the planning of blood donations but also to continue motivating the vol-
unteers it is important to streamline the practical organization of the timing of donations.
While donors are asked to return for donation after a suitable period, still a relevant pro-portion of blood donors is deferred from donation each year due to a too low hemoglobinlevel. Rejection of donation may demotivate the candidate donor and implies an inefficientplanning of the donation process. Hence, it is important to predict the future hemoglobinlevel to improve the planning of donors visits to the blood bank. Nasserinejad et al.
(2013) showed that transition models and mixed effects models can help in optimizing theplanning of blood donations. In general, the transition model provides a somewhat betterprediction than the mixed effects model, especially at high visit numbers. In addition, thetransition model offers a better trade-off between sensitivity and specificity when varyingthe cut-off values for eligibility in predicted values. Hence transition models make theprediction of hemoglobin level more precise and may lead to less deferral from donationin the future.
Lin et al. (2013) applies model selection methodology for hierarchical to the context
of genomic biomarkers, Demaerschalck et al. (2013) and Reynders et al. (2014) areapplications to public health in this respect. Tomsin et al. (2013, 2014) apply mixed-modelmethodology to longitudinal data collected in women with problematic pregnancies.
Complex data structures are also encountered in nursing science. Often, measure-
ments are taken at various units within a number of hospitals, and this hierarchical datastructure needs to be accounted for in the statistical analysis. Examples can be found inSchubert et al. (2013), Bruyneel et al. (2013), Van den Heede et al., 2013). A context
in which between-centre variability is important to study is the devolopment of qualityof care indicators to evaluate the performance of medical units. This requires assessingbetween- and within-unit variability, adjusting for important confounding factors. See forexample Penninckx et al. (2013).
Applications in ecology, earth and space sciencesIn modern Microbial Ecology high throughput technologies (high dimensional data) arefrequently used to infer the effects of controlled and uncontrolled environmental and ex-perimental conditions on the behavior of microbial communities, both in terms of theirabundance and functionality. Statistics and statisticians often play a crucial role in the de-sign and analysis stages of such studies. Within the scope of the StUDyS project, we haveemployed techniques which are beyond state-of-the-art methods in De Roy et al. (2013)and Ho et al. (2014). In particular, we have implemented complex optimal experimentaldesign methods and semiparametric quantile regression techniques.
Aerts et al. (2014) applies multiple-imputation methodology to study relationship
between astronomical observations between which complex relationships hold.
Applications in sport sciencesLiebl et al. (2014) propose testing procedures for differences in ankle palantarflexionstrengths of habitually rearfoot and forefoot runners. In order to approach this issue, theproblem of classifying different footfall patterns in human runners is revisited. A datasetof 119 subjects running shod and barefoot (speed 3:5 m/s) was analyzed. The footfallpatterns were clustered by a novel statistical approach, which is motivated by advances inthe statistical literature on functional data analysis.
Interactions with other work packages
Due to its special nature an role, this work package is linked to all others.
Network Activities
All activities of the IAP-statistics network can be followed closely from our web site. Theaddress of the web site is
The web site contains e.g.the following information:
• A brief history of the network referring to previous phases
• Composition of the network (including list of partners, members, personnel working
under the IAP project, visitors, .)
• List of members
• A brief description of the project (work packages and main objectives)
• Research activities (seminars, meetings, short courses, workshops, .)
• Brief overview of ongoing PhD and Postdoc projects within the network
• Lists of technical reports, of publications and of books written by members of the
• Contact details
New activities (seminars, short courses, meetings, .) are announced via various (ex-
isting) mailing lists. They are also announced at the IAP web site, where a link to theappropriate web page is added for more details.
Scientific meetings
Given the large number of research activities organized by IAP-network members, mainlyin the framework of the International Year of Statistics, the organization of the AnnualWorkshop will take place in the fall 2014 (instead of in spring 2014). The ULB partnerwill organize the next workshop, preliminarily announced for November 2014.
Many meetings were organized by members of the network in the period April 2013 –March 2014. The list below is restricted to meetings (co)-organized by IAP-members intheir own country.
• 4th Simulation models of infectious diseases (SIMID) Workshop, organised by UH, April
17 and 18, 2013.
• 2nd Dutch/Flemish Labmeeting Time Series and Dynamical Models, at the University
of Amsterdam. Co-organized by C. Albers (RUG). May 2013.
• New Developments in Econometrics and Time Series, Workshop, September 12–13,
2013, at ULB, Brussels (Belgium). Co-organized by D. Giannone, M. Hallin and S.
H¨
ormann (all ULB).
• The IAP-network organized a special lecture at the 21st Annual Meeting of the Belgian
Statistical Society, October 9–11, 2013, at the University of Gent (main organizer:S. Van Aelst, UG). Invited speaker for the network at the conference was A. Ko-marek (CU), with a talk on "Clustering for multivariate continuous and discretelongitudinal data".
• Statistics, your friend in daily life; whether you like it or not, UCL, October 25, 2013.
Main organizer (UCL, A. El Ghouch) and co-organizers from other partner univer-sities (P. Janssen (UH), G. Molenberghs (KUL-1/UH)). Meeting organized in theframework of the International year of Statistics 2013.
• Moving beyond questionable research practices: Symposium on good research practice in
behavioral sciences, an organization by IAP-members of KUL-2 (Francis Tuerlinckxand Wolf Vanpaemel), in a co-organization with University of Gent.
• International Hexa-Symposium on Biostatistics, Bioinformatics, and Epidemiology, or-
ganized by I-Biostat, at UH, November 14 and 15, 2013.
Awarding a Honorary Doctorate to Anastasios Tsiatis (Professor of Biostatistics,North Carolina State University, North Carolina, USA). Laudatio by G. Verbeke(KUL-1).
• Workshop on Applications of Modeling and Simulation in Drug Development, organized
by I-Biostat, at UH, December 12 and 13, 2013.
• International two-days workshop on the occasion of "25th Anniversary of LStat", with
several sessions organized by IAP-members (KUL-1 and KUL-2), December 13 and14, 2013.
Awarding a Honorary Doctorate to Nate Silver (ESPN, USA), Laudatio by I. Gijbels(KUL-1) and G. Molenberghs (KUL-1).
Some members of the network are also (co)-organizing workshops and conferences
abroad. Below some selection of such meetings (related to topics in the IAP-network),and participated by many members of the network.
• First Workshop on Model Selection, Nonparametrics and Dependence Modeling of the
Working Group on "Asymptotic Theory for Multidimensional Statistics" (see alsoSection 4.4.2), July 8–9 2013, Rennes, France. Co-organized by members of theKUL-1 (G. Claeskens and I. Gijbels) and UH (P. Janssen and A. Verhasselt) group.
• Mathematical Statistics and Limit Theorems, Conference in honor of Paul Deheuvels,
June 21–22, 2013, Paris (France). Involved in the organization: M. Hallin (ULB).
Organization of the network: administrative meeting
An administrative meeting will be organized in the fall 2014, at the occasion of the AnnualWorkshop.
Collaborations, working groups and seminars
The IAP network is working on a broad range of research topics in statistics. Thereis a large number of scientific collaborations within the network, as can be seen fromthe list of publications (see Section 5, and in particular Subsection 5.2, where all jointpublications are collected). Below, we mention a few examples of ongoing collaborationsbetween members of different teams of the network.
• Collaboration between KUL-1 (M. Giacofci, I. Gijbels, G. Claeskens) and ULB (M.
Jansen) on flexible wavelet estimation for functional data.
• A collaboration in multivariate survival analysis, more specifically in frailty models,
exists amongst P. Janssen (UH), C. Legrand (UCL) and L. Duchateau (UG).
• J.-M. Freyermuth (KUL-1) and M. Jansen (ULB) together work on applying multiscale
local polynomial decompositions to anisotropic multidimensional data.
ormann (ULB) and D. Hlubinka and M. Huˇskov´
a (both CU) are collaborating on
functional data problems, including also change point detection in a functional datasetup. These collaborations resulted in joint publications.
• Members of KUL-1 (among others I. Gijbels) are collaborating with D. Hlubinka (CU)
on research on data depth.
• M. Timmerman (RUG) is involved in an intensive research collaboration with several
people from KUL-2 (most notably E. Ceulemans), on multiblock component analysismodels, among others. This collaboration has, for the period 2013–2014, resulted inseveral peer-reviewed publications, including one in PloS ONE.
• Collaboration between several members of KUL-2 with H. Kiers (RUG) on models for
data fusion.
• Collaboration between several members of KUL-2 with M. Hubert (KUL-1) on robust
dimension reduction methods.
• Members of ULG and UCL collaborate on semi-competing risks (ULG: C. Heuchenne,
S. Laurent; UCL: C. Legrand and I. Van Keilegom), as well as on semiparametrictransformation models (ULG: C. Heuchenne; UCL: I. Van Keilegom, among others).
• Members of the USC and UCL groups have been collaborating in nonparametric esti-
mation and testing methods, and location-scale progressive three-state models (thelatter involving C. Cadarso-Su´
arez, from USC; and I. Van Keilegom, from UCL).
Specifically, there is an ongoing project on directional–linear regression, which is part
of the PhD thesis by E. Garc´ıa–Portugu´es, under the supervision of W. Gonz´alez–Manteiga and R.M. Crujeiras, and was developed during a research visit at UCL(from September–December 2013), where the student worked with I. Van Keilegom.
• There are extensive collaborations between (1) UH and KUL-1 on longitudinal data,
joint modeling of longitudinal and survival data, incomplete data methodology, sen-sitivity analysis for incomplete data and clinical trial methodology; (2) UH, KUL-1,and LSTHM on longitudinal and incomplete data and sensitivity analysis; (3) be-tween UH, KUL-1, and USC on longitudinal and incomplete data, sensitivity anal-ysis and clinical trial methodology. Further topics of collaboration include epidemicmodelling using social contact data and the role of (a)symptomatic infections (UHand LSTHM) and nonparametric estimation in flexible regression models and robustvariable selection techniques (UH and KUL-1).
• S. Nagy became a joint doctorate student of D. Hlubinka (co-supervisor, CU), I. Gi-
jbels (supervisor, KUL-1) and M. Hubert (co-supervisor, KUL-1) since October 1,2013. He has been awarded a PhD Scholarship from the Flemish Science Foundationenabling his long stay in Belgium.
• A number of people from the network, consisting of P. Janssen, Z. Shkeddy and R.
Braekers (UH) and L. Duchateau (UG) formed a group to coordinate a cross cut-ting initiative in Statistics sponsored by VLIR-UOS to deliver statistical courses indeveloping countries.
Below are a few examples of active working groups in the network. They are an importanttool to stimulate interactions between network partners, and to stay informed of theresearch achievements of other partners of the network.
• E. Ceulemans (KUL-2), and M. Timmerman and A. Stegeman (both RUG) are co-
chairs of the ERCIM Specialized team on multi-set and multi-way modeling, withM. Hubert (KUL-1), H. Kiers (RUG), and I. Van Mechelen (KUL-2) as members.
• F. Tuerlinckx and L. Bringmann (both KUL-2) and C. Albers and M. Timmerman
(both RUG) established a working group on time series in psychology, with a smallgroup meeting every six months.
• Members of the KUL-1, UCL and UH group are part of a scientific working group on
"Asymptotic Theory for Multidimensional Statistics". One of the aims of this groupis bringing together researchers working on asymptotic theory and mathematicalstatistics, and to stimulate collaborations in developing statistical theory.
• Members of the KUL-1 and UH group are part of a scientific working group on
"Longitudinal Data Analysis and Missing Data". One of the aims of this group is
bringing together researchers working on aspects related to longitudinal data anal-ysis, possibly subject to dropout, mixed models and sensitivity analysis.
• Members of the KUL-1 and UH groups are part of a scientific working group on
"Sensitivity, Surrogacy and Hierarchical Data Meeting". One of the aims of thisgroup is bringing together researchers working on correlated, multivariate, longitu-dinal data, and to stimulate collaborations in developing statistical methods, throughdiscussion sessions and informal talks. The meetings are also frequently visited bycolleagues from the Erasmus Universiteit Rotterdam, Maastricht University, and theInternational Drug Development Institute, among others.
• Members of UCL and ULG (C. Heuchenne and P. Lambert) are parts of a scientific
working group on "Semiparametric inference for survival and cure models".
• D. Magis (ULG) is member of the Research Group of Quantitative Psychology and
Individual Differences at KU Leuven, collaborating on research regarding DifferentialItem Functioning.
Each of the participating partners organizes on a regular basis statistics seminars at theiruniversities. Announcements of these seminars are sent out to most Belgian statisticians,including those participating in the network.
Apart from the regular statistics seminars at the universities involved, several seminars
have been organized by the network itself, e.g. around central themes of the network. Theyare on some occasions given by members of the network (at a different partner university),in order to foster research interactions and exchange of ideas. For brevity we only listthese seminars below.
• April 14, 2013: Christel Faes (UH), "Spatial disease mapping based on survey data: the
Health Interview Survey in Belgium", at UCL, Belgium.
• November 7, 2013: Marek Omelka (CU), "Testing for homogeneity of multivariate dis-
persions using dissimilarity measures", at the KU Leuven, Belgium.
• November 8, 2013: Davy Paindaveine (ULB), "On statistical depth and its local exten-
sions", at the UCL. Belgium.
• November 15, 2013: Daniel Hlubinka (CU), "Data Depth and Functional Data", at
ULB, Belgium.
• November 21, 2013: Daniel Hlubinka (CU), "Weighted halfspace data depths", at KUL-
• November 23, 2013: Eduardo Garc´ıa Portugu´es (USC), "Smoothing-based tests for
directional and linear data", at UCL, Belgium.
• November 28, 2013: Roel Braekers (UH), "Extending the Archimedean copula method-
ology to model multivariate survival data grouped in clusters of variable size", KUL-1, Belgium.
• February 14, 2014: Yvik Swan (ULG), "Entropy and the fourth moment phenomenon",
at UCL, Belgium.
• March 5, 2014: Marc Aerts (UH), "Constrained generalised profiling inference for
biomathematical models, with applications in microbial risk assessment", at CU,Czech Republic.
• March 7, 2014: Dominik Liebl (ULB), "Modelling Electricity Prices as Functional Data
on Random Domains", at UCL, Belgium.
• March 7, 2014: Laure Sansonnet (UCL): "A model of Poissonian interactions: detection
of dependence and nonparametric estimation", at ULB, Belgium.
For all other seminars on IAP-network topics organized during the reporting period,
we refer the reader to the web site.
Several short (intensive) courses have been organized. These courses are intended for allmembers of the network, and in particular (but not exclusively) for the PhD-studentsand the postdoctoral researchers. The announcements were posted on the web site, andsent out via existing mailing lists. No (or reduced) registration fees were required forIAP-members.
A list of the short courses organized during the period April 2013 – March 2014 is
given below.
• May 21–22, 2013. Short course on "Copula-based Dependence Models", given by E.F.
Acar (University of Manitoba, Canada), at UCL.
• August 3–4, 2013. Short course on "Foundations on recent advances in longitudinal and
incomplete data and in joint modeling". Organized at the Joint Statistical Meetings,Montreal, Canada. Lectures given by G. Molenberghs (UH), G. Verbeke (KUL-1),and D. Rizopoulos (Erasmus Medical Center, Rotterdam).
• September 17–21, 2013. ECAS'2013 course on "Functional and Complex Structure Data
Analysis". The ECAS 2013 session gave a general overview of the methodological andpractical aspects of Functional Data Analysis (FDA). The course took place at CastroUrdiales (Cantabria, Spain) with lectures by A. Cuevas (Universidad Aut´
Madrid, Spain), M. Febrero (USC), P. Hall (University of Melbourne, Australia) andV. Panaretos (Ecole Polytechnique F´ed´erale de Lausanne, Switzerland).
• September 23–27, 2013. Course in "Modelling Infectious Diseases and Health Economic
Evaluation of Vaccines". Lectures by N. Hens and N. Goeyvaerts (UH), and furtherP. Beutels (UA), J. Bilcke (UA), and M. Andraud (UA).
• November 5, 2013. Short course on "Dimension reduction in regression", given by F.
Portier (UCL), Belgium, at UCL.
• November 21, 2013. Short course on "Using Machine Learning and Bayesian Methods
to Analyze Large Supernovae Datasets", given by Dr. M. Varaghese (University ofCape Town, South Africa), at UCL.
• November 2013. Short course on "Causal inference in epidemiology: recent method-
ological developments", given by S. Van Steelandt (UG), at the on London Schoolof Hygiene and Tropical Medicine, London, UK.
• February 3–7, 2014. Short course on "Nonparametric Statistical Methods", by O. Thas
(UG), at the University of Wollongong, Australia.
Often short courses (in particular of advanced type), organized on a regular basis (for
example as part of a continuing education programme, or any other regular programme)are followed by PhD students from the network. We list a few examples of such courses:
• November 21-22 and 28-29, 2013: Short course "Optimization and numerical methods
in statistics: Concepts, models, and applications". The course took place at KULeuven and was given by F. Tuerlinckx (KUL-2), G. Molenberghs (KUL-1/UH), K.
van Deun (KUL-2), and T. Wilderjans (KUL-2).
• April 8–10, 2013: Short course on "Multilevel Analysis for Grouped and Longitudinal
Data", by L. Wijngaards-de Meij (University of Utrecht, NL), at UG.
Training of young researchers: PhDs and postdocs
Guidance of young researchers
Within the network there are many close collaborations. To foster collaborations and tostimulate exchange of knowledge, several PhD students in the network have a guidancecommittee that involves professors from at least two partners of the network (e.g. thepromoter and another member of a team from one of the other partners in the network).
Below are a few examples of IAP members that were/are part of the guidance com-
mittee or were/are a member of a PhD jury at other universities of the network. Thisparticipation is a very useful way to get familiar with the research carried out at othergroups of the network, and will be extended even more in the future. We give a selectionof such participations in training in the next tables. Information on the (co)-promoters ofthe PhD research at the university at which the student is affiliated, is not included. Thisand more information can be found on the web site.
IAP-members is the committee(s)
(+date defense)or ongoing
G. Claeskens (KUL-1):member guidance committee
Yudhie Andriyana,
A. Verhasselt (UH): member guidance committee
Mehreteab Fantahun
Z. Shkedy (UH): co-promoter
N. Hens (UH): member PhD jury
September 27, 2013
F. Tuerlinckx (KUL-2): member PhD jury
Isabelle Charlier,
C. Heuchenne: member guidance committee
doctoral examination committee
F. Tuerlinckx (KUL-2): member PhD jury
December 20, 2013
M. Hubert (KUL-1): member PhD jury
G. Molenberghs (UH): member PhD jury
G. Molenberghs (KUL-1/UH):
member guidance committee
R. Braekers (UH): co-promoter
September 27, 2013
Clement (UG): co-promoter
O. Thas (UG): member PhD jury
I. Van Mechelen (KUL-2): member PhD jury
M. Hubert (KUL-1): member PhD jury
doctoral examination committee
December 12, 2014
Yehenew Getachew,
P. Janssen (UH): co-promoter
G. Claeskens (KUL-1): guidance committee
M. Hubert (KUL-1): guidance committee
M. Aerts (UH): co-promoter
C. Faes (UH): member PhD jury
September 27, 2013
F. Tuerlinckx (KUL-2): member PhD jury
IAP-members is the committee(s)
(+date defense)or ongoing
G. Molenberghs (KUl-1/UH):
Trevor Kadengye, KUL-2
C. Faes (UH): member PhD jury
Gonzalize Montoro, USC
Edmund Njeri Nagi,
M. Kenward (LSHTM): member PhD jury
September 26, 2013
M. Kenward (LSHTM): member PhD jury
September 26, 2013
D. Hlubinka (CU): co-promoter
Majda Talamakrouni,
I. Gijbels (KUL-1): member guidance committee
Germain Van Bever,
M. Hubert (KUL-1): member PhD jury
September 6, 2013
Robin Van Oirbeek,
F. Tuerlinckx (KUL-2): member PhD jury
Marlies Vervloet,
G. Verbeke (KUL-1): member guidance committee
A complete list of PhD theses currently in preparation in the network can be found on
Training network for young researchers
Several members of the IAP Network (including Els Goetghebeur (UG), Marc Aerts (UH),Ir ene Gijbels (KUL-1)) have cofounded and are member of the steering committee of
flames which stands for "Flemish Training Network for Methodology and Statistics".
This is an inter-university training platform, that offers qualitative training in statisticsand methodology to young researchers (over all disciplines) dealing with statistical analysisin their research work. An inter-university team coordinates, develops and optimizes thestate-of-the-art training. The offered training makes use of the excellent competences andexpertises of all Flemish researchers in statistics and methodology. The KUL-1, KUL-2, UG, and UH partners of the IAP network are very actively involved in this traininginitiative. Within this platform these partners collaborate intensively in setting up newinter-university (IU) training courses and evensts/activities, in which at least three partneruniversities participate actively in the organization. The activities set up within the
framework of flames during the reporting period are listed below.
• May 23, 2013. Launching flames: Flanders Training Network for Methodology and
Statistics, at the Arsenal in Brussels. With presentations by Marleen Temmerman(World Health Organization), Pieternel Verhoeven (University College Roosevelt,The Netherlands) and John Crombez (State Secretary), among others.
• September 16–20, 2013. flames Summer School (FSS) 2013, organized at the KU
• October 9, 2013. Special Flames event (PhD day) at the Annual 2013 Meeting of the
Belgian Statistical Society, at Gent. Short course by Andrew Gelman on "StatisticalEducation Part I: Tricks for Teaching Statistics" and "Part II: Interesting StatisticsExample".
• March 26–28, 2014: flames IU Course on "Theory and Practice of Questionnaire Con-
struction and Analysis", at UH.
In addition to that there many local training courses offered. For more details we refer
the reader to the web site of this training platform:
Prizes or special recognition obtained by network members
Several members of the IAP network were internationally recognized for their researchwork. A selective list of such recognitions is provided below.
• Gerda Claeskens (KUL-1) was selected as Associate Editor of the Annals of Statistics
starting January 2013.
• Christine De Mol (ULB) was Chair of the SIAM Activity Group on Imaging Science
(January 2012–December 2013).
• Chella Ensoy (UH) received a best student poster award at GEOVET 2013, London.
• Domenico Giannone (ULB) was appointed as Fellow of the Centre for European
Policy Research (CEPR).
• Ir ene Gijbels (KUL-1) was selected as Editor-in-Chief of Journal of Nonparametric
Statistics, starting January 1, 2013.
• Marc Hallin (ULB) was appointed as Co-Editor-in-Chief of International Statistical
Review (2010–); and as Editor-in-Chief of Statistical Inference for Stochastic Pro-cesses (2013–).
• Marc Hallin (ULB) served as a member of the Institute of Mathematical Statistics
(IMS) Nominating Committee (August 2012–July 2013), and was selected as a chairof that committee (August 2013–July 2014).
• Niel Hens (UH) received a best poster award at the Epidemics Conference 2013,
• Philippe Lambert (ULg-UCL) is Associate Editor of Statistical Modelling: an Inter-
national Journal since 2007; and of Advances in Statistical Analysis since 2009.
• Philippe Lambert (ULg-UCL) is an elected member of the Executive Committee of
the Statistical Modelling Society since 2013.
• Christophe Ley's Ph.D. thesis (advisor: Davy Paindaveine (ULB)) won the Prix
Marie-Jeanne Laurent-Duhamel 2014 (this prize is awarded every three years by theFrench Statistical Society to the best Ph.D. in theoretical statistics defended in aFrench-speaking university)
• David Magis is "Statistical Software Reviews Editor" for the British Journal of
Mathematical and Statistical Psychology since 2007.
• Geert Molenberghs (KUL-1/UH) is co-editor of Biostatistics (2010–). He is a Series
Editor for the Wiley Series in Probability and Statistics. He is an Editor of StatRef,the OnLine statistical resource of Wiley.
• Mar´ıa Oliveira, a PhD student from USC, was awarded as the Best Young Researcher
in Biostatistics, at the Spanish Biometrics Conference held in May 2013 in Ciudad–Real, Spain.
• Davy Paindaveine (ULB) was selected as Co-Editor-in-Chief of Statistics & Proba-
bility Letters (2014–).
• Francis Tuerlinckx is member of the Board of Trustees of the Psychometric Society
• Iven Van Mechelen (KUL-2) was President of the International Federation of Clas-
sification Societies, 2012–2013.
• Wolf Vanpaemel (KUL-2) won the 2013 William K Estes Early Career Award of the
Society for Mathematical Psychology.
• Geert Verbeke (KUL-1) is elected member of council of the Royal Statistical Society,
• The NPCirc package for R (statistical software), developed by M. Oliveira, R.M.
Crujeiras and A. Rodr´ıguez–Casal, members of the USC group, received the John
Chambers 2014 Award from the American Statistical Association.
In this section we provide information on the scientific output of the IAP-statistics network.
We list the publications of network members in the period April 2013 – March 2014. Thepublication lists are restricted to (peer-reviewed) publications in international journals.
Refereed publications: We list all published papers in international journals (oredited books) in the period April 2013 – March 2014 (with refereeing system). Wemake the distinction between published papers and papers in press. See also theIAP-Statistics List of Published Papers on our web site:
Each published paper has a number of the form R13xxx or R14xxx. We do notmention these reference numbers below. For each published paper the web siteprovides a link towards the published paper (for example link to the journals page).
Books: These are books written by members of the network, that are published byinternational editors. They can also be found on the webpage
(reference numbers, provided on the web site, are of the form B13xxx and BP14xxx).
In the sections below we list the research output of the IAP-network for each of the
categories described above. We start with separate lists for each partner in the network,followed by a list of publications that are co-signed by researchers from at least two dif-ferent groups from the network.
Some summary statistics: the list includes
about 400 published papers,
about 120 papers to appear,
and about 25 books or chapters in books (published or to appear).
On the web site we also provide information regarding manuscripts written in the
period April 2013 – March 2014, and submitted for publication to an international journal.
These lists can be found at
Each Technical Report has a number of the form TR13xxx or TR14xxx.
List of publications per team
Katholieke Universiteit Leuven, KUL-1
Refereed publications (published).
Alfons, A., Croux, C. and Gelper, S. (2013). Sparse least trimmed squares regression
for analyzing high-dimensional large data sets. Annals of Applied Statistics, 7(1),226–248.
Alqerban, A., Hedesiu, M., Baciut, M., Nackaerts, O., Jacobs, R., Fieuws, S., Seden-
texCT Consortium, Willems, G. (2013). Pre-surgical treatment planning of maxil-lary canine impactions using panoramic vs cone beam CT imaging. DentomaxillofacRadiol., 42(9).
Altalie, S., Thevissen, P., Fieuws, S., Willems, G. (2014). Optimal dental age estimation
practice in United Arab Emirates' children. J Forensic Sci., 59(2), 383–385.
Andriyana, Y., Gijbels, I. and Verhasselt, A. (2014). P-splines quantile regression esti-
mation in varying coefficient models. Test, 23, 153–194.
Anthonissen, M., Daly, D., Fieuws, S., Massag´e, P., Van Brussel, M., Vranckx, J., Van
den Kerckhove, E. (2013). Measurement of elasticity and transepidermal water lossrate of burn scars with the Dermalab R
. Burns., 39(3), 420–428.
Apers, S., Moons, P., Goossens, E., Luyckx, K., Gewillig, M., Bogaerts, K., Budts, W.
(2013). Sense of coherence and perceived physical health explain the better quality oflife in adolescents with congenital heart disease. European Journal of CardiovascularNursing, 12(5), 475–483.
Aregay, M., Shkedy, Z., and Molenberghs, G. (2013). A hierarchical Bayesian approach
for the analysis of longitudinal count data with overdispersion: a simulation study.
Computational Statistics and Data Analysis, 57, 233–245.
Armstrong, P., Gershlick, A., Goldstein, P., Wilcox, R., Danays, T., Lambert, Y.,
Sulimov, V., Ortiz, F., Ostojic, M., Welsh, R., Carvalho, A., Nanas, J., Arntz, H.,Halvorsen, S., Huber, K., Grajek, S., Fresco, C., Bluhmki, E., Regelin, A., Vanden-berghe, K., Bogaerts, K., Van de Werf, F., the STREAM Investigative Team (2013).
Fibrinolysis or Primary PCI in ST-Segment Elevation Myocardial Infarction. TheNew England Journal of Medicine, 368(15), 1379–1387.
Autin, F., Claeskens, G. and Freyermuth, J.-M. (2014). Hyperbolic wavelet thresholding
methods and the curse of dimensionality through the maxiset approach. Applied andComputational Harmonic Analysis. 36(2), 239–255.
Barrette, K., Van Kelst, S., Wouters, J., Marasigan, V., Fieuws, S., Agostinis, P., van den
Oord, J., Garmyn, M. (2014). Epithelial-Mesenchymal Transition during invasionof cutaneous Squamous Cell Carcinoma is paralleled by AKT activation. BritishJournal of Dermatology, doi: 10.1111/bjd.12967.
Behl, P., Claeskens, G. and Dette, H. (2014). Focused model selection in quantile regres-
sion. Statistica Sinica, 24(2), 601–624.
Bertrand, V., Fieuws, S., Stalmans, I. and Zeyen, T. (2014). Rates of Visual Field Loss
Before and After Trabeculectomy. Acta Ophthalmol, 92(2), 116–120.
Bossuyt, X., Fieuws, S. (2014). Detection of anti-nuclear antibodies, added-value of solid
phase assay? Rheum Dis, 73(3), doi: 10.1136/annrheumdis-2013-204793.
Bouwland-Both, M.I., Steegers-Theunissen, R.P.M., Vujkovic, M., Lesaffre, E., Mook-
Kanamori, D.O., Hofman, A., Lindemans, J., Russcher, H., Jaddoe, V.W.V., Steegers,E.A.P. (2013). A periconceptional carbohydrate-rich dietary pattern is associatedwith first trimester growth: the Generation R study. BJOG: An International Jour-nal of Obstetrics & Gynaecology, 120, 435–445.
Brosens, V., Ghijselings, I., Lemiere, J., Fieuws, S., Clijmans, M. and Willems, G. (2014).
Changes in oral health-related quality of life reports in children during orthodontictreatment and the possible role of selfesteem: a follow-up study. European Journalof Orthodontics, 36, 186–191.
Brouckaert, O., Laenen, A., Smeets, A., Christiaens, M-R., Vergote, I., Wildiers, H.,
Moerman, P., Floris, G., Neven, P. (2014). Prognostic implications of lobular breastcancer histology: new insights from a single hospital cross-sectional study and SEERdata. Breast, doi:10.1016/j.breast.2014.01.007.
Brouckaert, O., Laenen, A., Wildiers, H., Floris, G., Moerman, P., Van Limbergen, E.,
Vergote, I., Billen, J., Christiaens, M.R., Neven, P. on behalf of MultidisciplinaryBreast Centre Leuven (2013). The prognostic role of preoperative and (early) post-operatively change in CA 15.3 serum levels in a single hospital cohort of primaryoperable breast cancers. Breast, 22(3), 254–262.
Bruyneel, L., Van den Heede, K., Aiken, L., Li, B., Lesaffre, E. and Sermeus, W. for the
RN4CAST consortium (2013). A multi-country perspective on nurses performingtasks below their skill level: reports by domestically trained nurses and nurses fromdeveloping countries. International Journal of Nursing Studies, 50(2), 202–209.
Bryan, S.R., Vermeer, K.A., Eilers, P.H.C., Lemij, H.G., Lesaffre, E. (2013). Robust
and censored modeling and prediction of progression in glaucomatous visual fields.
Invest Ophthalmol Vis Sci., 54(10), 6694–6700
Cecere, S., Groenen, P.J.F. and Lesaffre, E. (2013). The interval-censored biplot with an
application to the emergence sequence of permanent teeth. Journal of Computationaland Graphical Statistics, 22, 123–134.
Cerbone, L., Van Ginderdeuren, R., Van den Oord, J., Fieuws, S., Spileers, W., Van
Eenoo, L., Woniak, A., Sternberg, C.N., Schffski, P. (2014). Clinical presentation,pathological features and natural course of metastatic uveal melanoma, an orphanand commonly fatal disease. Oncology, 86, 185–189.
Ceulemans, E., Hubert, M. and Rousseeuw, P.J. (2013). Robust Multilevel Simultaneous
Component Analysis, Chemometrics and Intelligent Laboratory Systems, 129, 33–39.
Claeskens, G., Hubert, M, Slaets, L. and Vakili, K. (2014). Multivariate Functional
Halfspace Depth. Journal of the American Statistical Association, 109, 411–423.
Coolbrandt, A., Van den Heede, K., Clemens, K., Milisen, K., Laenen, A., Wildiers,
H., Verslype, C. (2013). The Leuven questionnaire for Patient Self-care duringChemotherapy (L-PaSC): Instrument development and psychometric evaluation.
European Journal of Oncology Nursing, 17(3), 275–283.
Coolbrandt, A., Van den Heede, K., Jans, E., Laenen, A. Verslype, C., Wildiers, H. and
Milisen, K. (2013). The Leuven Questionnaire on Patient Knowledge of Chemother-apy (L-PaKC): Instrument development and psychometric evaluation. EuropeanJournal of Oncology Nursing, 17(4), 465–473.
Coolbrandt, A., Wildiers, H., Aertgeerts, B., Van der Elst, E., Laenen, A., Dierckx de
Casterle, B., van Achterberg, T., Milisen, K. (2013). Characteristics and effective-ness of complex nursing interventions aimed at reducing symptom burden in adultpatients treated with chemotherapy: A systematic review of randomized controlledtrials. International Journal of Nursing Studies, 51, 495–510.
Croux, C., Filzmoser, P. and Fritz, H. (2013). Robust sparse principal component anal-
ysis. Technometrics, 55(2), 202–214.
Croux, C., Haesbroeck, G. and Ruwet, C. (2013). Robust estimation for ordinal regres-
sion. Journal of Statistical Planning and Inference, 143, 1486–1499.
Croux, C. and Reusens, P. (2013). Do stock prices contain predictive power for the future
economic activity? Journal of Macroeconomics, 35, 93–103.
Croux, C., Ronchetti, E., Salibian-Barrera, M. and Van Aelst, S. (2013). Special issue
on robust analysis of complex data of Computational Statistics & Data Analysis, 65,1–3.
De Brabanter, K., De Brabanter, J., Gijbels, I. and De Moor, B. (2013). Derivative
estimation with local polynomial fitting. Journal of Machine Learning Research,14, 281–301.
Declerck, D., Bottenberg, P., Carvalho, J., Declerck, K., De Vos, E., Vanden Abbeele,
A., Van Nieuwenhuysen, J., Vanobbergen, J., Bogaerts, K. (2013). Development andImplementation of an Oral Health Data Registration and Evaluation System for theBelgian Population. Journal of Dental, Oral and Craniofacial Epidemiology, 1(3),45–54.
Decramer, M., Janssens, W., Derom, E., Joos, G., Ninane, V., Deman, R., Van Renterghem,
D., Liistro, G., Bogaerts, K., Belgian Pulmonary Function Study Investigators (2013).
Contribution of four common pulmonary function tests to diagnosis of patients withrespiratory symptoms: a prospective cohort study. The Lancet Respiratory Medicine,1(9), 705–13.
Dejardin, D. and Lesaffre, E. (2013). Stochastic EM algorithm for doubly interval cen-
sored data. Biostatistics, 14(4), 766–778.
Demaerschalk, M., Vanden Boer, L., Bronselaer, J., Molenberghs, G. and Declerq, A.
(2013). The influence of Municipal Characteristics on the use of informal Home care& home care services by the Flemish Elderly. European Journal of Public Health,23, 241–246.
De Neubourg, D., Bogaerts, K., D'Hooghe, T. (2014). Reply: Legislation of embryo
transfer affected the denominator, not the numerator! Human Reproduction, 29(2),382.
De Neubourg, D., Bogaerts, K., Wyns, C., Albert, A., Camus, M., Candeur, M., Deguel-
dre, M., Delbaere, A., Delvigne, A., De Sutter, P., Dhont, M., Dubois, M., En-glert, Y., Gillain, N., Gordts, S., Lesaffre, E., Lejeune, B., Hautecoeur, W., Leroy,F., Ombelet, W., Perrier, S., Hauterive, D., Vandekerckhove, F., Van der Elst, J.,Hooghe, T. (2013). The history of Belgian assisted reproduction technology cycleregistration and control: a case study in reducing the incidence ofmultiple pregnancy.
Human Reproduction, 28(10), 2709–2719.
Denys, D., Shahbazian, M., Jacobs, R., Laenen, A., Wyatt, J., Vinckier, F., and Willems,
G. (2013). Importance of root development in autotransplantations: a retrospectivestudy of 137 teeth with a follow-up period varying from 1 week to 14 years. TheEuropean Journal of Orthodontics, 35(5), 680–688.
Dierickx, D., Tousseyn, T., Morscio, J., Fieuws, S., Verhoef, G. (2013). Validation of
Prognostic Scores in Post-Transplantation Lymphoproliferative Disorders. J ClinOncol., 31(27), 3443–3444.
Dierickx, D., Tousseyn, T., Sagaert, X., Fieuws, S., Wodarska, I., Morscio, J., Bre-
poels, L., Kuypers, D., Vanhaecke, J., Nevens, F., Verleden, G., Van Damme-Lombaerts, R., Renard, M., Pirenne, J., De Wolf-Peeters, C., Verhoef, G. (2013).
Single-center analysis of biopsy-confirmed posttransplant lymphoproliferative dis-order: incidence, clinico-pathological characteristics and prognostic factors. LeukLymphoma., 54(11), 2433–2440.
Drikvandi, R., Verbeke, G., Khodadadi, A. and Partovi Nia V. (2013). Testing multiple
variance components in linear mixed effects models. Biostatistics, 14, 144–159.
Forcheh, A.C., Verbeke, G., Kasim, A., Lin, D., Shkedy, Z., Talloen, W., Ghlmann,
H.W.H. and Clement, L. (2013). BeadarrayFilter: An R Package to Filter Beads.
The R Journal, 5, 171–180.
Franco, A., Thevissen, P., Fieuws, S., Souza, P.H., Willems, G. (2013). Applicability of
Willems model for dental age estimations in Brazilian children. Forensic Sci Int.,231(1-3).
Geerdens, C., Claeskens, G. and Janssen, P. (2013). Goodness-of-fit tests for the frailty
distribution in proportional hazards models with shared frailty. Biostatistics, 14(3),433–444.
Geraerts, I., Van Poppel, H., Devoogdt, N., Laenen, A., De Groef, A. and Van Kam-
pen, M. (2014). Progression and predictors of physical activity levels after radicalprostatectomy. BJU International, doi: 10.1111/bju.12465
Ghebretinsae, A.H., Faes, C., Molenberghs, G., De Boeck, M., and Geys, H. (2013). A
Bayesian generalized frailty model for comet assays. Journal of BiopharmaceuticalStatistics, 11, 449–455.
Ghijselings, I., Brosens, V., Willems, G., Fieuws, S., Clijmans, M. and Lemiere, J. (2014).
Normative and self-perceived orthodontic treatment need in11- to 16-year-old chil-dren. European Journal of Orthodontics, 36, 179–185.
Gijbels, I., Herrmann, K. and Verhasselt, A. (2013). Discussion to the paper "Large
covariance estimation by thresholding principal orthogonal components", by Fan,J., Liao, Y. and Mincheva, M. Journal of the Royal Statistical Society, Series B, 75,603–680. Discussion on pages 662–663.
Gijbels, I. and Omelka, M. (2013). Testing for homogeneity of multivariate dispersions
using dissimilarity measures. Biometrics, 69, 137–145.
Gijbels, I. and Sznajder, D. (2013a). Testing tail monotonicity by constrained copula
estimation. Insurance: Mathematics and Economics, 52, 338–351.
Gijbels, I. and Sznajder, D. (2013b). Positive quadrant dependence testing and con-
strained copula estimation. The Canadian Journal of Statistics, 41 (1), 36–64.
Goossens, G.A., J´erˆ
ome, M., Janssens, C., Peetermans, W.E., Fieuws, S., Moons, P.,
Verschakelen, J., Peerlinck, K., Jacquemin, M. and Stas, M. (2013). Comparingnormal saline versus diluted heparin to lock non-valved totally implantable venousaccess devices in cancer patients: a randomised, non-inferiority, open trial. Annalsof oncology, 24(7), 1892–1899.
Grobler, A., Matthews, G. and Molenberghs, G. (2014). The impact of missing data on
clinical trials: a re–analysis of a placebo controllied trial of hypericum perforatum(St John's Wort) and sertraline in major depressive disorder. Psychopharmacology,231(9), 1987–1999.
Hompes, D., D'Hoore, A., Wolthuis, A., Fieuws, S., Mirck, B., Bruin, S. and Verwaal, V.
(2014). The use of Oxaliplatin or Mitomycin C in HIPEC treatment for peritonealcarcinomatosis from colorectal cancer: A comparative study. J. Surg. Oncol., 109,527–532.
Hompes, T., Izzi, B., Gellens, E., Morreels, M., Fieuws, S., Pexsters, A., Schops, G.L.,
Dom, M., Van Bree, R., Freson, K., Verhaeghe, J., Spitz, B., Demyttenaere, K.,Glover, V., Van den Bergh, B., Allegaert, K., Claes, S. (2013). Investigating theinfluence of maternal cortisol and emotional state during pregnancy on the DNAmethylation status of the glucocorticoid receptor gene (NR3C1) promotor region incord blood. Journal of Psychiatric Research, 47(7), 880–891.
Hubert, M., Gijbels, I. and Vanpaemel, D. (2013). Reducing the Mean Squared Error
of quantile-based estimators by smoothing. Test, 22, 448–465. Special issue ICORS2011.
Iddi, S. and Molenberghs, G. (2013). A marginalized model for zero-inflated, overdis-
persed and correlated count data. Electronic Journal of Applied Statistical Analysis,6, 149–165.
Ip, E., Molenberghs, G., Che, S.H., A., Goegebeur, Y., and De Boeck, P. (2013). Func-
tionally unidimensional item response models. Multivariate Behavioral Research,48, 534–562.
Jaspers, S., Aerts, M., Verbeke, G., and Beloeil, P.A. (2014). A new semi-parametric
mixture model for interval censored data, with applications in the field of antimicro-bial resistance. Computational Statistics and Data Analysis, 71, 30–42.
Jaspers, S., Aerts, M., Verbeke, G., and Beloeil, P.A. (2014). Estimation of the wild-type
MIC value distribution. Statistics in Medicine, 33, 289–303.
Joniau, S., Briganti, A., Gontero, P., Gandaglia, G., Tosco, L., Fieuws, S., Tombal, B.,
Marchioro, G., Walz, J., Kneitz, B., Bader, P., Frohneberg, D., Tizzani, A., Graefen,M., van Cangh, P., Karnes, R.J., Montorsi, F., Van Poppel, H., Spahn, M. (2014).
European Multicenter Prostate Cancer Clinical and Translational Research Group(EMPaCT) Stratification of High-risk Prostate Cancer into Prognostic Categories: AEuropean Multi-institutional Study. Eur Urol, http://dx.doi.org/10.1016/j.eururo.2014.01.020.
Joniau, S., Van den Bergh, L., Lerut, E., Deroose, C., Haustermans, K., Oyen, R.,
Budiharto, T., Ameye, F., Bogaerts, K., Van Poppel, H. (2013). Mapping of PelvicLymph Node Metastases in Prostate Cancer. European Urology, 63(3), 450–458.
Klingels, K., Feys, H., Molenaers, G., Verbeke, G., Van Daele, S., Hoskens, J., Desloovere,
K., and De Cock, P. (2013). Randomized trial of modified constraint-induced move-ment therapy with and without an intensive therapy programme in children withunilateral cerebral palsy. Neurorehabilitation and Neural Repair, 27(9) 799–807.
Leroy, R., Bogaerts, K., Hoppenbrouwers, K., Martens, L., Declerck, D. (2013). Den-
tal attendance in preschool children - a prospective study. International journal ofpaediatric dentistry / the British Paedodontic Society [and] the International Asso-ciation of Dentistry for Children, 23(2), 84–93.
Li, B., Bruyneel, L., Sermeus, W., Van den Heede, K. Matawie, K., Aiken, L. and
Lesaffre, E. (2013). Group-level impact of work environment dimensions on burnoutexperiences among nurses: a multivariate multilevel probit model. InternationalJournal of Nursing Studies, 50(2), 281–291.
Lin, D., Tilahun, A., Corti˜
nas, J., Shkedy, Z., Molenberghs, G., Goelhmann, H.W.H.,
Talloen, W. and Bijnens, L. (2013). Comparison of methods for the selection ofgenomic biomarkers. International Journal of Data Mining and Bioinformatics, 8,24–41.
Lintermans, A., Van Asten, K., Wildiers, H., Laenen, A., Paridaens, R., Weltens, C.,
Verhaeghe, J., Vanderschueren, D., Smeets, A., Van Limbergen, E., Leunen, K.,Christiaens, M.R., Neven, P. (2014). A prospective assessment of musculoskeletaltoxicity and loss of grip strength in breast cancer patients receiving adjuvant aro-matase inhibitors and tamoxifen, and relation with BMI Breast. Breast CancerResearch and Treatment, 146(1), 109–116.
Maleux, G., Deroose, C., Fieuws, S., Van Cutsem, E., Heye, S., Bosmans, H., Verslype,
C. (2013). Prospective comparison of hydrogel-coated microcoils versus fibered plat-inum microcoils in the prophylactic embolization of the gastroduodenal artery beforeyttrium-90 radioembolization. J Vasc Interv Radiol., 24(6), 797–803.
Maleux, G., Gillardin, P., Fieuws, S., Heye, S., Vaninbroukx, J., Nackaerts, K. (2013).
Large-bore nitinol stents for malignant superior vena cava syndrome: factors influ-encing outcome. AJR Am J Roentgenol., 201(3), 667-674.
Menten, J., Boelaert, M. and Lesaffre, E. (2013). Bayesian meta-analysis of diagnostic
tests allowing for imperfect reference standards. Statist. Med., 32, 5398–5413.
Meuleman, C., Tomassetti, C., Wolthuis, A., Van Cleynenbreugel, B., Laenen, A., Pen-
ninckx, F., Vergote, I., D'Hoore, A, D'Hooghe, T. (2014). Clinical outcome after rad-ical excision of moderate-severe endometriosis with or without bowel resection andreanastomosis: A prospective cohort study. Annals of Surgical Oncology, 259(3),522–531.
Minalu, G., Aerts, M., Coenen, S., Versporten, A., Muller, A., Adriaenssens, N., Beutels,
P., Molenberghs, G., Goossens, H., and Hens, N. (2013). Adaptive change-pointmixed models applied to data on outpatient antibiotic use in Europe. StatisticalModeling, 13, 253–274.
Molas, M., Noh, M., Lee, Y., and Lesaffre, E. (2013). Joint hierarchical generalized
linear models with multivariate Gaussian random effects. Computational Statisticsand Data Analysis, 68, 239–250.
Molenberghs, G., Alonso Abad, A., Van der Elst, W., Burzykowski, T., and Buyse, M.
(2013). Review: Clinical Trial Methodology: Surrogate endpoints: when shouldthey be used? Clinical Investigation, 3, 1147–1155.
Molenberghs, G. and Lesaffre, E. (2013). Missing Values. In: Wiley Encyclopedia of
Clinical Trials (2nd ed.) (D'Agostino, Sullivan, and Massaro, eds.) New York: JohnWiley & Sons, Inc.
Molenberghs, G., Kenward, M.G., Verbeke, G., Efendi, A., and Iddi, S. (2013). On
the connections between bridge distributions, marginalized multilevel models, andgeneralized linear mixed models. International Journal of Statistics and Probability,2, 1–21.
Mutsvari, T., Bandyopadhyay, D., Declerck, D. and Lesaffre, E. (2013). A multilevel
model for spatially correlated binary data in the presence of misclassification: anapplication in oral health research. Statist. Med., 32, 5241–5259.
Mutsvari, T., Declerck, D. and Lesaffre, E. (2013). Correction for misclassification of
caries experience in the absence of internal validation data. Clinical Oral Investiga-tions, 17(8), 1799–1805.
Nasserinejad, K., de Kort, W., Baart, M., Komarek, A., van Rosmalen, J., Lesaffre,
Using dynamic regression and random effects models for predicting
hemoglobin levels in novel blood donors. BMC Medical Research Methodology, 2,13–62.
Njeru Njagi, E., Rizopoulos, D., Molenberghs, G., Dendale, P., and Willekens, K. (2013).
A joint survival-longitudinal modelling approach for the dynamic prediction of re-hospitalization in telemonitored chronic heart failure patients. Statistical Modeling,13, 179–198.
Omelka, M., Veraverbeke, N. and Gijbels, I. (2013). Bootstrapping the conditional cop-
ula. Journal of Statistical Planning and Inference, 143, 1–23.
Peeraer, K., Debrock, S., Laenen, A., De Loecker, P., Spiessens, C., De Neubourg, D.,
D'Hooghe, T. (2014). The impact of legally restricted embryo transfer and reim-bursement policy on cumulative delivery rate after treatment with assisted repro-ductive technology. Human Reproduction, 29(2), 267–275.
Penninckx, F., Kartheuser, A., Van de Stadt, J., Pattyn, P., Mansvelt, B., Bertrand,
C., Van Eycken, E., Jegou, D., Fieuws, S., PROCARE. (2013). Outcome follow-ing laparoscopic and open total mesorectal excision for rectal cancer. Br J Surg.,100(10), 1368–1375.
Permentier, L., Maenhout, D., Broekman, K., Deley, W., Verbeke, G., and Geers, R.
(2013). Effect of different dietary total Lys: Energy ratios and dietary energy con-centrations in phase feeding from weaning to slaughter on performance and carcassmeasures of crossbred pigs selected for leanness. Livestock Science, 155, 332–342.
Reynders, A., Brouckaert, O., Laenen, A., Smeets, A., Leunen, K., Amant, F., Persyn,
F., Yoshihara, E., Soens, J., Moerman, Ph., Vergote, I., Christiaens, M.R., Neven, P.
(2014). Prediction of non-sentinel lymph node involvement in breast cancer patientswith a positive sentinel lymph node. Breast, doi: 10.1016/j.breast.2014.03.009.
Rosselle, L., Permentier, L., Verbeke, G., Driessen, B., and Geers, R. (2013). Interactions
between climatological variables and sheltering behavior of pastoral beef cattle dur-ing sunny weather in a temperate climate. Journal of Animal Science, 91, 943–949.
Schubert, M., Ausserhofer, D., Desmedt, M., Schwendimann, R., Lesaffre, E., Li, B., De
Geest, S. (2013). Frequency and correlates of implicit rationing of nursing care inSwiss acute care hospitals a cross sectional study. International Journal of NursingStudies, 50(2), 230–239.
Sikorska, K., Lesaffre, E., Groenen, P.F.J. and Eilers, P.H.C. (2013). GWAS on your
notebook: fast semi-parallel linear and logistic regression for genome-wide associa-tion studies. BMC Bioinformatics, 14(1).
Sikorska, K., Rivadeneira, F., Groenen, P.J.F., Hofman, A., Uitterlinden, A.G., Eilers,
P.H.C. and Lesaffre, E. (2013). Fast linear mixed model computations for genome-wide association studies with longitudinal data. Statistics in Medicine, 32(1), 165–180.
Slaets, L., Claeskens, G. and Silverman, B.W. (2013). Warping functional data in R and
C via a Bayesian multiresolution approach. Journal of Statistical Software, 55(3),1–22.
Smeets, A., Brouwers, B., Hatse, S., Laenen, A., Paridaens, R., Floris, G., Wildiers,
H., Christiaens, M.R. (2013). Circulating CCL5 levels in patients with breast can-cer : is there a correlation with lymph node metastasis? ISRN Immunology, doi:10.1155/2013/453561.
Smeets, A., Yoshihara, E., Laenen, A., Reynders, A., Soens, J., Wildiers, H., Paridaens,
R., Van Ongeval, C., Floris, G., Neven, P., Christiaens, M.R. (2013). Is the sentinellymph node biopsy more sensitive for the identification of positive lymph nodesin breast cancer than the axillary lymph node dissection? SpringerPlus, 275(2),doi:10.1186/2193-1801-2-275.
Song, T., Dong, Q., Sankoh, A.J., and Molenberghs, G. (2013). Evaluation of overall
treatment effect in MMRM. Journal of Biopharmaceutical Statistics, 23, 1281–1293.
Sweldens, C., Peeters, S., Van Limbergen, E., Janssen, H., Laenen, A., Patil, S., Van
Zee, K.J., Weltens, C. (2014). Local relapse after breast conserving therapy for insitu carcinoma: a European single center experience and external validation of theMSKCC nomogram. The Cancer Journal: JPPO, 20(1), 1–7.
Tharmaratnam, K. and Claeskens, G. (2013). A comparison of robust versions of the
AIC based on M, S and MM estimators. Statistics: A Journal of Theoretical andApplied Statistics, 47(1), 216–235.
Thilakarathne, P.J., Verbeke, G., Engelen, K., Marchal, K., and Lin, D. (2013). Identify-
ing differentially expressed genes in the absence of replication. International Journalof Bioinformatics Research and Applications, 9, 71–90.
Tomassetti, C., Geysenbergh, B., Meuleman, C., Timmerman, D., Fieuws, S., D'Hooghe,
T. (2013). External validation of the endometriosis fertility index (EFI) stagingsystem for predicting non-ART pregnancy after endometriosis surgery. Human Re-production, 28(5), 1280–1288.
Tomsin, K., Mesens, T., Molenberghs, G., Peeters, L., and Gyselaers, W. (2013). Char-
acteristics of heart, arteries, and veins in low and high cardiac output preeclampsia.
European Journal of Obstetrics & Gynecology and Reproductive Biology, 169, 218–222.
Topal, B., Fieuws, S. (2013). Pancreaticogastrostomy after pancreaticoduodenectomy?
Lancet Oncol., 14(9).
Topal, B., Fieuws, S., Aerts, R. et al. (2013). Pancreaticojejunostomy versus pancre-
aticogastrostomy reconstruction after pancreaticoduodenectomy for pancreatic orperiampullary tumours: a multicentre randomised trial. Lancet Oncol., 14, 655–662.
Van Binnebeek, S., Baete, K., Vanbilloen, B., Terwinghe, C., Koole, M., Mottaghy, F.M.,
Clement, P.M., Mortelmans, L., Haustermans, K., Van Cutsem, E., Verbruggen, A.,Bogaerts, K., Verslype, C., Deroose, C.M. (2014). Individualized dosimetry-basedactivity reduction of (90)Y-DOTATOC prevents severe and rapid kidney functiondeterioration from peptide receptor radionuclide therapy. Eur J Nucl Med Mol Imag-ing., 41(6), 1141–1157.
Van den Branden, S., Van den Broucke, S., Leroy, R., Declerck, D., Bogaerts, K., Hop-
penbrouwers, K. (2013). Effect evaluation of an oral health promotion intervention inpreschool children. European Journal of Public Health, doi:10.1093/eurpub/ckt204.
Van den Heede, K., Florquin, M., Bruyneel, L., Aiken, L., Diya, L., Lesaffre, E., Sermeus,
W. (2013). Effective strategies for nurse retention in acute hospitals: A mixedmethod study. International Journal of Nursing Studies, 50(2), 185–194.
Vande Voorde, J., Sabuncuoglu, S., Noppen, S., Hofer, A., Ranjbarian, F., Fieuws, S.,
Balzarini, J. and Liekens, S. (2014). Nucleoside-Catabolizing Enzymes in Mycoplasma-Infected Tumor Cell Cultures Compromise the Cytostatic Activity of the AnticancerDrug Gemcitabine. Journal of Biological Chemistry, 289, 13054–13065.
Vandewalle, E., Abego Pinto, L., Van Bergen, T., Spielberg, L., Fieuws, S., Moons, L.,
Spileers, W., Zeyen, T., Stalmans, I. (2013). Intracameral bevacizumab as an ad-junct to trabeculectomy: a 1-year prospective, randomised study. Br J Ophthalmol.,98(1), 73–78.
Vandewalle, G., Baeten, M., Bogaerts, K., Vandekerckhove, P., Compernolle, V. (2013).
Evaluation of 6 years of confidential unit exclusion at the Belgian Red Cross FlandersBlood Service. Vox Sanguinis, 106(4), 354–360.
Van Limbergen, E.J., Dok, R., Laenen, A., Hauben, E., Van den Weyngaert, D., Vo-
ordeckers, M., De Neve, W., Duprez, F., Nuyts, S. (2014). HPV-related oropharyn-geal cancers in Flanders (Belgium): A multicenter study. B-ENT, 10, 7–14.
Van Nieuwenhuyse, A., Burdorf, A., Crombez, G., Verbeke, G., Masschelein, R., Mairi-
aux, P., Moens, G. and the BelCoBack Study Group (2013). Sick leave due toback pain in a cohort of young workers. International Archives of Occupational andEnvironmental Health, 86, 887–899.
Veraverbeke, N., Gijbels, I. and Omelka, M. (2014). Pre-adjusted nonparametric estima-
tion of a conditional distribution function. Journal of the Royal Statistical Society,Series B, 76 (2), 399–438.
Verbeke, G. and Molenberghs, G. (2013). The gradient function for checking goodness-
of-fit of the random-effects distribution in mixed models. Biostatistics, 14, 477–490.
Verschuere, T. Altered galectin-1 serum levels in patients diagnosed with high-grade
glioma. Journal of Neuro-Oncology, 115(1), 9–17.
Wellens, N.I.H., Verbeke, G., Flamaing, J., Moons, P., Boonen, S., Tournoy, J., and
Milisen, K. (2013). Clinical changes in older patients during hospitalization: Re-sponsiveness of the MDS interRAI Acute Care instrument. Journal of the AmericanGeriatrics Society, 61, 799–804.
Willemsen, S.P., de Ridder, M., Hokken-Koelega, A., Eilers, P.H.C. and Lesaffre, E.
(2013). Modelling height for children born small for gestational age treated withgrowth hormone. Statistical Methods in Medical Research, 0962280212473320.
Yoshihara, E., Smeets, A., Laenen, A., Reynders, A., Soens, J., Van Ongeval, C., Moer-
man, P., Paridaens, R., Wildiers, H., Neven, P., Christiaens, M.R. (2013). Predictorsof axillary lymph node metastases in early breast cancer and their applicability inclinical practice. Breast, 22, 357–361.
Yusof, M.Y., Thevissen, P.W., Fieuws, S., Willems, G. (2014). Dental age estimation
in Malay children based on all permanent teeth types. Int J Legal Med., 128(2),329–333.
Refereed publications (in press, accepted for publication).
Aerts, C., Molenberghs, G., Kenward, M.G. and Neiner, C. (2014). The surface nitrogen
of a massive star and its oscillations, rotation, and magnetic field. AstrophysicalJournal, to appear.
Antoniadis, A., Gijbels, I. and Lambert-Lacroix, S. (2014). Penalized estimation in
additive varying coefficient models using grouped regularization. Statistical Papers,to appear.
Aregay, M., Shkedy, Z. and Molenberghs, G. (2014). Comparison of Additive and Mul-
tiplicative Bayesian Models for Longitudinal Count Data With Overdispersion Pa-rameters: A Simulation Study. Communications in Statistics, Computation andSimulation, to appear.
Aregay, M., Shkedy, Z., Molenberghs, G., David, M.-P., and Tibaldi, F. (2014). Model-
based estimation of long-term persistence of induced HPV antibodies; a flexiblesubject-specific approach. Journal of Biopharmaceutical Statistics, to appear.
De Boever, P., Wens, B., Forcheh, A.C., Valkenborg, D., Reynders, H., Nelen, V., Klein-
jans, J., Van Larebeke, N., Verbeke, G. and Schoeters, G. (2014). Longitudinalstudy of the blood transcriptome in healthy individuals: A baseline for applyinggene expression analysis in human biomonitoring campaigns. Genomics, to appear.
Donneau, A.F., Mauer, M., Lambert, P., Molenberghs, G. and Albert, A. (2014). Simulation-
based study comparing multiple imputation methods for non-monotone missing ordi-nal data in longitudinal settings. Journal of Biopharmaceutical Statistics, to appear.
Efendi, A. and Molenberghs, G. (2014). A multilevel model for hierarchical, repeated,
and overdispersed time-to-event outcomes and its estimation strategies. Journal ofBiopharmaceutical Statistics, to appear.
Efendi, A., Molenberghs, G., and Iddi, S. (2014). A Marginalized Combined Model for
Repeated, Overdispersed Time-to-event Outcomes. Communications in Statistics,to appear.
Efendi, A., Molenberghs, G., Njeru Njagi, E. and Dendale, P. (2014). A joint model for
longitudinal continuous and time-to-event outcomes with direct marginal interpre-tation. Biometrical Journal, to appear.
Geva, D., Shahar, D., Harris, T., Tepper, S., Molenberghs, G. and Friger, M., (2014).
Snapshot of statistical methods used in geriatric cohort studies: how we treat missingdata in publications? International Journal of Statistics in Medical Research, toappear.
Ivanova, A., Molenberghs, G. and Verbeke, G. (2014). A model for overdispersed hierar-
chical ordinal data. Statistical Modeling, to appear.
Kassahun, W., Neyens, T., Molenberghs, G., Faes C. and Verbeke, G. (2014). A joint
model for hierarchical continuous and zero-inflated overdispersed count data. Jour-nal of Statistical Computation and Simulation, to appear.
Kenward, M.G. and Molenberghs, G. (2014). A Taxonomy of Mixing and Outcome
Distributions Based on Conjugacy and Bridging. Communications in Statistics,Theory and Methods, to appear.
Loeys, T. and Molenberghs, G. (2014). The analysis of (negatively) correlated non-
Gaussian dyadic outcomes: non-multilevel-based alternatives? Psychological Meth-ods, to appear.
Mallinckrodt, C., Roger, J., Chuang-Stein, C., Molenberghs, G., O'Kelly, M., Ratitch, B.,
Xu, L., Gilbert, S., Mehrotra, D., Wolfinger, R., and Thijs, H. (2014). Recent devel-opments in the prevention and treatment of missing data. Therapeutic Innovation& Regulatory Science, to appear.
Njeru Njagi, E., Molenberghs, G., Rizopoulos, D., Verbeke, G., Kenward, M.G., Dendale,
P. and Willekens, K. (2014). A flexible joint-modelling framework for longitudinaland time-to-event data with overdispersion. Statistical Methods in Medical Research,to appear.
Oellerer, V., Croux, C. and Alfons, A. (2014). The influence function of penalized re-
gression estimators. Statistics, to appear.
Reynders, A., Molenberghs, G., Van Audenhove, C. and Kerkhof, A.J.F.M. (2014). Atti-
tudes and stigma in relation to help — seeking intentions for psychological problemsin low and high suicide rate regions. Social Psychiatry and Psychiatric Epidemiology,to appear.
Tomsin, K., Oben, J., Staelens, A., Mesens, T., Molenberghs, G. Peeters, L. and Gyse-
laers, W. (2014). Cardiovascular hemodynamics throughout normal pregnancy andpostpartum as measured by impedance cardiography. Acta Obstetrica et Gynecolog-ica Scandinavica, to appear.
Van Beirendonck, S., Driessen, B., Van Thielen, J. and Verbeke, G. (2014). The asso-
ciation between sow and piglet behavior. Journal of Veterinary Behavior: ClinicalApplications and Research, to appear.
Van der Borght, K., Verbeke, G. and van Vlijmen, H. (2014). Multi-model inference using
mixed effects from a linear regression based genetic algorithm. BMC Bioinformatics,to appear.
Van der Elst, W., Molenberghs, G., Van Boxtel, M.P.J. and Jolles, J. (2014). Estab-
lishing normative data for repeated cognitive assessment: a comparison of differentstatistical methods. Behavior Research Methods, to appear.
Books & Book Chapters (published).
Claeskens, G. (2013). Lack of fit, Graphics and Multilevel Model Diagnostics. In The
SAGE Handbook of Multilevel Modeling. Edited by Scott, M.A., Simonoff, J.S. andMarx, B.D., SAGE Publications Ltd, London, pp. 425–445.
Books & Book Chapters (in press, accepted for publication).
Claeskens, G. and Jansen, M. (2014). Model selection (and model averaging). In Inter-
national encyclopedia of social and behavioral sciences, second edition. Editor J.D.
Wright, Elsevier, Oxford. To appear.
Gijbels, I., Herrmann, K. and Sznajder, D. (2014). Flexible and dynamic modeling of
dependencies via copulas. In Lecture Notes in Statistics: Modeling and StochasticLearning for Forecasting in High Dimension, Editors: Anestis Antoniadis, XavierBrossat, Jean-Michel Poggi. Springer. To appear.
Pircalabelu, E., Claeskens, G. and Waldorp, L. (2014). Constructing Graphical Models
via the Focused Information Criterion. In: Lecture Notes in Statistics: Modeling andStochastic Learning for Forecasting in High Dimension, Editors: Anestis Antoniadis,Xavier Brossat, Jean-Michel Poggi. Springer. To appear.
Katholieke Universiteit Leuven, KUL-2
Refereed publications (published).
Braeken, J., Kuppens, P., De Boeck, P. and Tuerlinckx, F. (2013). Contextualized person-
ality questionnaires: A case for copulas in structural equation models for categoricaldata. Multivariate Behavioral Research, 48, 845–870.
Brans, K., Van Mechelen, I., Rim´e, B. and Verduyn, P. (2013). The relation between
social sharing and the duration of emotional experience. Cognition and Emotion,27, 1023–1041.
Bringmann, L.F., Scholte, H.S. and Waldorp, L.J. (2013). Matching structural, effective,
and functional connectivity: A comparison between structural equation modelingand ancestral graphs. Brain Connectivity, 3, 375–385.
Bulteel, K., Ceulemans, E., Thompson, R.J., Waugh, C.E., Gotlib, I.H., Tuerlinckx,
F. and Kuppens, P. (2014). DeCon: A tool to detect emotional concordance inmultivariate time series data of emotional responding. Biological Psychology, 98,29–42.
Bulteel, K., Wilderjans, T.F., Tuerlinckx, F. and Ceulemans, E. (2013). CHull as an
alternative to AIC and BIC in the context of mixtures of factor analyzers. BehaviorResearch Methods, 45, 782–791.
Ceulemans, E., Hubert, M. and Rousseeuw, P. (2013). Robust multilevel simultaneous
component analysis. Chemometrics and Intelligent Laboratory Systems, 129, 33–39.
Cho, S.J., De Boeck, P., Embretson, S. and Rabe-Hesketh, S. (2014). Additive multilevel
item structure models with random residuals: Item modeling for explanation anditem generation. Psychometrika, 79, 84–104.
Debeer, D. and Janssen, R. (2013). Modeling item-position effects within an IRT frame-
work. Journal of Educational Measurement, 50, 164–185.
De Roover, K., Ceulemans, E., Timmerman, M.E., Nezlek, J.B. and Onghena, P. (2013).
Modeling differences in the dimensionality of multiblock data by means of clusterwisesimultaneous component analysis. Psychometrika, 78, 648–668.
De Roover, K., Timmerman, M.E., Mesquita, B. and Ceulemans, E. (2013). Common
and cluster-specific simultaneous component analysis. PLoS ONE, 8, e62280, 1–14.
De Roover, K., Timmerman, M.E., Van Diest, I., Onghena, P. and Ceulemans, E. (2014).
Switching principal component analysis for modeling means and covariance changesover time. Psychological Methods, 19, 113–132.
De Roover, K., Timmerman, M.E., Van Mechelen, I. and Ceulemans, E. (2013). On the
added value of multiset methods for three-way data analysis. Chemometrics andIntelligent Laboratory Systems, 129, 98–107.
Dusseldorp, E. and Van Mechelen, I. (2014). Qualitative interaction trees: A tool to iden-
tify qualitative treatment-subgroup interactions. Statistics in Medicine, 33, 219–237.
Fried, E.I., Tuerlinckx, F. and Borsboom, D. (2014). Mental health: More than neuro-
biology. Nature, 508, 458–458.
alez, J., De Boeck, P. and Tuerlinckx, F. (2014). Linear mixed modelling for data
from a double mixed factorial design with covariates: A case-study on semanticcategorization response times. Journal of the Royal Statistical Society: Series C(Applied Statistics), 63, 289–302.
Ip, E., Molenberghs, G., Chen, S.H., Goegebeur, Y. and De Boeck, P. (2013). Function-
ally unidimensional item response models for multivariate binary data. MultivariateBehavioral Research, 48, 534–562.
Kadengye, D.T., Ceulemans, E. and Van den Noortgate, W. (2014).
hood analysis and multiple imputation for missing item scores in multilevel cross-classification educational data. Applied Psychological Measurement, 38, 61–80.
Koval, P., Ogrinz, B., Kuppens, P., van den Bergh, O., Tuerlinckx, F. and Stterlin, S.
(2013). Affective instability in daily life is predicted by resting heart rate variability.
PLoS ONE, 8, e81536, 1–10.
Kuppens, P., Tuerlinckx, F., Russell, J.A. and Barrett, L.F. (2013). The relation between
valence and arousal in subjective experience. Psychological Bulletin, 139, 917–940.
Meulders, M., Tuerlinckx, F. and Vanpaemel, W. (2013). Constrained multilevel la-
tent class models for the analysis of three-way three-mode binary data. Journal ofClassification, 30, 306–337.
Partchev, I., De Boeck, P. and Steyer, R. (2013). How much power and speed is measured
in this test? Assessment, 20, 242–252.
Pe, M.L., Vandekerckhove, J. and Kuppens, P. (2013). A diffusion model account of
the relationship between the emotional flanker task and rumination and depression.
Emotion, 13, 739–747.
Rodr´ıguez, S., Wilderjans, T.F., Sosa, N. and Bernik, D. (2013). Image texture analysis
and gas sensor array studies applied to vanilla encapsulation by octenyl succinicanhydride starches. Journal of Food Research, 2, 36–48.
Schouteden, M., Van Deun, K., Pattyn, S. and Van Mechelen, I. (2013). SCA with
rotation to distinguish common and distinctive information in linked data. BehaviorResearch Methods, 45, 822–833.
Timmerman, M.E., Ceulemans, E., De Roover, K. and Van Leeuwen, K. (2013). Subspace
K-means clustering. Behavior Research Methods, 45, 1011–1023.
van de Leemput, I.A., Wichers, M., Cramer, A.O.J., Borsboom, D., Tuerlinckx, F.,
Kuppens, P., van Nes, E.H., Viechtbauer, W., Giltay, E.J., Aggen, S.H., Derom, C.,Jacobs, N., Kendler, K. S., van der Maas, H.L.J., Neale, M.C., Peeters, F., Thiery,E., Zachar, P. and Scheffer, M. (2014). Critical slowing down as early warning forthe onset and termination of depression. Proceedings of the National Academy ofSciences, 111, 87–92.
Van Deun, K., Smilde, A.K., Thorrez, L., Kiers, H.A.L. and Van Mechelen, I. (2013).
Identifying common and distinctive processes underlying multiset data. Chemomet-rics and Intelligent Laboratory Systems, 129, 40–51.
Wichers, M., Borsboom, D., Tuerlinckx, F., Kuppens, P., Viechtbauer, W., van de Leem-
put, I. A., Kendler, K. S. and Scheffer, M. (2014). Reply to Bos and De Jonge:Between-subject data do provide first empirical support for critical slowing down indepression. Proceedings of the National Academy of Sciences, 111, E879, 1–1.
Wilderjans, T.F. and Ceulemans, E. (2013). Clusterwise Parafac to identify heterogene-
ity in three-way data. Chemometrics and Intelligent Laboratory Systems, 129, 87–97.
Refereed publications (in press, accepted for publication).
Bartlema, A., Lee, M.D., Wetzels, R. and Vanpaemel, W. (in press). A Bayesian hierar-
chical mixture approach to individual differences: Case studies in selective attentionand representation in category learning. Journal of Mathematical Psychology, toappear.
De Roover, K., Timmerman, M.E., De Leersnyder, J., Mesquita, B. and Ceulemans,
E. (in press). Whats hampering measurement invariance: Detecting non-invariantitems using clusterwise simultaneous component analysis. Frontiers in Psychology,to appear.
Doove, L.L., Dusseldorp, E., Van Deun, K. and Van Mechelen, I. (in press). A comparison
of five recursive partitioning methods to find person subgroups involved in meaning-ful treatment-subgroup interactions. Advances in Data Analysis and Classification,to appear.
Heylen, J., Verduyn, P., Van Mechelen, I. and Ceulemans, E. (in press). Variability in
anger intensity profiles: Structure and predictive basis. Cognition and Emotion, toappear.
Kadengye, D.T., Ceulemans, E. and Van den Noortgate, W. (in press). A generalized
longitudinal mixture IRT model for measuring differential growth in learning envi-ronments. Behavior Research Methods, to appear.
Magis, D. (in press). A note on weighted likelihood and Jeffreys modal estimation of
proficiency levels in polytomous item response models. Psychometrika, to appear.
Magis, D. (in press). On the asymptotic standard error of a class of robust estimators of
ability in dichotomous item response models. British Journal of Mathematical andStatistical Psychology, to appear.
Magis, D. and De Boeck, P. (in press). Type I error inflation in DIF identification with
Mantel-Haenszel: An explanation and a solution. Educational and PsychologicalMeasurement, to appear.
Pe, M.L., Kircanski, K., Thompson, R.J., Bringmann, L.F., Tuerlinckx, F., Mestdagh,
M., Mata, J., Jaeggi, S.M., Buschkuehl, M., Jonides, J., Kuppens, P. and Gotlib,I.H. (in press). Emotion network density in major depressive disorder. ClinicalPsychological Science, to appear.
Rodr´ıguez, S.D., Barletta, D.A., Wilderjans, T.F. and Bernik, D.L. (in press). Fast and
efficient food quality control using electronic noses: Adulteration detection achievedby Unfolded Cluster Analysis coupled with Time-Windows selection. Food AnalyticalMethods, to appear.
San Mart´ın, E., Gonz´
alez, J. and Tuerlinckx, F. (in press). On the unidentifiability of
the fixed-effects 3PL model. Psychometrika, to appear.
Schouteden, M., Van Deun, K., Wilderjans, T.F. and Van Mechelen, I. (in press). Per-
forming DISCO-SCA to search for distinctive and common information in linkeddata. Behavior Research Methods, to appear.
Verdonck, S. and Tuerlinckx, F. (in press). The Ising Decision Maker: A binary stochas-
tic network for choice response time. Psychological Review, to appear.
Books & Book Chapters (in press, accepted for publication).
Kruschke, J.K. and Vanpaemel, W. (in press). Bayesian estimation in hierarchical models.
In Oxford Handbook of Computational and Mathematical Psychology, J. Busemeyer,J. Townsend, Z.J. Wang and A. Eidels (Eds.), Oxford: Oxford University Press, toappear.
e catholique de Louvain, UCL
Refereed publications (published).
Autin, F., Freyermuth, J.M. and von Sachs, R. (2014). Block-threshold-adapted Esti-
mators via a Maxiset Approach. Scandinavian Journal of Statistics : theory andapplications, 41(1), 240–258.
Bauwens, L., Hafner, C. and Pierret, D. (2013). Multivariate volatility modeling of
electricity futures. Journal of Applied Econometrics, 28, 5, 743–761.
Bˇadin, L., Daraio, C. and Simar, L. (2013). How to measure the impact of environmental
factors in a nonparametric production model. European Journal of OperationalResearch, 223(3), 818–833.
adin, L., Daraio, C. and Simar, L. (2014). Explaining inefficiency in nonparametric
production models: the state of the art. Annals of Operations Research, 214, 1,5–30.
Chen, S.X. and Van Keilegom, I. (2013). Estimation in semiparametric models with
missing data. Annals of the Institute of Statistical Mathematics, 65, 4, 785–805.
Christiansen, M.C. and Denuit, M. (2013). Worst-case actuarial calculations consistent
with single- and multiple-decrement life tables. Insurance: Mathematics and Eco-nomics, 52(1), 1–5.
Dahlke, M., Breidt, J., Opsomer, J. and Van Keilegom, I. (2013). Nonparametric en-
dogenous post-stratification estimation. Statistica Sinica, 23, 1, 189–211.
Daouia, A. and Park, B. (2013) On Projection-type Estimators of Multivariate Isotonic
Functions. Scandinavian Journal of Statistics, 40, 2, 363–386.
Daraio, C. and Simar, L. (2014). Directional distances and their robust versions: Com-
putational and testing issues. European Journal of Operational Research, 237, 1,358–369.
de Carvalho, M., Oumow, B., Segers, J. and Warchol, M. (2013). A Euclidean Likelihood
Estimator for Bivariate Tail Dependence. Communications in Statistics: Theory andMethods, 42(7), 1176–1192.
Denuit, M. and Eeckhoudt, L. (2013). Improving your chances: A new result. Economics
Letters, 118, 3, 475–477.
Denuit, M. and Eeckhoudt, L. (2013). Risk attitudes and the value of risk transforma-
tions. International Journal of Economic Theory, 9, 3, 245–254.
Denuit, M., Eeckhoudt, L. and Jokung, O. (2013). Non-differentiable transformations
preserving stochastic dominance. Journal of the Operational Research Society, 64,9, 1441–1446.
Denuit, M., Eeckhoudt, L. and Schlesinger, H. (2013). When Ross meets Bell: The linex
utility function. Journal of Mathematical Economics, 49(2), 177–182.
Denuit, M., Haberman, S. and Renshaw, A.E. (2013). Approximations for quantiles of
life expectancy and annuity values using the parametric improvement rate approachto modelling and projecting mortality. European Actuarial Journal, 3(1), 191–201.
Denuit, M. and Mesfioui, M. (2013). A sufficient condition of crossing type for the
bivariate orthant convex order. Statistics & Probability Letters, 83(1), 157–162.
Denuit, M. and Mesfioui, M. (2013). Ordering Functions of Random Vectors, with Ap-
plication to Partial Sums. Journal of Theoretical Probability, 26(2), 474–479.
Denuit, M. and Rey, B. (2013). Another look at risk apportionment. Journal of Mathe-
matical Economics, 49(4), 335–343.
Dewe, W., Benoˆıt, A. and Legrand, C. (2013). Assessing vaccine efficacy in influenza
clinical trials: challenges and difficulties. Expert Review of Clinical Pharmacology,6(4), 403–411.
Dunker, F., Florens, J.P., Hohage, T., Johannes, J., Mammen, E. (2014). Iterative
estimation of solutions to noisy nonlinear operator equations in nonparametric in-strumental regression. Journal of Econometrics, 178(3), 444–455.
Faraz, A., Heuchenne, C., Saniga, E. and Foster, E. (2013). Monitoring delivery chains us-
ing multivariate control charts. European Journal of Operational Research, 228(1),282–289.
Florens, J.P., Simar, L. and Van Keilegom, I. (2013). Frontier estimation in nonpara-
metric location-scale models. Journal of Econometrics, 178(3), 456–470.
Forget, P., Machiels, J.P., Coulie, P., Berli ere, M., Poncelet, A., Tombal, B., Stainier,
A., Legrand, C., Canon, J.L., Kremer, Y. and De Kock, M. (2013). Neutrophil:Lymphocyte Ratio and Intraoperative Use of Ketorolac or Diclofenac are Prognos-tic Factors in Different Cohorts of Patients Undergoing Breast, Lung, and KidneyCancer Surgery. Annals of Surgical Oncology, 20(3), S650–660.
Francq, B. and Govaerts, B. (2014a). Hyperbolic confidence bands of errors-in-variables
regression lines applied to method comparison studies. Journal de la Soci´et´e Franaisede Statistique & Revue de Statistique Appliqu´ee, 155(1), 23–45.
Francq, B. and Govaerts, B. (2014b). Measurement methods comparison with errors-
in-variables regressions. From horizontal to vertical OLS regression, review andnew perspectives. In: Chemometrics and Intelligent Laboratory Systems, 134(1),123–139.
Grothe, O., Schnieders, J. and Segers, J. (2013). Measuring association and dependence
between random vectors. Journal of Multivariate Analysis, 123, 91–110.
Heuchenne, C., Laurent, S., Legrand, C. and Van Keilegom, I. (2014). Likelihood-Based
Inference for Semi-Competing Risks. Communications in Statistics - Simulation andComputation, 43(5), 1112–1132.
Jaeger, J. and Lambert, P. (2013). Bayesian P-spline estimation in hierarchical mod-
els specified by systems of affine differential equations. Statistical Modelling : aninternational journal, 13(1), 3–40.
Johannes, J. and Schenk, R. (2013). On rate optimal local estimation in functional linear
regression. Electronic Journal of Statistics, 7, 191–216.
Johannes, J. and Schwarz, M. (2013). Adaptive Gaussian Inverse Regression with Par-
tially Unknown Operator. Communications in Statistics - Theory and Methods,42(7), 1343–1362.
Johannes, J., Van Bellegem and S., Vanhems, A. (2013). Iterative regularisation in non-
parametric instrumental regression. Journal of Statistical Planning and Inference,143(1), 24–39.
Klein, N., Denuit, M., Lang, S. and Kneib, T. (2014). Nonlife ratemaking and risk man-
agement with Bayesian generalized additive models for location, scale, and shape.
Insurance: Mathematics and Economics, 55, 225–249.
Loeys T., Legrand C., Schettino A. and Pourtois G. (2014). Semi-parametric proportional
hazards models with crossed random effects for psychometric response times. Br JMath Stat Psychol, 43(5), 1112–1132.
Lopez, O., Patilea, V. and Van Keilegom, I. (2013). Single index regression models in
the presence of censoring depending on the covariates. Bernoulli, 19(3), 721–747.
Meira-Machado, L., Roca-Pardi˜
nas, J., Van Keilegom, I. and Cadarso-Su´arez, C. (2013).
Bandwidth selection for the estimation of transition probabilities in the location-scale progressive three-state model. Computational Statistics, 28(5), 2185–2210.
Moreira, C. and Van Keilegom, I. (2013). Bandwidth selection for kernel density esti-
mation with doubly truncated data. Computational Statistics & Data Analysis, 61,107–123.
Noh, H., El Ghouch, A. and Van Keilegom, I. (2013a). Assessing model adequacy in
possibly misspecified quantile regression. Computational Statistics & Data Analysis,57(1), 558–569.
Noh, H., El Ghouch, A. and Van Keilegom, I. (2013b). Quality of fit measures in the
framework of quantile regression. Scandinavian Journal of Statistics : theory andapplications, 40(1), 105–118.
Pigeon, M., Antonio, K. and Denuit, M. (2013). Individual Loss Reserving with the
Multivariate Skew Normal Framework. ASTIN Bulletin, 43(3), 399–428.
Rotolo, F., Legrand, C. and Van Keilegom, I. (2013). A simulation procedure based
on copulas to generate clustered multi-state survival data. Computer methods andprograms in biomedicine, 109(3), 305–312.
Schwarz, M., Jongbloed, G. and Van Keilegom, I. (2013). On the identifiability of copulas
in bivariate competing risks models. Canadian Journal of Statistics, 41(2), 291–303.
Segers, J. and Uyttendaele, N. (2013). Nonparametric estimation of the tree structure
of a nested Archimedean copula. Computational Statistics & Data Analysis, 72,190–204.
Simar, L. and Wilson, P. (2013). Estimation and Inference in Nonparametric Frontier
Models: Recent Developments and Perspectives. Foundations and Trends in Econo-metrics, 5(3-4), 183–337.
Timmermans, C., Delsol, L., von Sachs, R. (2013). Using Bagidis in nonparametric
functional data analysis: Predicting from curves with sharp local features. Journalof Multivariate Analysis, 115, 421–444.
Wang, L., Kai, B., Heuchenne, C. and Tsai, C.L. (2013). Penalized profiled semipara-
metric estimating functions. Electronic Journal of Statistics, 7, 2656–2682.
Wei, J., Carroll, R., M¨
uller, U., Van Keilegom, I. and Chatterjee, N. (2013). Robust
estimation for homoscedastic regression in the secondary analysis of casecontrol data.
Journal of the Royal Statistical Society. Series B, Statistical methodology, 75(1),185–206.
Wunsch, G., Mouchart, M. and Russo, F. (2014). Functions and Mechanisms in Structural-
Modelling Explanations. Journal for General Philosophy of Science, 45(1), 187–208.
Yang, S. J., El Ghouch, A. and Van Keilegom, I. (2014). Varying coefficient models hav-
ing different smoothing variables with randomly censored data. Electronic Journalof Statistics, 8, 226–252.
Refereed publications (in press, accepted for publication).
ucher, A., Segers, J. and Volgushev, S. (2014). When uniform weak convergence fails:
empirical processes for dependence functions via epi- and hypographs. The Annalsof Statistics, to appear.
Janssen, A. and Segers, J. (2014). Markov Tail Chains. Advances in Applied Probability,
andez, J. C., Jim´enez-Gamero, M. D. and El Ghouch, A. (2014). A Non-
parametric ANOVA-type Test for Regression Curves Based on Characteristic Func-tions. Scandinavian Journal of Statistics, to appear.
Segers, J., van den Akker, R. and Werker, B. (2014). Semiparametric Gaussian copula
models: Geometry and efficient rank-based Estimation. The Annals of Statistics, toappear.
Books & Book Chapters (published).
Bonaccorsi, A., Daraio, C. and Simar, L. (2014). Scale and research specialization in
European Universities: a directional distance approach to teaching efficiency. In:Knowledge, Diversity and Performance in European Higher Education: A ChangingLandscape, A. Bonaccorsi (Ed.), pp. 292–311. Edward Elgar Publishing Limited:Cheltenham, UK.
Denuit, M., Eeckhoudt, L., Tsetlin, I. and Winkler, R. (2013). Multivariate Concave
and Convex Stochastic Dominance. In: Risk Measures and Attitudes (EuropeanActuarial Academy Series), F. Biagini, A. Richter and H. Schlesinger (Eds.), pp.
11–32, Springer-Verlag: London.
uller, U. and Van Keilegom, I. (2014). Efficient Quantile Regression with Auxiliary
Information. In Contemporary Developments in Statistical Theory (A Festschrift forHira Lal Koul) (Springer Proceedings in Mathematics & Statistics; 68), S. Lahiri, A.
Schick, A. SenGupta and T.N. Sriram (Eds.), pp. 365–374. Springer InternationalPublishing: Switzerland.
Universiteit Gent, UG
Refereed publications (published).
Baele, G., Lemey, P., and Vansteelandt, S. (2013). Make the most of your samples:
Bayes factor estimators for high-dimensional models of sequence evolution. BMCBioinformatics, 14, Article 85.
Buyze, J., and Goetghebeur, E. (2013). Crossover studies with survival outcomes. Sta-
tistical Methods in Medical Research, 22, 612–629.
Claesen, J., Clement, L., Shkedy, Z., Foulqui-Moreno, M.R. and Burzykowski, T. (2013).
Simultaneous mapping of multiple gene loci with pooled segregants. PLoS ONE,8(2), e55133.
De Neve, J., Thas, O. and Ottoy, J.P. (2013). Goodness-of-Fit methods for probabilistic
index models. Communications in Statistics - Theory and Methods, 42, 1193–1207.
De Neve, J., Thas, O., Ottoy, J.P. and Clement, L. (2013b).
An extension of the
Wilcoxon-Mann-Whitney test for analyzing RT-qPCR data. Statistical Applicationsin Genetics and Molecular Biology, 13, 333–346.
De Roy, K., Marzorati, M., Negroni, A., Thas, O., Balloi, A., Fava, F., Verstraete, W.,
Daffonchio, D. and Boon, N. (2013). Environmental Conditions and CommunityEveness Determine the Outcome of Biological Invasion. Nature Communications, 4,1383.
De Ruyck, K., Duprez, F., Werbrouck, J., Sabbe, N., De Lange, S., Boterberg, T.,
Madani, I., Thas, O., De Neve, W. and Thierens, H. (2013). A predictive model fordysphagia following IMRT for head and neck cancer: Introduction of the EMLassotechnique. Radiotherapy and Oncology, 107, 295–299.
Duchateau, L., Getachew, Y., Asale, A., Speybroeck, N. and Yewhalaw, D. (2013).
Avoiding the trap of nonrandomisation in evaluating mosquito trapping. Pathogensand Global Health, 107(8), 437.
Forcheh, A. C., Verbeke, G., Kasim, A., Lin, D., Shkedy, Z., Talloen, W. Ghlmann, H.
and Clement, L. (2013). beadarrayFilter: An R package to filter beads. R Journal,5(1).
Getachew, Y., Janssen, P., Yewhalaw, D., Speybroeck, N., Duchateau, L. (2013). Coping
with time and space in modelling malaria incidence: a comparison of survival andcount regression models. Statistics in Medicine, 32, 3224–3233.
Loeys, T., Moerkerke, B., De Smet, O., Buysse, A., Steen, J. and Vansteelandt, S.
(2013). Flexible mediation analysis in the presence of non-linear relations: beyondthe mediation formula. Multivariate Behavioral Research, 48, 871–894.
Lutz, S., Vansteelandt, S. and Lange, C. (2013). Testing for direct genetic effects using
a screening step in family-based association studies. Frontiers in Applied GeneticEpidemiology, 4, 243.
Martinussen, T. and Vansteelandt, S. (2013). A note on collapsibility and confounding
bias in Cox and Aalen regression models. Lifetime Data Analysis, 19, 279–296.
Mensaert, K., Denil, S., Trooskens, G., Van Criekinge, W., Thas, O. and De Meyer, T.
(2014). Next-Generation Technologies and Data Analytical Approaches for Epige-nomics. Environmental and Molecular Mutagenesis, 55, 155–170.
Mereta, S.T., Yewhalaw, D., Boets, P., Ahmed, A., Duchateau, L., Speybroeck, N.,
Vanwambeke, S.O., Legesse, W., De Meester, L. and Goethals, P.L.M. (2013).
Physico-chemical and biological characterization of anopheline mosquito larval habi-tats (Diptera: Culicidae): implications for malaria control. Parasites and Vectors,6, 320.
Rayner, J.C.W., Thas, O., Pipelers, P. and Beh, E. (2013). Calculating Bivariate Or-
thonormal Polynomials by Recurrence. Australian and New Zealand Journal ofStatistics, 55, 15–24.
Sabbe, N., Thas, O. and Ottoy, J.P. (2013). EMLasso: Logistic Lasso with Missing Data.
Statistics in Medicine, 32, 3143–3157.
Sinova, B., Van Aelst, S., Lopez, M.T. and Gil, M.A. (2014). A parameterized L2 metric
between fuzzy numbers and its parameter interpretation. Fuzzy Sets and Systems,245, 101–115.
Van Aelst, S. and Willems, G. (2013). Fast and robust bootstrap for multivariate infer-
ence: the R package FRB. Journal of Statistical Software, 53(3), 1–32.
VanderWeele, T. and Vansteelandt, S. (2013). Mediation Analysis with Multiple Media-
tors. Epidemiologic Methods, 2, 95–115.
VanderWeele, T. and Vansteelandt, S. (2014). Invited Commentary: Some Advantages
of the Relative Excess Risk due to Interaction (RERI)–Towards Better Estimatorsof Additive Interaction. American Journal of Epidemiology, 179, 670–671.
VanderWeele, T., Vansteelandt, S. and Robins, J.M. (2014). Effect decomposition in the
presence of an exposure-induced mediator-outcome confounder. Epidemiology, 25,300–306.
Vansteelandt, S., Martinussen, T. and Tchetgen Tchetgen, E. (2014). On adjustment
for auxiliary covariates in additive hazard models for the analysis of randomizedexperiments. Biometrika, 101, 237–244.
Yang, Y., Foulqui-Moreno, M., Clement, L., Erdei, E., Tanghe, A., Schaerlaekens, K.,
Dumortier, F. and Thevelein, J. M. (2013). QTL analysis of high thermotolerancewith superior and downgraded parental yeast strains reveals new minor QTLs andconverges on novel causative alleles involved in RNA processing. PLoS genetics,9(8), e1003693.
Yewhalaw, D., Getachew, Y., Tushune, K., Michael, K.W., Kassahun, W., Duchateau,
L. and Speybroeck, N. (2013). The effect of dams and seasons on malaria incidenceand anopheles abundance in Ethiopia. BMC Infectious Diseases 13, 161.
Refereed publications (in press, accepted for publication).
Bartlett, J.W., Tilling, K., Carpenter, J.R. and Vansteelandt, S. (2014). Improving upon
complete case analysis with covariates MNAR. Biostatistics, to appear.
De Neve, J., Meys, J., Ottoy, J.P., Clement, L. and Thas, O. (2014). unifiedWMWqPCR:
the unified Wilcoxon-Mann-Whitney test for analyzing RT-qPCR data in R. Bioin-formatics, accepted for publication.
Ho, A., De Roy, K., Thas, O., De Neve, J., Hoefman, S., Vandamme, P., Heylen, K. and
Boon, N. (2014). The more, the merrier: heterotroph richness stimulates methan-otrophic activity. The ISME Journal, accepted for publication.
Loeys, T. Moerkerke, B., Raes, A., Rosseel, Y. and Vansteelandt, S. (2014). Estimation of
controlled direct effects in the presence of exposure-induced confounding and latentvariables. Structural Equation Modeling, to appear.
Moerkerke, B., Loeys, T. and Vansteelandt, S. (2014). Structural Equation Modeling
versus Marginal Structural Modeling for assessing mediation in the presence of post-treatment confounding. Psychological Methods, to appear.
Stckl, D., Van Uytfanghe, K., Van Aelst, S., and Thienpont, L.M. (2014). A statistical
basis for harmonization of thyroid stimulating hormone immunoassays using a robustfactor analysis model. Clinical Chemistry and Laboratory Medicine, accepted forpublication.
Van Aelst, S. (2014). Stahel-Donoho Estimation for High-Dimensional Data. Interna-
tional Journal of Computer Mathematics, accepted for publication.
Vansteelandt, S. and Daniel, R.M. (2014). On regression adjustment for the propensity
score. Statistics in Medicine, to appear.
Varewyck, M., Goetghebeur, E., Eriksson, M. and Vansteelandt, S. (2014). On shrinkage
and model extrapolation in the evaluation of clinical center performance. Biostatis-tics, to appear.
Books & Book Chapters (in press, accepted for publication).
Rotnitzky, A. and Vansteelandt, S. (2014). Double-robust methods. In Handbook of
Missing Data, to appear.
Vansteelandt, S. (2014). Semi-parametric sensitivity analysis. In Handbook of Missing
Data, to appear.
Universiteit Hasselt, UH
Refereed publications (published).
Abrams, S., Beutels, P. and Hens, N. (2014). Assessing Mumps Outbreak Risk in Highly
Vaccinated Populations Using Spatial Seroprevalence Data. American Journal ofEpidemiology, 179(8), 1006–1017.
Aerts, C., Molenberghs, G., Kenward, M.G. and Neiner, C. (2014). The surface nitrogen
abundance of a massive star in relation to its oscillations, rotation, and magneticfield. The Astrophysical Journal, 781, 2.
Alqerban, A., Hedesiu, M., Baciut, M., Nackaerts, O., Jacobs, R., Fieuws, S., SedentexCT
Consortium, Willems, G., (2013). Pre-surgical treatment planning of maxillary ca-nine impactions using panoramic vs cone beam CT imaging. DentomaxillofacialRadiology, 42, 9.
Andraud, M., Hens, N. and Beutels, P. (2013). A simple periodic-forced model for dengue
fitted to incidence data in Singapore. Mathematical Biosciences, 244(1), 22–28.
Andriyana, Y., Gijbels, I. and Verhasselt, A. (2014). P–splines quantile regression esti-
mation in varying coefficient models. Test, 23, 153–194.
Anumendem, D., Verbeke, G., De Fraine, B., Onghena, P. and Van Damme, J. (2013).
Double serial correlation for multilevel growth curve models. Quality and Quantity,47, 3, 1413–1427.
Apers, S., Moons, P., Goossens, E., Luyckx, K., Gewillig, M., Bogaerts, K. and Budts,
W. (2013). Sense of coherence and perceived physical health explain the betterquality of life in adolescents with congenital heart disease. European Journal ofCardiovascular Nursing, 12(5), 475–483.
Ayele, B., Lipkovich, I., Molenberghs, G. and Mallinckrodt, C. (2014). A multiple–
imputation–based approach to sensitivity analyses and effectiveness assessments inlongitudinal clinical trials. Journal of biopharmaceutical statistics, 24, 2, 211–28.
Arain, A., Bourgeois, S., de Galocsy, C., Deltenre, P., Henrion, J., DHeygere, F.G.,
Georges, C., Bastens, B., Van Overbeke, L., Verrando, R., Bruckers, L., Mathei, C.,Buntinx, F., Van Vlierberghe, H., Francque, S., Laleman, W., Moreno, C., Nevens,F. and Robaeys, G. (2013). The Belgian experience in treatment of persons whoused drugs with the new standard of care in genotype 1 HCV infected patients: aninterim analysis. Hepatology, 58, S1, 1134A–1135A.
Aregay, M., Shkedy, Z. and Molenberghs, G. (2013). A hierarchical Bayesian approach
for the analysis of longitudinal count data with overdispersion: A simulation study.
Computational Statistics & Data Analysis, 57(1), 233–245.
Aregay, M., Shkedy, Z. and Molenberghs, G. (2013). Comparison of additive and multi-
plicative bayesian models for longitudinal count data with overdispersion parameters:a simulation study. Communications in Statistics: Simulation and Computation.
Aregay, M., Shkedy, Z., Molenberghs, G., David, M. and Tibaldi, F. (2013) Model based
estimation of long–term persistence of induced HPV antibodies; a flexible subject–specific approach. Journal of Biopharmaceutical Statistics, 23(6), 1228–1248.
Armstrong, P.W., Gershlick, A.H., Goldstein, P., Wilcox, R., Danays, T., Lambert, Y.,
Sulimov, V., Rosell Ortiz, F., Ostojic, M., Welsh, R.C., Carvalho, A.C., Nanas, J.,Arntz, H.-R., Halvorsen, S., Huber, K., Grajek, S., Fresco, C., Bluhmki, E., Regelin,A., Vandenberghe, K., Bogaerts, K. and Van de Werf, F. (2013). Fibrinolysis orPrimary PCI in ST-Segment Elevation Myocardial Infarction. New England Journalof Medicine, 368(15), 1379–1387.
Azmon, A., Faes, C. and Hens, N., (2014). On the estimation of the reproduction number
based on misreported epidemic data. Statistics in Medicine, 33(7), 1176–1192.
Beauclair, R., Meng, F., Deprez, N., Temmerman, M., Welte, A., Hens, N. and Delva, W.
(2013). Evaluating audio computer assisted self-interviews in urban south Africancommunities: evidence for good suitability and reduced social desirability bias of across-sectional survey on sexual behaviour. BMC, Medical Research Methodology,13, 11.
Bervoets, L., Van Hoorenbeeck, K., Kortleven, I., Van Noten, C., Hens, N., Vael, C.,
Desager, K.N., Goossens, H. and Vankerckhoven, V. (2013). Differences in gutmicrobiota composition between obese and lean children: a cross-sectional study.
Gut Pathogens, 5(10), 1–10.
Biermans, G., Horemans, N., Hens, N., Vives i Battle, J., Vandenhove, H. and Cuypers,
A. (2014). A dynamic dosimetry model for radioactive exposure scenarios in Ara-bidopsis thaliana. Journal of Theoretical Biology, 347, 54–62.
Blommaert, A., Coenen, S., Gielen, B., Goossens, H., Hens, N. and Beutels, P. (2013).
Patient and prescriber determinants for the choice between amoxicillin and broader-spectrum antibiotics: a nationwide prescription-level analysis. Journal of Antimi-crobial Chemotherapy, 68(10), 2383–2392.
Blommaert, A., Hens, N. and Beutels, P. (2014). Data mining for longitudinal data
under multicollinearity and time dependence using penalized generalized estimatingequations. Computational Statistics & Data Analysis, 71, 667–680.
Blommaert, A., Marais, C., Hens, Niel. Coenen, S., Muller, A., Goossens, H. and Beutels,
P. (2014). Determinants of between–country differences in ambulatory antibiotic useand antibiotic resistance in Europe: a longitudinal observational study. Journal ofAntimicrobial Chemotherapy, 69(2), 535–547.
Bollaerts, K., Antoine, J., Van Casteren, V., Ducoffre, G., Hens, N. and Quoilin, S.
(2013). Contribution of respiratory pathogens to influenza-like illness consultations.
Epidemiology and Infection, 141(10), 2196–2204.
Braeckman, T., Theeten, H., Lernout, T., Hens, N., Roelants, M., Hoppenbrouwers,
K. and Van Damme, P. (2014). Rotavirus vaccination coverage and adherence torecommended age among infants in Flanders (Belgium) in 2012. Eurosurveillance,19, 20.
Bruckers, L., de Mulder, K. Vundelinckx, B.J., De Schepper, J. and Van Esbroeck, G.
(2013). Functional and Radiographic Short-Term Outcome Evaluation of the Vi-sionaire System, a Patient-Matched Instrumentation System for Total Knee Arthro-plasty. The Journal of Arthroplasty, 28(6), 964–970.
Bruyndonckx, R., Hens, N., Aerts, M., Goossens, H., Molenberghs, G. and Coenen, S.
(2014). Measuring trends of outpatient antibiotic use in Europe: jointly modellinglongitudinal data in defined daily doses and packages. Journal of AntimicrobialChemotherapy.
Buyse, M. and Michiels, S. (2013). Omics-based clinical trial designs. Current Opinion
in Oncology, 25(3), 289–295.
Castro, M., Sanchez, L., Perez, D., Sebrango Rodriguez, C., Shkedy, Z. and Van der
Stuyft, P. (2013). The Relationship between Economic Status, Knowledge on Dengue,Risk Perceptions and Practices. PLoS ONE, 8, 12.
Castro Sanchez, A.Y., Aerts, M., Shkedy, Z., Vickerman, P., Faggiano, F., Salamina, G.
and Hens, N. (2013). A mathematical model for HIV and hepatitis C co-infectionand its assessment from a statistical. Epidemics, 5(1), 56–66.
Ciani, O., Buyse, M., Garside, R., Pavey, T., Stein, K., Sterne, J.A., C. and Taylor,
R.S. (2013). Comparison of treatment effect sizes associated with surrogate andfinal patient relevant outcomes in randomised controlled trials: meta-epidemiologicalstudy. British Medical Journal, 346, Article f457.
Claesen, J., Clement, L., Shkedy, Z., Foulqui-Moreno, M.R., and Burzykowski, T. (2013).
Simultaneous Mapping of Multiple Gene Loci with Pooled Segregants. PloS ONE,8(2) ,(e55133), 1-9.
Coenen, S., Gielen, B., Blommaert, A., Beutels, P., Hens, N. and Goossens, H. (2014).
Appropriate international measures for outpatient antibiotic prescribing and con-sumption: recommendations from a national data comparison of different measures.
Journal of Antimicrobial Chemotherapy, 69(2), 529–534.
Croes, K., Colles, A., Koppen, G., De Galan, S., Vandermarken, T., Govarts, E., Bruck-
ers, L., Nelen, V., Schoeters, G., Van Larebeke, N., Denison, M., S., Mampaey, M.,and Baeyens, W. (2013). Determination of PCDD/Fs, PBDD/Fs and dioxin-likePCBs in human milk from mothers residing in the rural areas in Flanders, using theCALUX bioassay and GC-HRMS. Talanta, 113, 99–105.
Croes, K., De Coster, S., De Galan, S., Morrens, B., Loots, I., Van de Mieroop, E., Nelen,
V., Sioen, I., Bruckers, L., Nawrot, T., Colles, A., Den Hond, E., Schoeters, G., vanLarebeke, N., Baeyens, W., Gao Y. (2013). Health effects in the Flemish populationin relation to low levels of mercury exposure: From organ to transcriptome level.
International Journal of Hygiene and Environmental Health, 217(2-3), 239–247.
Cuypers, K., Leenus, D., van den Berg, F.E., Levin, O., Thijs, H., Swinnen, S. and
Meesen, R. (2013). Long-term TENS treatment decreases cortical motor represen-tation in multiple sclerosis. Neuroscience, 250, 1–7.
Cuypers, K., Leenus, D., van den Berg, F.E., Nitsche, M.A., Thijs, H., Wenderoth, N.
and Meesen, R.L., J. (2013). Is Motor Learning Mediated by tDCS Intensity? PLoSONE, 8, 6.
Cuypers, K., Leenus, D., Van Wijmeersch, B., Thijs, H., Levin, O., Swinnen, S. and
Meesen, R. (2013). Anodal tDCS increases corticospinal output and projectionstrength in multiple sclerosis. Neuroscience Letters, 554, 151-155.
Cuypers, K., Thijs, H. and Meesen, R. (2014). Optimization of the Transcranial Magnetic
Stimulation Protocol by Defining a Reliable Estimate for Corticospinal Excitability.
PLoS ONE, 9, 1.
Cuypers, K., Thijs, H., Duque, J., Swinnen, S.P., Oron, L. and Meesen, R.L.J. (2013).
Age-related differences in corticospinal excitability during a choice reaction timetask. AGE, 35(5), 1705–1719.
De Boever, P., Wens, B., Forcheh, Anyiawung C., Reynders, H., Nelen, V., Kleinjans, J.,
Van Larebeke, N., Verbeke, G., Valkenborg, D. and Schoeters, G. (2014). Charac-terization of the peripheral blood transcriptome in a repeated measures design usinga panel of healthy individuals. Genomics, 103, 1, 31–39.
Dejardin, D. and Lesaffre, E. (2013). Stochastic EM algorithm for doubly interval-
censored data. Biostatistics, 14(4), 766–778.
Del Fava, E., Shkedy, Z., Aregay, M. and Molenberghs, G. (2013). Modeling multivari-
ate, overdispersed binomial data with additive and multiplicative random effects.
Statistical modelling, 14(2), 99–133.
Delva, W., Meng, F., Beauclair, R., Deprez, N., Temmerman, M., Welte, A. and Hens,
N. (2013). Coital frequency and condom use in monogamous and concurrent sex-ual relationships in Cape Town, South Africa. Journal of the International AIDSSociety, 16:18034.
Demaerschalk, M. F., Vanden Boer, L.E., Bronselaer, J.L., Molenberghs, G. and Declercq,
A. G. (2013). The influence of municipal characteristics on the use of informal homecare and home care services by the elderly Flemish. European Journal of PublicHealth, 23(2), 241–246.
De Neubourg, D., Bogaerts, K., Wyns, C., Albert, A., Camus, M., Candeur, M., Deguel-
dre, M., Delbaere, A., Delvigne, A., De Sutter, P., Dhont, M., Dubois, M., Englert,Y., Gillain, N., Gordts, S., Hautecoeur, W., Lesaffre, E., Lejeune, B., Leroy, F.,Ombelet, W., DHauterive, S., Perrier, Vandekerckhove, F., Van der Elst, J., andDHooghe, T. (2013). The history of Belgian assisted reproduction technology cycleregistration and control: a case study in reducing the incidence of multiple preg-nancy. Human Reproduction, 28(10), 2709–2719.
Den Hond, E., Paulussen, M., Geens, T., Bruckers, L., Baeyens, W., David, F., Du-
mont, E., Loots, I., Morrens, B., de Bellevaux, B.N., Nelen, V., Schoeters, G., VanLarebeke, N. and Covaci, A. (2013). Biomarkers of human exposure to personalcare products: Results from the Flemish Environment and Health Study (FLEHS2007-2011). Science of the Total Environment, 463, 102–110.
de Una-Alvarez, J. and Veraverbeke, N. (2013). Generalized copula-graphic estimator.
Test, 22(2), 343–360.
De Vocht, N., Lin, D., Praet, J., Hoornaert, C., Reekmans, K., Le Blon, D., Daans,
J., Pauwels, P., Goossens, H., Hens, N., Berneman, Z., Van der Linden, A. andPonsaerts, P. (2013). Quantitative and phenotypic analysis of mesenchymal stro-mal cell graft survival and recognition by microglia and astrocytes in mouse brain.
Immunobiology, 218(5), 696–705.
de Vries, J., Antoine, J-M., Burzykowski, T., Chiodini, A., Gibney, M., Kuhnle, G.,
Meheust, A., Pijls, L. and Rowland, I. (2013). Markers for nutrition studies: reviewof criteria for the evaluation of markers. European Journal of Nutrition, 52(7),1685–1699.
Dierickx, D., Tousseyn, T., Morscio, J., Fieuws, S. and Verhoef, G. (2013). Validation of
Prognostic Scores in Post-Transplantation Lymphoproliferative Disorders. Journalof Clinical Oncology, 31(27), 3443–3444.
Dierickx, D., Tousseyn, T., Sagaert, X., Fieuws, S., Wlodarska, I., Morscio, J., Brepoels,
L., Kuypers, D., Vanhaecke, J., Nevens, F., Verleden, G., Van Damme-Lombaerts,R., Renard, M., Pirenne, J., De Wolf-Peeters, C. and Verhoef, G. (2013). Single-center analysis of biopsy-confirmed posttransplant lymphoproliferative disorder: in-cidence, clinicopathological characteristics and prognostic factors. Leukemia & Lym-phoma, 54(11), 2433–2440.
Dittwald, P. and Valkenborg, D. (2014). BRAIN 2.0: Time and Memory Complexity
Improvements in the Algorithm for Calculating the Isotope Distribution. Journal ofthe American Society for Mass Spectrometry, 25(4), 588–594.
Dittwald, P., Claesen, J., Burzykowski, T., Valkenborg, D. and Gambin, A. (2013).
BRAIN: A Universal Tool for High-Throughput Calculations of the Isotopic Distri-bution for Mass Spectrometry. Analytical Chemistry, 85(4), 1991–1994.
Donneau, A., Mauer, M., Lambert, P., Molenberghs, G. and Albert, A. (2013). Simulation-
based study comparing multiple imputation methods for non–monotone missing or-dinal data in longitudinal settings. Journal of Biopharmaceutical Statistics.
Donneau, A., Mauer, M., Molenberghs, G. and Albert, A. (2014). A simulation study
comparing multiple imputation methods for incomplete longitudinal ordinal data.
Communications in Statistics: Simulation and Computation.
Dreesen, K., Kellens, A., Wevers, M., Thilakarathne, P. and Willems, G. (2013). The
influence of mixing methods and disinfectant on the physical properties of alginateimpression materials. European Journal of Orthodontics, 35(3), 381–387.
Drikvandi, R., Verbeke, G., Khodadadi, A. and Nia, Vahid Partovi (2013). Testing
multiple variance components in linear mixed-effects models. Biostatistics, 14, 1,144–159.
Duez, N., Eeckhoudt, D., Kirkpatrick, A., Solbu, G., Suciu, S., Sylvester, R., Van
Glabbeke, M., Buyse, M., Dalesio, O., Renard, J. and Rotmensz, N. (2013). Pro-fessor Maurice Staquet, MD, MS,(1930-2013) In Memoriam. Statistics in Medicine,32(30), 5219–5220.
Efendi, A. and Molenberghs G. (2013) A multilevel model for hierarchical, repeated,
overdispersed time-to-event outcomes and its estimation strategies. Journal of Bio-pharmaceutical Statistics, 23, 6, 1420–1434.
Efendi, A., Molenberghs, G. and Iddi, S. (2013) A marginalized combined model for re-
peated, overdispersed time-to-event outcomes. Communications in Statistics: The-ory and Methods.
Efendi, A., Molenberghs, G. and Iddi, S. (2013). A marginalized combined gamma
frailty and normal random-effects model for repeated, overdispersed time-to-eventoutcomes. Journal of Biopharmaceutical Statistics, 1–12.
Efendi, A., Molenberghs, G., Njagi, E. and Dendale, P. (2013). A joint model for longi-
tudinal continuous and time to event outcomes with direct marginal interpretation.
Biometrical Journal, 551(4), 572–588.
Ejigu, B.A., Valkenborg, D., Baggerman, G., Vanaerschot, M., Witters, E., Dujardin, J.-
C., Burzykowski, T. and Berg, M., (2013). Evaluation of normalization methods topave the way towards large-scale LC-MS-based metabolomic profiling experiments.
Omics-A Journal of Integrative Biology, 17(9), 473–485.
Ensoy, C., Aerts, M., Welby, S., Van der Stede, Y. and Faes, C. (2013). A Dynamic
Spatio-Temporal Model to Investigate the Effect of Cattle Movements on the Spreadof Bluetongue BTV-8 in Belgium. PLoS ONE, 8, 11.
Faes, C., van der Stede, Y., Guis, H., Staubach, C., Ducheyne, E., Hendrickx, G. and
Mintiens, K. (2013). Factors affecting Bluetongue serotype 8 spread in NorthernEurope in 2006: The geographical epidemiology. Preventive Veterinary Medicine,110(2), 149–158.
Forcheh Anyiawung, C., Verbeke, G., Kasim, A., Lin, D., Shkedy, Z., Talloen, W.,
Goehlmann, H. W. H. and Clement, L. (2013). beadarrayFilter: An R Packageto Filter Beads. R JOURNAL, 5(1), 171–180.
Geerdens, C., Claeskens, G. and Janssen, P. (2013). Goodness-of-fit tests for the frailty
distribution in proportional hazards models with shared frailty. Biostatistics, 14(3),433–446.
Geerdens, C., Vanderlinden, J., Pieters, G., De Herdt, A. and Probst, M. (2013). Missing
Data in Long-term Follow-up of Patients with Eating Disorders using the BodyAttitude Test. European Eating Disorders Review, 21(3), 224–229.
Getachew, Y., Janssen, P., Yewhalaw, D., Speybroeck, N. and Duchateau, L. (2013).
Coping with time and space in modelling malaria incidence: a comparison of survivaland count regression models. Statistics in Medicine, 32(18), 3224–3233.
Geva, D., Shahar, D., Harris, T. Tepper, S., Molenberghs, G. and Friger, M. (2013).
Snapshot of statistical methods used in geriatric cohort studies: how we treat missingdata in publications? International journal of statistics in medical research, 2(4),289–296.
Ghebretinsae, A., Molenberghs, G., Dmitrienko, A., Offen, W. and Sethuraman, G.
(2014). Assessment of type I error rate associated with dose group switching ina longitudinal alzheimer trial. Journal of Biopharmaceutical Statistics, 24(3), 660–684.
Ghebretinsae, A., Faes, C., Molenberghs, G., De Boeck, M. and Geys, H. (2013). A
Bayesian, generalized frailty model for comet assays. Journal of BiopharmaceuticalStatistics, 23(3), 618–636.
Gijbels, I., Herrmann, K. and Verhasselt, A. (2013). Discussion on "Large covariance
estimation by thresholding principal orthogonal complements". Journal of the RoyalStatistical Society Series B-Statistical Methodology, 75, 662–663.
alez Montoro, A., Cao, R., Faes, C., Molenberghs, G., Espinosa, N., Cudeiro, J.,
Marino, J. (2014). Cross nearest–spike interval based method to measure synchronydynamics. Mathematical Biosciences and Engineering, 11, 27–48.
Goossens, G., A., Jerome, M., Janssens, C., Peetermans, W.E., Fieuws, S., Moons, P.,
Verschakelen, J., Peerlinck, K., Jacquemin, M. and Stas, M. (2013). Comparingnormal saline versus diluted heparin to lock non-valved totally implantable venousaccess devices in cancer patients: a randomised, non-inferiority, open trial. Annalsof Oncology, 24(7), 1892–1899.
Grobler, A., Matthews, G. and Molenberghs, G. (2014). The impact of missing data on
clinical trials: a re–analysis of a placebo controllied trial of hypericum perforatum(St John's Wort) and sertraline in major depressive disorder. Psychopharmacology,231(9), 1987–1999.
Guzzetta, G., Poletti, P., Del Fava, E., Ajelli, M., Tomba, G.P.S., Merler, S. and Man-
fredi, P. (2013). Hope-Simpsons Progressive Immunity Hypothesis as a PossibleExplanation for Herpes Zoster Incidence Data. American Journal of Epidemiology,177(10), 1134–1142.
Hens, N., Ghebretinsae, A.H., Hardt. K., Van Damme, P. and Van Herck, K. (2014).
Model based estimates of long-term persistence of inactivated hepatitis: A vaccine-induced antibodies in adults. Vaccine, 32(13), 1507–1513.
Hompes, T., Izzi, B., Gellens, E., Morreels, M., Fieuws, S., Pexsters, A., Schops, G.,
Dom, M., Van Bree, R., Freson, K., Verhaeghe, J., Spitz, B., Demyttenaere, K.,Glover, V., Van den Bergh, B., Allegaert, K. and Claes, S. (2013). Investigating theinfluence of maternal cortisol and emotional state during pregnancy on the DNAmethylation status of the glucocorticoid receptor gene (NR3C1) promoter region incord blood. Journal of Psychiatric Research, 47(7), 880–891.
Hu, H., Dittwald, P., Zaia, J. and Valkenborg, D. (2013). Comment on "Computa-
tion of Isotopic Peak Center-Mass Distribution by Fourier Transform". AnalyticalChemistry, 85(24), 12189–12192.
Iddi, S. and Molenberghs, G. (2013). A marginalized model for zero–inflated, overdis-
persed and correlated count data. Electronic Journal of Applied Statistical Analysis,6, 149–165.
Ip, E., Molenberghs, G., Chen, S., Goegebeur, Y. and De Boeck, P. (2013). Functionally
Unidimensional Item Response Models for Multivariate Binary Data. MultivariateBehavioral Research, 48(4), 534–562.
Janssen, P., Swanepoel, J. and Veraverbeke, N. (2014). A note on the asymptotic be-
havior of the Bernstein estimator of the copula density. Journal of MultivariateAnalysis, 124, 480–487.
Jaspers, S., Aerts, M., Verbeke, G. and Beloeil, P.-A. (2013). A new semi-parametric
mixture model for interval censored data, with applications in the field of antimicro-bial resistance. Computational Statistics & Data Analysis, 71, 30–42.
Jaspers, S., Aerts, M., Verbeke, G. and Beloeil, P.-A. (2014). Estimation of the wild–
type minimum inhibitory concentration value distribution. Statistics in Medicine,33, 289–303.
Jombart, T., Aenensen, D.M., Baguelin, M., Birrell, P., Cauchemez, S., Camacho, A.,
Colijn, C., Collins, C., Cori, A., Didelot, X., Fraser, C., Frost, S., Hens, N., Hugues,J., Ho hle, M., Opatowski, L., Rambaut, A., Ratmann, O., Soubeyrand, S., Suchard,M.A., Wallinga, J., Ypma, R. and Ferguson, N. (2014). OutbreakTools: A newplatform for disease outbreak analysis using the R software. Epidemics, 7, 28–34.
Kalema, G., Iddi, S., and Molenberghs, G. (2014). The combined model: a tool for
simulating correlated counts with overdispersion. Communications in Statistics:Simulation and Computation.
Kassahun, W., Neyens, T., Molenberghs, G., Faes, C. and Verbeke, G. (2013). A joint
model for hierarchical continuous and zero-inflated overdispersed count data. Jour-nal of Statistical Computation and Simulation.
Kassahun W., Neyens T., Faes C., Molenberghs G. and Verbeke G. (2014). A zero–
inflated overdispersed hierarchical Poisson model. Statistical Modelling.
Kenward, M. G. and Molenberghs, G.(2013). A Taxonomy of Mixing and Outcome
Distributions Based on Conjugacy and Bridging. Communications in Statistics –Theory and Methods.
Kelchtermans, P., Bittremieux, W., De Grave, K., Degroeve, S., Ramon, J., Laukens, K.,
Valkenborg, D., Barsnes, H. and Martens, L. (2014). Machine learning applicationsin proteomics research: How the past can boost the future. Proteomics, 14(4-5),353–366.
Kenyon, C., Colebunders, R. and Hens, N. (2013). Determinants of generalized herpes
simplex virus-2 epidemics: the role of sexual partner concurrency. InternationalJournal of STD & AIDS, 24(5), 375–382.
Kenyon, C., Colebunders, R., Buve, A. and Hens, N. (2013). Partner-concurrency asso-
ciated with herpes simplex virus 2 infection in young South Africans. InternationalJournal of STD & AIDS, 24(10), 804–812.
Khamiakova, T., Shkedy, Z., Amaratunga, D., Talloen, W., Gohlmann, H., Bijnens, L.
and Kasim, A. (2014). Quality control of Platinum Spike dataset by probe–levelmixed models. Mathematical Biosciences, 248, 1–10.
Klingels, K., Feys, H., Molenaers, G., Verbeke, G., Van Daele, S., Hoskens, J., Desloovere,
K. and De Cock, P. (2013) Randomized Trial of Modified Constraint–Induced Move-ment Therapy With and Without an Intensive Therapy Program in Children WithUnilateral Cerebral Palsy. Neurorehabilitation and neural repair, 27(9), 799–807.
Laporte, S., Squifflet, P., Baroux, N., Fossella, F., Georgoulias, V., Pujol, J.-L., Douillard,
J.-Y., Kudoh, S., Pignon, J.-P., Quinaux, E. and Buyse, M. (2013). Prediction ofsurvival benefits from progression-free survival benefits in advanced non-small-celllung cancer: evidence from a meta-analysis of 2334 patients from 5 randomised trials.
BMJ OPEN, 3, 3.
Lermyte, F., Konijnenberg, A., Williams, J., Brown, JM., Valkenborg, D. and Sobott, F.
(2014). ETD Allows for Native Surface Mapping of a 150 kDa Noncovalent Complexon a Commercial Q–TWIMS–TOF Instrument. Journal of the American Society forMass Spectrometry, 25(3), 343–350.
Lernout, T., Theeten, H., Hens, N., Braeckman, T., Roelants, M., Hoppenbrouwers, K.
and Van Damme, P. (2014). Timeliness of infant vaccination and factors relatedwith delay in Flanders, Belgium. Vaccine, 32(2), 284–289.
Leroy, R., Bogaerts, K., Hoppenbrouwers, K., Martens, LC., and Declerck, D. (2013).
Dental attendance in preschool children - a prospective study. International Journalof Paediatric Dentistry, 23(2), 84–93.
Lin, D., Tilahun, A., Abrahantes, J.C., Shkedy, Z., Molenberghs, G., Gohlmann, H. W.
H., Talloen, W. and Bijnens, L. (2013). Comparison of methods for the selectionof genomic biomarkers. International Journal of Data Mining and Bioinformatics,8(1), 24–41.
Loeys, T. and Molenberghs, G. (2013). Modeling actor and partner effects in dyadic data
when outcomes are categorical. Psychological methods, 18(2), 220–236.
Loquiha, O., Hens, N., Chavane, L., Temmerman, M. and Aerts, M. (2013). Modeling
heterogeneity for count data: A study of maternal mortality in health facilities inMozambique. Biometrical Journal, 55(5), 647–660.
Luyten, J., Desmet, P., Dorgali, V., Hens, N. and Beutels, P. (2014). Kicking against
the pricks: vaccine sceptics have a different social orientation. European Journal ofPublic Health, 24(2), 310–314.
Luyten, J., Dorgali, V., Hens, N. and Beutels, P. (2013). Public preferences over ef-
ficiency, equity and autonomy in vaccination policy: An empirical study. SocialScience & Medicine, 77, 84–89.
Mackiewicz, J., Wysocki, P., Karczewska-Dzionka, A., Kwiatkowska-Borowczyk, E., Ko-
zlowska, A., Burzykowski, Tomasz. Rose-John, S., Wiznerowicz, M. and Mackiewicz,A. (2013). Immunotargeting melanoma stem cells with Hyper-IL6 modified wholecell therapeutic vaccine (AGI-101H) in patients with advanced melanoma - a jointanalysis of two phase 2 studies. European Journal of Cancer, 49, S870–S870.
Maes, E., Valkenborg, D., Mertens, I., Broeckx, V., Baggerman, G., Sagaert, X., Landuyt,
B., Prenen, H. and Schoofs, L. (2013). Proteomic analysis of formalin-fixed paraffin-embedded colorectal cancer tissue using tandem mass tag protein labeling. MolecularBiosystems, 9(11), 2686–2695.
Maleux, G., Gillardin, P., Fieuws, S., Heye, S., Vaninbroukx, J. and Nackaerts, K. (2013).
Large-Bore Nitinol Stents for Malignant Superior Vena Cava Syndrome: FactorsInfluencing Outcome. American Journal of Roentgenology, 201(3), 667–674.
Mallinckrodt, C., Lin, Q., Carpenter, J., Kenward, M. and Molenberghs G. (2013). A
structured framework for assessing sensitivity to missing data assumptions in longi-tudinal clinical trials. Pharmaceutical Statistics, 12(1), 1–6.
Mallinckrodt, C., Roger, J., Chuang-Stein, C., Molenberghs, G., Lane, P.W., Kelly, M.
O., Ratitch, B., Xu, L., Gilbert, S., Mehrotra, D. V., Wolfinger, R. and Thijs, H.
(2013). Missing Data: Turning Guidance Into Action. Statistics in Biopharmaceu-tical Research, 5(4), 369–382.
Mallinckrodt, C., Roger, J., Chuang–Stein, C., Molenberghs, G., O'Kelly, M., Ratitch,
B., Janssens, M. and Bunouf, I. (2014). Recent Developments in the Prevention andTreatment of Missing Data. Therapeutic Innovation & Regulation Science, 48(1),68–80.
Meesen, R., Thijs, H., Leenus, D. and Cuypers, K. (2013). A single session of 1mA anodal
tDCS-supported motor training does not improve motor performance in patientswith multiple sclerosis. Restorative Neurology and Neuroscience, 32, 293–300.
Minalu, G., Aerts, M., Coenen, S., Versporten, A., Muller, A., Adriaenssens, N., Beutels,
P., Molenberghs, G., Goossens, H. and Hens, N. (2013). Adaptive change-pointmixed models applied to data on outpatient tetracycline use in Europe. StatisticalModelling, 13(3), 253–274.
Molenberghs, G., and Iddi, S. (2013). A marginalized model for zero-inflated, overdis-
persed and correlated count data. Electronic Journal of Applied Statistical Analysis,6(2), 149–165.
Molenberghs, G., Alonso Abad, A., Van der Elst, W., Burzykowski, T. and Buyse, M.
(2013). Review: Clinical Trial Methodology: Surrogate endpoints: when shouldthey be used? Clinical Investigation, 3(12), 1147–1155.
Molenberghs, G., Kenward, M. G., Verbeke, G., Iddi, S. and Efendi, A. (2013). On
the Connections Between Bridge Distributions, Marginalized Multilevel Models, andGeneralized Linear Mixed Models. International Journal of Statistics and Probabil-ity, 2(4), 1–21.
Molenberghs, G., Kenward, M., Aerts, M., Verbeke, G., Tsiatis, A., Davidian, M. and Ri-
zopoulos, D. (2014). On random sample size, ignorability, ancillarity, completeness,separability, and degeneracy: sequential trials, random sample size, and missingdata. Statistical Methods in Medical Research, 23, 11–41.
Nguyen, T.T.V., Tran-Duy, A., Heldmann, F., Braun, J., Thijs, H. and Boonen, A.
(2013). Presenteeism Predicts Sick Leave Better Than Basdai and/Or BASFI In ALongitudinal Cohort Of Patients With Ankylosing Spondylitis (EASIC). Arthritisand Rheumatism, 65, S434–S434.
Njeru Njagi, E., Molenberghs, G., Rizopoulos, D., Verbeke, G., Kenward, M., Dendale, P.
and Willekens, K. (2013). A flexible joint–modelling framework for longitudinal andtime–to–event data with overdispersion. Statistical Methods in Medical Research.
Njeru Njagi, E., Rizopoulos, D., Molenberghs, G., Dendale, P. and Willekens, K. (2013).
A joint survival–longitudinal modelling approach for the dynamic prediction of re-hospitalization in telemonitored chronic heart failure patients. Statistical Modelling,13, 179–198.
Nooraee, N., Molenberghs, G. and van den Heuvel, E. (2014). GEE for longitudinal or-
dinal data: comparing R–replr, R–ordgee, SAS–GENMOD, SPSS–GENLIN. Com-putational Statistics & Data Analysis.
Oba, K., Paoletti, X., Alberts, S., Bang, Y.-J., Benedetti, J., Bleiberg, H., Catalano,.
Lordick, F., Michiels, S., Morita, S., Ohashi, Y., Pignon, J.-P., Rougier, P., Sasako,M., Sakamoto, J., Sargent, D., Shitara, K., Van Cutsem, E., Buyse, M. and Burzykowski,T. (2013). Disease-Free Survival as a Surrogate for Overall Survival in AdjuvantTrials of Gastric Cancer: A Meta-Analysis. JNCI-Journal of the National CancerInstitute, 105(21), 1600–1607.
Oba, K., Paoletti, X., Bang, Y-J., Bleiberg, H., Burzykowski, T., Fuse, N., Michiels,
S., Morita, S., Ohashi, Y., Pignon, J-P., Rougier, P., Pompidou, G., Sakamoto, J.,Sargent, D., Sasako, M., Shitara, K., Tsuburaya, A., Van Cutsem, E. and Buyse, M.
(2013). Role of chemotherapy for advanced/recurrent gastric cancer: An individual-patient-data meta-analysis. European Journal of Cancer, 49(7), 1565–1577.
Ogunjimi, B., Hens, N., Malfait, R., Van Tendeloo, V. and Smits, E. (2013). Creat-
ing a robust framework for the analysis of cryopreserved samples in quantitativeimmunological experiments. Journal of Immunological Methods, 392(1-2), 63–67.
Ogunjimi, B., Smits, E., Heynderickx, S., Van den Bergh, J., Bilcke, J., Jansens, H.,
Malfait, R., Ramet, J., Maecker, H.T., Cools, N., Beutels, P. and Van Damme, P.
(2014). Influence of Frequent Infectious Exposures on General and Varicella–Zoster
Virus–Specific Immune Responses in Pediatricians. Clinical and Vaccine Immunol-ogy, 21(3), 417–426.
Ogunjimi, B., Theeten, H., Hens, N. and Beutels, P. (2014). Serology indicates cy-
tomegalovirus infection is associated with varicella–zoster virus reactivation. Journalof Medical Virology, 86(5), 812–819.
Ogunjimi, B., Van Damme, P. and Beutels, P. (2013). Herpes Zoster Risk Reduction
through Exposure to Chickenpox Patients: A Systematic Multidisciplinary Review.
PLoS ONE, 8, 6.
Omelka, M., Veraverbeke, N. and Gijbels, I. (2013). Bootstrapping the conditional cop-
ula. Journal of Statistical Planning and Inference, 143(1), 1–23.
Paoletti, X., Oba, K., Bang, Y-J., Bleiberg, H., Boku, N., Bouche, O., Fuse, N., Michiels,
S., Moehler, M., Morita, S., Ohashi, Y., Ohtsu, A., Roth, A., Rougier, P., Sakamoto,J., Sargent, D., Sasako, M., Shitara, K., Thuss-Patience, P., Van Cutsem, E.,Burzykowski, T. and Buyse, M. (2013). Progression-Free Survival as a Surrogate forOverall Survival in Advanced/Recurrent Gastric Cancer Trials: A Meta-Analysis.
JNCI-Journal of the National Cancer Institute, 105(21), 1667–1670.
Pennemans, V., Rigo, J-M., Faes, C., Reynders, C., Penders, J. and Swennen, Q. (2013).
Establishment of reference values for novel urinary biomarkers for renal damagein the healthy population: are age and gender an issue? Clinical Chemistry andLaboratory Medicine, 51(9), 1795–1802.
Penninckx, F., Kartheuser, A., Van de Stadt, J., Pattyn, P., Mansvelt, B., Bertrand, C.,
Van Eycken, E., Jegou, D. and Fieuws, S. (2013). Outcome following laparoscopicand open total mesorectal excision for rectal cancer. British Journal of Surgery,100(10), 1368–1375.
Poleto, F., Paulino, C., Singer, J. and Molenberghs, G. (2014). Semi–parametric Bayesian
analysis of binary responses with a continuous covariate subject to non–randommissingness. Statistical Modelling.
Poletti, P., Melegaro, A., Ajelli, M., del Fava, E., Guzzetta, G., Faustini, L., Tomba,
G.S., Lopalco, P., Rizzo, C., Merler, S. and Manfredi, P. (2013). Perspectives onthe Impact of Varicella Immunization on Herpes Zoster. A Model-Based Evaluationfrom Three European Countries. PLoS ONE, 8, 4.
Potter, G.E., and Hens, N. (2013). A penalized likelihood approach to estimate within-
household contact networks from egocentric data. Journal of the Royal StatisticalSociety Series C-Applied Statistics, 62(4), 629–648.
Remy, S., Govarts, E., Bruckers, L., Paulussen, M., Wens, B., Den Hond, E., Nelen,
V., Baeyens, W., van Larebeke, N., Loots, I., Sioen, I. and Schoeters, G. (2014).
Expression of the sFLT1 Gene in Cord Blood Cells Is Associated to Maternal ArsenicExposure and Decreased Birth Weight. PLoS ONE, 9(3).
Rosselle, L., Permentier, L., Verbeke, G., Driessen, B. and Geers, R. (2013). Interac-
tions between climatological variables and sheltering behavior of pastoral beef cattleduring sunny weather in a temperate climate. Journal of Animal Science, 91(2),943–949.
Sinnaeve, P., Armstrong, P., Gershlick, A., Goldstein, P., Wilcox, R., Danays, T., Soulat,
L., Vandenberghe, K., Regelin, A., Bogaerts, K. and Van de Werf, F. (2013). OneYear Mortality in STEMI Patients Randomized to Primary PCI or a Pharmaco-invasive Strategy. The Stream 1 Year Follow-up. Circulation, 128(24), 2709–2709.
Solmi, F., Onghena, P., Salmaso, L. and Bult, I. (2014). A permutation solution to test
for treatment effects in alternation design single–case experiments. Communicationsin Statistics–Simulation and Computation, 43(5), 1094–1111.
Solmi, F., Onghena, P., Salmaso, L. and Bult, I. (2014). Extensions of permutation
solutions to test for treatment effects in replicated single–case alternation exper-iments with multivariate response. Communications in Statistics–Simulation andComputation, 43(5), 1036–1051.
Song, T., Dong, Q., Sankoh, A. and Molenberghs, G. (2013) Evaluation of overall treat-
ment effect in MMRM. Journal of Biopharmaceutical Statistics, 23(6), 1281–1293.
Stevens, A., Hansen, D., Vandoren, V., Westerlaken, R., Creemers, A., Eijnde, B.O. and
Dendale, P. (2014). Mandatory oral glucose tolerance tests identify more diabet-ics in stable patients with chronic heart failure: a prospective observational study.
Diabetology and Metabolic Syndrome, 6, 44.
Teshome, B., Lipkovich, I., Molenberghs, G. and Mallinckrodt, C. (2014). A multiple
imputation based approach to sensitivity analyses and effectiveness assessments inlongitudinal clinical trials. Journal of Biopharmaceutical Statistics, 24, 211–228.
Thevissen, P.W., Fieuws, S. and Willems, G. (2013). Third Molar Development: Eval-
uation of Nine Tooth Development Registration Techniques for Age Estimations.
Journal of Forensic Sciences, 58(2), 393–397.
Thilakarathne, P., Verbeke, G., Engelen, K., Marchal, K. and Lin, D. (2013). Identifying
Differentially Expressed genes in the Absence of Replication. International Journalof Bioinformatics Research and Applications, 9, 71–90.
Tomassetti, C., Geysenbergh, B., Meuleman, C., Timmerman, D., Fieuws, S., and
D'Hooghe, T., (2013). External validation of the endometriosis fertility index (EFI)staging system for predicting non-ART pregnancy after endometriosis surgery. Hu-man Reproduction, 28(5), 1280–1288.
Tomsin, K., Mesens, T., Molenberghs, G., Peeters, L. and Gyselaers, W. (2013). Char-
acteristics of heart, arteries, and veins in low and high cardiac output preeclampsia.
European Journal of Obstetrics & Genecology and Reproductive Biology, 169, 218–222.
Tomsin, K., Oben, J., Staelens, A., Mesens, T., Molenberghs, G., Peeters, L. and Gyse-
laers, W. (2013). Cardiovascular hemodynamics throughout normal pregnancy andpostpartum as measured by impedance cardiography. Acta Obstetricia et Gyneco-logica Scandinavica.
Tremmery, B., Danckaerts, M., Bruckers, L., Molenberghs, G., De Hert, M., Wampers,
M., De Var´e, J. and de Decker, A. (2014). Registration of aggressive incidents in anadolescent forensic psychiatric unit and implications for further practice. EuropeanChild & Adolescent Psychiatry.
Uranga, R. and Molenberghs, G. (2014). Conditional models with intermittent missing-
ness: SAS code and application. Journal of Statistical Computation and Simulation,84, 4, 753–780.
Van Beirendonck, S., Van Thielen, J., Verbeke, G. and Driessen, B. (2014). The asso-
ciation between sow and piglet behavior. Journal of Veterinary Behavior: ClinicalApplications and Research, 9(3), 107–113.
Van der Borght, K., Verbeke, G. and van Vlijmen, H. (2014). Multi–model inference using
mixed effects from a linear regression based genetic algorithm. BMC Bioinformatics,15.
Van der Elst, W., Molenberghs, G., Van Boxtel, M. and Jolles, J. (2013). Establishing
normative data for repeated cognitive assessment: a comparison of different statis-tical methods. Behavior Research Methods, 45(4), 1073–1086.
Van Duffel, D., Van Gemert, R., Starinieri, P., Pauwels, J-L., Natukunda, A., Rakhmawati,
T.W., Chirehwa, M.T., Orwa, J., Thys, H., Deboosere, P., Robic, B., Mees, U. andHendrikx, M. (2013). Elective reconstruction of the ascending aorta for aneurysmaldisease restores normal life expectancy. An analysis of risk factors for early and latemortality. Acta Cardiologica, 68(4), 349–353.
Van Kerckhove, K., Hens, N., Edmunds, W., J. and Eames, K.T., D. (2013). The Impact
of Illness on Social Networks: Implications for Transmission and Control of Influenza.
American Journal of Epidemiology, 178(11), 1655–1662.
Vannieuwenhuyze, J., Loosveldt, G. and Molenberghs, G. (2014). Evaluating Mode Ef-
fects in Mixed–Mode Survey Data Using Covariate Adjustment Models. Journal ofOfficial Statistics, 30(1), 1–21.
Veraverbeke, N., Gijbels, I. and Omelka, M. (2014). Preadjusted non–parametric estima-
tion of a conditional distribution function. Journal of the Royal Statistical SocietySeries B–Statistical Methodology, 76(2), 399–438.
Verbeke, G., Drikvandi, R., Khodadadi, A. and Partovi Nia, V. (2013). Testing multiple
variance components in linear mixed effects models. Biostatistics, 14, 144–159.
Verbeke, G., Fieuws, S., Molenberghs, G. and Davidian, M. (2014). The analysis of
multivariate longitudinal data: A review. Statistical methods in medical research,23(1), 42–59.
Verbeke, G. and Molenberghs, G., (2013). The gradient function as an exploratory
goodness-of-fit assessment of the random-effects distribution in mixed models. Bio-statistics, 14(3), 477–490.
Vandendijck, Y., Faes, C. and Hens, N. (2013). Eight Years of the Great Influenza Survey
to Monitor Influenza-Like Illness in Flanders. PLoS ONE, 8(5), 1–8.
Vandewalle, C., S., Baeten, M., Bogaerts, K., Vandekerckhove, P. and Compernolle, V.
(2013). Evaluation of 6 Years of Confidential Unit Exclusion at the Belgian RedCross Flanders: Waste or Value? Vox Sanguinis, 105, 165–165.
Verhasselt, A. (2014). Generalized varying coefficient models: a smooth variable selection
technique. Statistica sinica, 24, 147–171.
Vinken, M., Maes, M., Cavill, R., Valkenborg, D., Ellis, JK., Decrock, E., Leybaert, L.,
Staes, A., Gevaert, K., Oliveira, A.G., Menezes, G.B., Cogliati, B., Zaidan Dagli,M.L., Ebbels, T.M., D., Witters, E., Keun, H.C., Vanhaecke, T. and Rogiers, V.
(2013). Proteomic and metabolomic responses to connexin43 silencing in primaryhepatocyte cultures. Archives of Toxicology, 87(5), 883–894.
Vlayen, A., Hellings, J., Schrooten, W., Garcia Barrado, L., Haelterman, M. and Pele-
man, H. (2014). Evolution of Patient Safety Culture in Belgian Hospitals AfterImplementing a National Patient Safety Plan. BMJ Quality & Safety, 23(4), 346–347.
Vrijens, J., Leermakers, M., Stalpaert, M., Schoeters, G., Den Hond, E., Bruckers, L.,
Colles A., Nelen V., Van den Mierop E., Van Larebeke N., Loots I. and Baeyens W.
(2014). Trace metal concentrations measured in blood and urine of adolescents inFlanders, Belgium: Reference population and case studies Genk–Zuid and Menen.
International Journal of Hygiene and Environmental Health, 217(4-5), 515–527.
Wami, W., Buntinx, F., Bartholomeeusen, S., Goderis, G., Mathieu, C. and Aerts, M.
(2013). Influence of chronic comorbidity and medication on the efficacy of treatmentin patients with diabetes in general practice. British Journal of General Practice,63, 609, E267–E273.
Wellens, N., Verbeke, G., Flamaing, J., Moons, P., Boonen, S. Tournoy, J. and Milisen,
K. (2013). Clinical Changes in Older Adults During Hospitalization: Responsivenessof the interRAI Acute Care Instrument. Journal of the American Geriatrics Society,61(5), 799–804.
Wellens, N., Flamaing, J., Tournoy, J., Hanon, T., Moons, P., Verbeke, G., Boonen, S.
and Milisen K. (2013). Convergent validity of the Cognitive Performance Scale of theinterRAI Acute Care and the Mini–Mental State Examination. American Journalof Geriatric Psychiatry, 21, 636–645.
Willem, L., Stijven, S., Vladislavleva, E., Broeckhove, J., Beutels, P. and Hens, N. (2014)
Active learning to understand infectious disease models and improve policy making.
PLoS Computational Biology, 10, 4.
Zamanzad Ghavidel, F., Claesen, J., Burzykowski, T. and Valkenborg, D. (2014). Com-
parison of the Mahalanobis Distance and Pearsons chi(2) Statistic as Measures ofSimilarity of Isotope Patterns. Journal of the American Society For Mass Spectrom-etry, 25(2), 293–296.
Zamanzad Ghavidel, F., Mertens, I., Baggerman, G., Laukens, K., Burzykowski, T. and
Valkenborg, D. (2014). The use of the isotopic distribution as a complementaryquality metric to assess tandem mass spectra results. Journal of Proteomics, 98,150–158.
Zhu, Q. and Burzykowski, T. (2013). A Markov-chain-based regression model with ran-
dom effects for the analysis of 18O-labelled mass spectra. Journal of StatisticalComputation and Simulation, 83(1), 145–157.
Refereed publications (in press, accepted for publication).
Abrams, S., Hens, N. (2014). Correlated Frailty Models for Non-immunizing Infections,
Biostatistics, to appear.
Bruyndonckx, R., Hens, N., Aerts, M., Goossens, H., Molenberghs, G. and Coenen S.
(2014). Measuring trends of outpatient antibiotic use in Europe: jointly modellinglongitudinal data in defined daily doses and packages. The Journal of AntimicrobialChemotherapy, to appear.
Costa, R., Bergwerg, I., Santermans, H., De Vocht, N., Praet, J., Daans, J., Blon, D.L.,
Hoornaert, C., Reeksmans, K., Hens, N., Goossens, H., Berneman, Z., Parolini, O.,Alviano, F. and Ponsaerts, P. (2014). Distinct in vitro properties of embryonic andextra-embryonic fibroblast-like cells are reflected in their in vivo behaviour followinggrafting in the adult mouse brain, Cell Transplantation, to appear.
Ensoy, C., Faes, C., Welby, S., Van der Stede, Y., Aerts, M. (2014). Exploring cattle
movements in Belgium. Preventive Veterinary Medicine, to appear.
Efendi, A. and Molenberghs, G. (2013). A multilevel model for hierarchical, repeated,
and overdispersed time-to-event outcomes and its estimation strategies. Journal ofBiopharmaceutical Statistics, to appear.
Fieuws, S., Molenberghs, G. and Davidian, M. (2014). The analysis of multivariate
longitudinal data: A review. Statistical Methods in Medical Research, 21, to appear.
Ivanova, A., Molenberghs, G. and Verbeke, G. (2013). A model for overdispersed hierar-
chical ordinal data. Statistical Modelling, to appear.
Kassahun, W., Neyens, T., Molenberghs, G., Faes, C., Verbeke, G. (2014). A joint model
for hierarchical continuous and zero-inflated overdispersed count data. Journal ofStatistical Computation and Simulation, to appear.
Le Blon, D., Hoornaert, C., Daans, J., Santermans, E., Hens, N., Goossens, H., Berne-
man, Z. and Ponsaerts, P. (2014). Distinct spatial distribution of microglia andmacrophages following mesenchymal stem cell implantation in mouse brain. Im-munology and Cell Biology, to appear.
Loeys, T. and Molenberghs, G. (2013). The analysis of (negatively) correlated non-
Gaussian dyadic outcomes: non-multilevel-based alternatives. Psychological meth-ods, to appear.
Mallinckrodt, C., Roger, J., Chuang-Stein, C., Molenberghs, G., Lane, P.W., O'Kelly,
M., Ratitch, B., Lu, X., Gilbert, S., Mehrotra, D., Wolfinger, R., and Thijs, H.
(2014). Missing data: Turning guidance into action. Statistics in BiopharmaceuticalResearch, to appear.
Milanzi, E., Molenberghs, G., Alonso, A., Kenward, M., Tsiatis, A., Davidian, M. and
Verbeke, G. (2014). Estimation after a group sequential trial. Statistics in Bio-Sciences.
Milanzi, E., Molenberghs, G., Alonso, A., Verbeke G. and De Boeck, P. (2014). Reliabil-
ity measures in item response theory: Manifest versus latent correlation functions.
British Journal of Mathematical and Statistical Psychology, to appear.
Molenberghs, G., Verbeke, G., Efendi, A., Braekers, R. and Dem´etrio, C. (2014). A com-
bined gamma frailty and normal random–effects model for repeated, overdispersedtime–to–event data. Statistical Methods in Medical Research, to appear. (KUL-1and UH)
Oben, J., Tomsin, K., Mesens, T., Staelens, A., Molenberghs, G. and Gyselaers, W.
(2014). Maternal cardiovascular profiling in the first trimester of pregnancies com-plicated with gestation–induced hypertension or fetal growth retardation: a pilotstudy. The journal of maternal–fetal & neonatal medicine: the official journal of theEuropean Association of Perinatal Medicine, the Federation of Asia and OceaniaPerinatal Societies, the International Society of Perinatal Obstetricians, to appear.
Reynders, A., Kerkhof, A., Molenberghs, G., Van Audenhove, C. (2014). Attitudes and
stigma in relation to help–seeking intentions for psychological problems in low andhigh suicide rate regions. Social Psychiatry and Psychiatric Epidemiology, to appear.
Tremmery, S., Danckaerts, M., Bruckers, L., Molenberghs, G., De Hert, M., Wampers,
M., De Var´e, J., and de Decker, A. (2014). Registration of aggressive incidents inan adolescent forensic psychiatric unit and implications for further practice. Eur.
Child Adolesc Psychiatry, to appear.
Vlayen, A., Schrooten, W., Wami W.M., Aerts, M., Claes, N., Barrado, L. and G.,
Hellings, J. (2014). Variability of patient safety culture in Belgian acute hospitals.
Journal of Patient Safety, to appear.
Books & Book Chapters (published).
Alonso Abad, A., Molenberghs, G. and Van Breukelen, G. (2014). Statistical validation
of surrogate markers in clinical trials. In Developments in Statistical Evaluation ofClinical Trials, van Montfort, Kees; Oud, Johan; Ghidey, Wendimagegn (Eds.).
Amaratunga, D., Cabrera, J. and Shkedy, Z. (2014). Exploration and Analysis of DNA
Microarray and Other High-Dimensional Data. Wiley Series in Probability andStatistics (2nd edition). New York: Wiley.
Ejigu, B.A. (2013). Marginal and Random effects models in Vaccine Clinical Trials: Sta-
tistical Modeling of Solicited Symptoms in Vaccine Clinical Trials. LAP LAMBERTAcademic Publishing.
Galecki, A. and Burzykowski, T. (2013). Linear Mixed Effects Models Using R. A Step-
by-Step Approach: Springer Texts in Statistics. New York.
Molenberghs, G. and Lesaffre, E. (2013). Missing Data. In Wiley Encyclopedia of Clinical
Trials, DAgostino, R. Massaro, J. and Sullivan, L. (Eds.).
Theeten, H., Lefevere, E., Vandermeulen, C., Van Damme, P. and Hens, N. (2013). Fac-
tors in- fluencing infant and adolescent vaccine uptake in Flanders, Belgium. InModeling the Interplay Between Human Behavior and the Spread of Infectious Dis-eases, Manfredi, Piero, Donofrio, Alberto (Eds.), pp. 43–55.
e libre de Bruxelles, ULB
Refereed publications (published).
D'Agostino, A., Gambetti, L. and Giannone, D. (2013). Macroeconomic Forecasting and
Structural Change. Journal of Applied Econometrics, 28, 81–101.
Ampe, E., Hestir, E., Bresciani, M., Salvadore, E., Brando, V., Dekker, A., Malthus, T.,
Jansen, M., Triest, L. and Batelaan., O. (2014). A wavelet approach for estimatingchlorophyll-a from inland waters. IEEE Geoscience and Remote Sensing Letters,11(1), 89–93.
ormann, S. and Kokoszka, P. (2013). Monitoring the intraday volatility
pattern. Journal of Time Series Econometrics, 5(2), 87–116.
Hallin, M. (2013). Discussion of "Large covariance estimation by thresholding principal
orthogonal complements", by J. Fan, Y. Liao, and M. Mincheva. Journal of theRoyal Statistical Society Series B, 75, 647–648.
Hallin, M. and Lippi, M. (2013). Factor models in high-dimensional time series: a time-
domain approach. Stochastic Processes and their Applications, 123(7), 2678–2695.
Hallin, M. and Lu, Z. (2013). Discussion of "Local quantile regression", by V. Spokoiny,
W. Wang, and W. H¨
ardle. Journal of Statistical Planning and Inference, 143, 1130–
Hallin, M., Paindaveine, D. and Verdebout, Th. (2013). Optimal rank-based tests for
common principal components. Bernoulli, 19, 2524–2556.
Jansen, M. (2014). Information criteria for variable selection under sparsity. Biometrika,
101(1), 37–55.
Lecharlier, L. and De Mol, C. (2013). Regularized blind deconvolution with Poisson data.
Journal of Physics: Conference Series, 464, 012003.
Onatski, A., Moreira, M. and Hallin, M. (2013). Asymptotic power of sphericity tests
for high-dimensional data. Annals of Statistics, 41, 1204–1231.
Onatski, A., Moreira, M. and Hallin, M. (2014). Signal detection in high dimension: the
multispiked case. Annals of Statistics, 42, 225–254.
Paindaveine, D. and Van Bever, G. (2013). From depth to local depth : a focus on
centrality. Journal of the American Statistical Association 105, 1105–1119.
Paindaveine, D. and Van Bever, G. (2014). Inference on the shape of elliptical distribu-
tions based on the MCD. Journal of Multivariate Analysis 129, 125–144.
Refereed publications (in press, accepted for publication).
Aue, A., Dubart Norinho, D. and H¨
ormann, S. (2014). On the prediction of functional
time series. Journal of the American Statistical Association, to appear.
ormann, S., Horv´
ath, L. and Huˇskov´
a, M. (2014). Dependent functional
linear models with applications to monitoring structural change. Statistica Sinica,to appear.
Bada, O. and Liebl, D. (2014). The R-package phtt: panel data analysis with heteroge-
neous time trends. Journal of Statistical Software, to appear.
Dehon, C., Desbordes, R. and Verardi, V. (2014). The pitfalls of ignoring outliers in
instrumental variables estimations: an application to the deep determinants of de-velopment. Advanced Studies in Theoretical and Applied Econometrics, to appear.
Dette, H., Hallin, M., Kley, T. and Volgushev, S. (2014). Of quantiles, ranks, and spectra:
an L1 approach to spectral analysis. Bernoulli, to appear.
Forni, M., Hallin, M., Lippi, M. and Zaffaroni, P. (2014). Dynamic factor models with
infinite-dimensional factor space: one-sided representations. Journal of Economet-rics, to appear.
Giannone, D., Lenza, M., Momferatou, D. and Onorante, L. (2014). Short-term inflation
projections: a bayesian vector autoregressive approach. International Journal ofForecasting, to appear.
Giannone, D., Lenza, M. and Primiceri, G. (2014). Prior selection for vector autoregres-
sions. Review of Economics and Statistics, to appear.
Hallin, M. and Ley, Chr. (2014). Skew-symmetric distributions and Fisher information:
the double sin of the skew-normal. Bernoulli, to appear.
Hallin, M., Lu, Z., Paindaveine, D. and ˇ
Siman, M. (2014). Local bilinear multiple-output
quantile regression. Bernoulli, to appear.
Hallin, M. and Mehta, Ch. (2014). R-estimation for asymmetric Independent Component
Analysis. Journal of the American Statistical Association, to appear.
Hallin, M., Paindaveine, D. and Verdebout, Th. (2014). Efficient R-estimation of princi-
pal and c ommon principal components. Journal of the American Statistical Asso-ciation, to appear.
H¨ormann, S. and Kidzi´
nski, L. (2014). A note on estimation in Hilbertian linear models.
Scandinavian Journal of Statistics, to appear.
ormann, S., Kidzi´
nski, L. and Hallin, M. (2014). Dynamic functional principal compo-
nents. Journal of the Royal Statistical Society: Series B, to appear.
Liebl, D., Willwacher, S., Hamill, J. and Br¨
uggemann, G.-P. (2014). Ankle plantarflexion
strength in rearfoot and forefoot runners: a novel clusteranalytic approach. HumanMovement Science, to appear.
Paindaveine, D. and Van Bever, G. (2014). Nonparametrically consistent depth-based
classifiers. Bernoulli, to appear.
Peeters, C., Dehon, C. and Garcia-Prieto, P. (2014). The attention stimulus of cultural
differences in global services sourcing. Journal of International Business Studies, toappear.
Books & Book Chapters (published).
Banbura, M., Giannone, D., Modugno, M. and Reichlin, L. (2013). Now-casting and the
real-time data-flow. In Handbook of Economic Forecasting, Volume 2, Part A, G.
Elliot and A. Timmermann (Eds.), pp. 195–237. North-Holland, Elsevier.
Hallin, M., Swan, Y. and Verdebout, Th. (2013). On Hodges and Lehmann's "6/π
result". In Contemporary Developments in Statistical Theory, a Festschrift for HiraLal Koul, S.N. Lahiri, A. Schick, A. Sengupta, and T.N. Sriram (Eds), pp. 137–153.
Springer.
Jansen, M. (2013). Noise reduction in an adaptive multiscale local polynomial decompo-
sition. In Proceedings of the 21st European Signal Processing Conference (Eusipco).
Jansen, M. (2014). Nonseparable Laplacian pyramids with multiscale local polynomials
for scattered data. In Proceedings IWSSIP, 21st International Conference on Sys-tems, Signals and Image Processing, M. Mu˘stra, D. Trali´c, M. Grgi´c and B. Zovko-Cihlar (Eds.), pp.115-118.
Books & Book Chapters (in press, accepted for publication).
Claeskens, G. and Jansen, M. (2014). Model selection and model averaging. In Interna-
tional Encyclopedia of Social and Behavioral Sciences (2nd Ed.), J. Wright (Ed.).
Hallin, M., van den Akker, R. and Werker, B. (2014). On quadratic expansions of log-
likelihoods and a general asymptotic linearity result. In Mathematical Statistics andLimit Theorems: Festschrift in Honor of Paul Deheuvels, M. Hallin, D. Mason, D.
Pfeifer and J. Steinebach (Eds.). Springer.
Jansen, M. (2014). Multiscale local polynomial models for estimation and testing. In
Topics in NonParametric Statistics, Proceedings of the First Conference of the In-ternational Society for Nonparametric Statistics, M. Akritas, S. N. Lahiri and D.
Politis (Eds.).
Paindaveine, D. and Verdebout, Th. (2014). Optimal rank-based tests for the location
parameter of a rotationally symmetric distribution on the hypersphere. In Mathe-matical Statistics and Limit Theorems: Festschrift in Honor of Paul Deheuvels, M.
Hallin, D. Mason, D. Pfeifer and J. Steinebach (Eds.). Springer.
Refereed publications (published).
Jaeger, J. and Lambert, P. (2013). Bayesian P-spline estimation in hierarchical models
specified by systems of affine differential equations. Statistical Modelling, 13, 3–40.
Lambert, P. (2013). Nonparametric additive location-scale models for interval censored
data. Statistics and Computing, 23, 75–90.
Lebrun, P., Boulanger, B., Debrus, B. and Lambert, P. (2013). A Bayesian design space
for analytical methods based on multivariate models and predictions. Journal ofBiopharmaceutical Statistics, 23, 1330–1351.
Magis, D. (2013). A note on the item information function of the four-parameter logistic
model. Applied Psychological Measurement, 37, 304–315.
Magis, D. (2014a). Accuracy of asymptotic standard errors of the maximum and weighted
likelihood estimators of proficiency levels with short tests. Applied PsychologicalMeasurement, 38, 105–121.
Magis, D. and Facon, B. (2013). Item purification does not always improve DIF detec-
tion: a counter-example with Angoff's Delta plot. Educational and PsychologicalMeasurement, 73, 293–311.
Raiche, G., Walls, T. A., Magis, D., Riopel, M. and Blais, J.-G. (2013). Non-graphical
solutions for Cattells scree test. Methodology: European Journal of Research Methodsfor the Behavioral and Social Sciences, 9, 23–29.
Wang, L., Kai, B., Heuchenne, C. and Tsai, C.-L. (2013). Penalized possibly nonsmooth
profiling semiparametric estimating equations. Electronic Journal of Statistics, 7,2656–2682.
Refereed publications (in press, accepted for publication).
Bremhorst, V. and Lambert, P. (2014). Flexible estimation in cure survival models using
Bayesian P-splines. Computational Statistics and Data Analysis, to appear.
Celano, G., Faraz, A., Saniga, E. (2013). Control charts monitoring product's loss to
society. Quality and Reliability Engineering International, to appear.
Colling, B., Heuchenne, C., Samb, R. and Van Keilegom, I. (2013). Estimation of the
error density in a semiparametric transformation model. Annals of the Institute ofStatistical Mathematics, to appear.
Donneau, A.F., Mauer M., Lambert, P., Molenberghs, G. and Albert, A. (2014). Simulation-
based study comparing multiple imputation methods for non-monotone missing ordi-nal data in longitudinal settings. Journal of Biopharmaceutical Statistics, to appear.
Faraz, A., Celano, G., Heuchenne, C., Saniga, E. and Fichera, S. (2013). The variable
parameters T2 chart with run rules. Statistical Papers, to appear.
Frasso, G. and Eilers P.H.C. (2014). L- and V-curves for optimal smoothing. Statistical
Modelling, to appear.
Jaeger, J. and Lambert, P. (2014). Bayesian penalized smoothing approaches in models
specified using affine differential equations with unknown error distributions. Journalof Applied Statistics, to appear.
Lambert, P. (2014). Spline approximation to conditional Archimedean copula. Stat
(Wiley), to appear.
Magis, D. (2014b). A note on weighted likelihood and Jeffreys modal estimation of
proficiency levels in polytomous item response models. Psychometrika, to appear.
Magis, D. (2014c). On the asymptotic standard error of a class of robust estimators of
ability in dichotomous item response models. British Journal of Mathematical andStatistical Psychology, to appear.
Magis, D. and De Boeck, P. (2014). Type I error inflation in DIF identification with
Mantel-Haenszel: an explanation and a solution. Educational and PsychologicalMeasurement, to appear.
Nourdin, I., Peccati, G. and Swan, Y. (2014). Integration by parts and representation of
information functionals. Proceedings of the 2014 IEEE International Symposium onInformation Theory, to appear.
Seif, A., Faraz, A., Sadeghifar, M. (2014a). Evaluation of the Economic Statistical De-
sign of the Multivariate T2 Control Chart with Multiple Variable Sampling IntervalsScheme: NSGA-II Approach. Journal of Statistical Computation and Simulation, toappear.
Charles University at Prague, CU
Refereed publications (published).
Antoch, J. and Jaruˇskov´
a, D. (2013). Testing for multiple change points. Computational
Statistics, 28, 2161–2183.
Antoch, J., Prchal, L. and Sarda, P. (2013). Combining association measures for lexical
classification using clustering of receiver operating characteristic curves. Journal ofClassification, 30, 100–123.
Aue, A., Hormann, S., Horv´
ath, L and Huˇskov´
a, M. (2014). Dependent functional linear
models with applications to monitoring structural change. Statistica Sinica, 24(3)+ plus supplement 12 pp.
Gijbels, I. and Omelka, M. (2013). Testing for homogeneity of multivariate dispersions
using dissimilarity measures. Biometrics, 69, 137–145.
ath, L. and Huˇskov´
a, M. (2014). Change point detection in panel data. In: Con-
tributions in infinite-dimensional statistics and related topics, Eds. E.G.Bongiorno,E.Salinelli, A.Goia, P.Vieu, published by Societ Editrice Esculapio, 155–160.
Omelka, M. and Hudecov´
S. (2013). A comparison of the Mantel test with a generalized
distance covariance test. Environmetrics, 24, 449–460.
Omelka, M., Veraverbeke, N. and Gijbels, I. (2013). Bootstrapping the conditional cop-
ula. Journal of Statistical Planning and Inference, 143, 1–23.
Veraverbeke, N., Gijbels, I. and Omelka, M. (2014). Pre-adjusted nonparametric estima-
tion of a conditional distribution function. Journal of the Royal Statistical Society:Series B, 76, 399–438.
Refereed publications (in press, accepted for publication).
arek, A., Kom´arkov´
a, L. (2014). Capabilities of R package mixAK for clustering
based on multivariate continuous and discrete longitudinal data. Journal of Statis-tical Software, to appear.
Nagy, S. (2014). On the consistency of depth functionals (2014). Proceedings IWFOS
(Contributions in infinite-dimensional statistics and related topics), to appear.
ak, M., Antoch, J., Kula, J. and Chvojka, J. (2013). Estimation of fiber system
orientation for nonwoven and nanofibrous layers: Local approach based on imageanalysis. Textile Research Journal, to appear.
Rijkskuniversiteit Groningen, RUG
Refereed publications (published).
Albers, C.J. and Gower, J.C. (2014). Canonical analysis: ranks, ratios and fits. Journal
of Classification, 31(1), 2–27.
Anacleto, O. and Queen, C.M. and Albers, C.J. (2013). Forecasting multivariate road
traffic flows using Bayesian dynamic graphical models, splines and other traffic vari-ables. Australian and New Zealand Journal of Statistics, 55(2), 69–86.
Anacleto, O. and Queen, C.M. and Albers, C.J. (2013). Multivariate forecasting of road
traffic flows in the presence of heteroscedasticity and measurement errors. Journalof the Royal Statistical Society, Series C: Applied Statistics, 62(2), 251–270.
Barendse, M. T., Oort, F. J., Jak, S. and Timmerman, M. E. (2013). Multilevel ex-
ploratory factor analysis of discrete data. Netherlands Journal of Psychology, 67(4),114–121.
Cserjesi, R., Van Braeckel, K. N. J. A., Timmerman, M., Butcher, P. R., Kerstjens, J.
M., Reijneveld, S. A., Bouma, A., Bos, A. F. and Geuze, R. H. (2013). Cserjesi etal. reply. Developmental Medicine & Child Neurology, 7, 674.
de Koning, B., Timmerman, M. E., van Geert, P. and van der Meulen, B. F. (2014).
Identifying learning trajectories while playing a learning-to-learn computer gamein different children and instruction types. Journal of Cognitive Education andPsychology, 13(1), 53–75.
De Roover, K., Timmerman, M. E., Van Mechelen, I. and Ceulemans, E. (2013). On
the added value of multiset methods for three-way data analysis. Chemometrics andIntelligent Laboratory Systems, 129, 98–107.
De Roover, K., Timmerman, M. E., Mesquita, B. and Ceulemans, E. (2013). Common
and Cluster-Specific Simultaneous Component Analysis. Plos One, 8(5), e62280.
Morey, R. D., Romeijn, J.-W. and Rouder, J. N. (2013). The humble Bayesian: model
checking from a fully Bayesian perspective. British Journal of Mathematical andStatistical Psychology, 66(1), 68–75.
de Vries, R. M. and Morey, R. D. (2013). Statistical Analysis of Single-Subject Data: A
Bayesian Model. Psychologial Methods.
Refereed publications (in press, accepted for publication).
Elliott, E. M., Morey C. C., Morey, R. D., Eaves, S. D. and Shelton, J. T. (2014).
Assessing Interference Effects Across Modalities: A Comparison of Three Versionsof Stroop Tasks. Journal of Cognitive Psychology, to appear.
Hoekstra, R., Morey, R. D., Rouder, J. N. Wagenmakers, E.-J. (2014). Robust mis-
interpretation of condence intervals among students andresearchers. PsychonomicBulletin & Review, to appear.
Huitsing, G., Snijders, T. A. B., Van Duijn, M. A.J., and Veenstra, R. (2014). Victims,
bullies, and their defenders: A longitudinal study of the co-evolution of positive andnegative networks. Development and Psychopathology, to appear.
Morey, C. C., Morey, R. D., and van der Reijden, M. (2014). Asymmetric cross-domain
interference between two working memory tasks: Implications for models of workingmemory. Journal of Memory and Language, to appear.
Morey, R. D. (2014). The consistency test does not and cannot deliver what is advertised:
A comment on Francis (2013). Journal of Mathematical Psychology, to appear.
Morey, R. D., Rouder, J. N., Verhagen, J., and Wagenmakers E.-J. (2014). Why Hy-
pothesis Tests Are Essential for Psychological Science: A Comment on Cumming(2014).Psychological Science, to appear.
Rouder, J. N., Morey, R. D., Gomez, P. I. and Heathcote, A. (2014). TheLognormal
Race: A Cognitive-Process Model of Choice and Latency with Desirable Psychome-tric Properties. Psychometrika, to appear.
Schuppert, H.M., Albers, C.J., Minderaa, R., Emmelkamp, P., Nauta, M. (2014). Sever-
ity of borderline personality symptoms in adolescence: relationship with maternalparenting stress, maternal psychopathology, and rearing styles. Journal of Person-ality Disorders, to appear.
Snijders, T.A.B and Christian, E.G. Steglich (2014). Representing Micro-Macro Link-
ages by Actor-Based Dynamic Network Models. Sociological Methods & Research,to appear.
Books & Book Chapters (published).
Wittek, R., Snijders, T.A.B. and Nee, V. (eds). (2013). The Handbook of Rational Choice
Social Research. Stanford, CA: Stanford University Press.
Universidad de Santiago de Compostela, USC
Refereed publications (published).
Boente, G., Cao, R., Gonz´
alez–Manteiga, W. and Rodr´ıguez, D. (2013). Testing in
generalized partially linear models: A Robust approach. Statistics & ProbabilityLetters, 83, 203–212.
Cuevas, A., Llop, P. and Pateiro–L´
opez, B. (2014). On the estimation of the medial axis
and inner parallel body. Journal of Multivariate Analysis, 129, 171–185.
Febrero–Bande, M. and Gonz´
alez–Manteiga, W. (2013). Generalized Additive Models
for Functional Data. Test, 22(2), 278–292.
Fuentes-Santos, I., Marey–P´erez, M.F. and Gonz´
alez–Manteiga, W. (2013). Forest fires
Spatial patterns analysis in Galicia (NW Spain). Journal of Environmental Man-agement, 15, 30–42.
Garc´ıa–Portugu´es, E., Crujeiras, R. M. and Gonz´
alez–Manteiga, W. (2013). Exploring
wind direction and SO2 concentration by circular-linear density estimation. Stochas-tic Environmental Research and Risk Assesment, 27, 1055–1067.
Garc´ıa–Portugu´es, E. (2013). Exact risk improvement of bandwidth selectors for kernel
density estimation with directional data. Electronic Journal of Statistics, 7, 1655–1685.
Garc´ıa–Portugu´es, E., Crujeiras, R.M. and Gonz´
alez–Manteiga, W. (2013). Kernel den-
sity estimation for directional-linear data. Journal of Multivariate Analysis, 121,152–175.
alez–Manteiga, W. and Crujeiras, R.M. (2013). An updated review of Goodness-of-
Fit tests for regression models. Test, 22, 361–447.
Lafarge, T., Pateiro–L´
opez, B., Possolo, A. and Dunkers, J.P. (2014). R Implementation
of a Polyhedral Approximation to a 3D Set of Points Using the alpha-Shape. Journalof Statistical Software, 56(4), 1–18, 2014.
Meira–Machado, L., Roca–Pardi˜
nas, J., Van Keilegom, I. and Cadarso–Su´arez, C.M.
(2013). Bandwidth selection for the estimation of transition probabilities in thelocation-scale progressive three-state model. Computational Statistics, 28, 2185–2210.
Oliveira, M., Crujeiras, R.M. and Rodr´ıguez–Casal, A. (2013). Nonparametric circular
methods for exploring environmental data. Journal of Environmental and EcologicalStatistics, 20, 1–17.
Oliveira, M., Crujeiras, R., Rodr´ıguez–Casal, A. (2014). CircSiZer: an exploratory tool
for circular data. Journal of Environmental and Ecological Statistics, 21, 143–159.
opez, B. and Rodr´ıguez–Casal, A. (2013). Recovering the shape of a point
cloud in the plane. TEST, 22, 19–45.
Reyes–Sant´ıas F., Cadarso–Su´
arez, C.M. and Mart´ınez–Calvo, A. (2013). Applying a
simulation model in order to manage waiting lists for hospital inpatient activity inan EU region. Mathematical and Computer Modelling, 57, 1840–1846.
Rodr´ıguez, S., Miguens, X., Rodr´ıguez–Calvo, M.S., Febrero-Bande, M., Muoz Bars, JI.
(20??). Estimating adult stature from radiographically determined metatarsal lengthin a Spanish population. Forensic Science International, 226(1-3), 297.e1–297.e4.
Rodr´ıguez–Girondo, M., Kneib, T., Cadarso–Su´
arez, C.M. and Abu–Assi, E. (2013).
Model Building in Non Proportional Hazard Regression. Statistics in Medicine, 32,5301–5314.
Ruiz–Medina, M.D., Crujeiras, R. (2014). A central limit result in the wavelet domain
for minimum contrast estimation of fractal random fields. Theory of Probability andits Applications, 58, 1–34.
Refereed publications (in press, accepted for publication).
Abalo–Lojo J.M., Carollo–Limeres, M.C., Arias–G´
omez, M., Baleato–Gonz´alez, S., Cadarso–
arez, C.M., Capeans, C., Gonz´
alez, F. (2014). Retinal nerve fibre layer thick-
ness, brain atrophy and disability in multiple sclerosis patients. Journal of Neuro–Ophthalmology, to appear.
Boente, G., Gonz´
alez–Manteiga, W. and Rodr´ıguez, D. (2014). Goodness-of-fit test for
directional data. Scandinavian Journal of Statistics, to appear.
Duarte, E., de Sousa, B., Cadarso–Su´
arez, C.M., Rodrigues, B. and Kneib, T. (2014).
Structured additive regression (STAR) modeling of age of menarche. BiometricalJournal, to appear.
Garc´ıa–Portugu´es, E., Gonz´
alez–Manteiga, W. and Febrero–Bande, M. (2013). A goodness-
of-fit test for the functional linear model with scalar response. Journal of Computa-tional and Graphical Statistics, to appear.
Garc´ıa–Portugu´es, E., Barros, A.M.G., Crujeiras, R.M., Gonz´alez–Manteiga, W. and
Pereira, J.M.C. (2014). A test for directional-linear independence, with applica-tions to wildfire orientation and size. Stochastic Environmental Research and RiskAssessment, to appear.
Gayoso–Diz P., Otero–Gonz´
alez A., Rodr´ıguez– ´
Alvarez, MX., Gude, F., Cadarso–Su´arez,
C.M., Garc´ıa F. and De Francisco, A. (2014). Insulin resistance index (HOMA-IR)levels in a general adult population: Curves percentile by gender and age. TheEPIRCE study. Diabetes Res Clin Pract, to appear.
Ojeda, J. L. , Gonz´
alez–Manteiga, W. and Crist´
obal–Crist´obal, J.A. (2014). Testing
regression models with selection–biased data. Annals of the Institute of StatisticalMathematics, to appear.
Pardo–Seco, J., Amigo, J., Gonz´
alez–Manteiga, W. and Salas A. (2014). A generalized
model to estimate the statistical power in mitochondrial disease studies involving2xk tables. PLoS ONE, to appear.
Rodr´ıguez, S., Gonz´
on, A., Rodr´ıguez–Calvo, M.S., Febrero–Bande, M.,
Cordeiro, C., Mu˜
us, J.I. (2014). The use of computerized tomography in
determining stature and sex from metatarsal bones. Legal Medicine, to appear.
Santana–Mora, U., L´
on, M., Mora, MJ., Cadarso–Su´arez, C.M., L´opez–Cedr´
J. and Santana–Pen´ın, U.A. (2014). Surface raw electromyography has a moderatediscriminatory capacity for differentiating between healthy individuals and thosewith TMD: A diagnostic study. Journal of Electromyography and Kinesiology, toappear.
Wiesenfahrt, M., Hisgen, C.M., Kneib, T., Cadarso–Su´
arez, C.M. (2014). Bayesian Non-
parametric Instrumental Variables Regression based on Penalized Splines and Dirich-let Process Mixtures. Journal of Business and Economic Statistics, to appear.
London School of Hygiene and Tropical Medicine, LSHTM
Refereed publications (published).
Aerts, C., Molenberghs, G., Kenward and M. G., Neiner, C. (2014). The surface nitrogen
abundance of a massive star in relation to its oscillations, rotation and magnetic field,Astrophysical journal, 781, 1.
Bamia, C., White, I.R. and Kenward, M.G. (2013). Some consequences of assuming
simple patterns for the treatment effect over time in a linear mixed model, Statisticsin Medicine, 32, 2585–2594.
Bell, M.L., Kenward, M.G., Fairclough, D.L. and Horton, N.J. (2013).
dropout and bias in randomised controlled trials: when it matters and when itmay not, BMJ-British Medical Journal, 346, e868.
Carpenter, J.R., Roger, J.H. and Kenward, M.G. (2013). Analysis of longitudinal tri-
als with protocol deviation: a framework for relevant, accessible assumptions, andinference via multiple imputation, Journal of Biopharmaceutical statistics, 23, 1352–1371.
Devries, K.M., Kenward, M.G. and Free, C.J. (2013). Preventing Smoking Relapse Using
Text Messages: Analysis of Data From the txt2stop Trial, Nicotine and TobaccoResearch, 15, 77–82.
Diaz-Ordaz, K., Kenward, M. G. and Grieve, R. (2014). Handling missing values in cost
effectiveness analyses that use data from cluster randomized trials, Journal of theRoyal Statistical Society, Series A, 177, 457–474
Keenan, K., Kenward, M.G., Grundy, E. and Leon, D.A. (2013). Longitudinal Prediction
of Divorce in Russia: The Role of Individual and Couple Drinking Patterns, Alcoholand Alcoholism, 48, 737–742.
Mallinckrodt, C., Lin, Q., Carpenter, J., Kenward, M. and Molenberghs G. (2013). A
structured framework for assessing sensitivity to missing data assumptions in longi-tudinal clinical trials. Pharmaceutical Statistics, 12(1), 1–6.
Molenberghs, G., Kenward, M., Aerts, M., Verbeke, G., Tsiatis, A., Davidian, M. and Ri-
zopoulos, D. (2014). On random sample size, ignorability, ancillarity, completeness,separability, and degeneracy: sequential trials, random sample size, and missingdata. Statistical Methods in Medical Research, 23, 11–41.
Molenberghs, G., Kenward, M. G., Verbeke, G., Iddi, S. and Efendi, A. (2013). On
the Connections Between Bridge Distributions, Marginalized Multilevel Models, andGeneralized Linear Mixed Models. International Journal of Statistics and Probabil-ity, 2(4), 1–21.
Ng, E.S.W, Grieve, R. and Carpenter, J.R. (2013). Two-stage nonparametric bootstrap
sampling with shrinkage correction for clustered data, STATA journal, 13, 141–164.
Pantazis, N., Kenward, M.G. and Touloumi, G. (2013. Performance of parametric sur-
vival models under non-random interval censoring: A simulation study, Computa-tional Statistics and Data Analysis, 63, 16–30
Refereed publications (in press, accepted for publication).
Kenward, M.G. and Molenberghs, G. (2014). A taxonomy of mixing and outcome distri-
butions based on conjugacy and bridging. Communications in Statistics – Theoryand Methods, to appear.
Njeru Njagi, E., Molenberghs, G., Rizopoulos, D., Verbeke, G., Kenward, M.G., Dendale,
P. and Willekens, K. (2014). A flexible joint-modelling framework for longitudinaland time-to-event data with overdispersion. Statistical Methods in Medical Research,to appear.
Njeru Njagi, E., Molenberghs, G., Kenward, M.G., Verbeke, G. and Rizopoulos, D.
(2014). A characterization of missingness at random in a generalized shared-parameterjoint modelling framework for longitudinal and time-to-event data, and sensitivityanalysis. Biometrical Journal, to appear.
Milanzi, E., Molenberghs, G., Alonso, A., Kenward, M.G., Tsiatis, A.A., Davidian, M.
and Verbeke, G. (2014). Estimation after a group sequential trial. Statistics in Bio-sciences, to appear.
List of co-publications
Here we list all scientific publications co-authored by IAP-members belonging to at leasttwo different research teams (partners). For each publication we indicate between bracketsthe contributing teams.
Refereed publications (published).
Aerts, C., Molenberghs, G., Kenward, M.G. and Neiner, C. (2014). The surface nitrogen
abundance of a massive star in relation to its oscillations, rotation, and magneticfield. The Astrophysical journal, 781, 2. (KUL-1/UH and LSHTM)
Andriyana, Y., Gijbels, I. and Verhasselt, A. (2014). P-splines quantile regression esti-
mation in varying coefficient models. Test, 23, 153–194. (KUL-1 and UH)
Aregay, M., Shkedy, Z. and Molenberghs, G. (2013). A hierarchical Bayesian approach
for the analysis of longitudinal count data with overdispersion: A simulation study.
Computational Statistics & Data Analysis, 57(1), 233–245. (KUL-1 and UH)
Aregay, M., Shkedy, Z. and Molenberghs, G. (2013). Comparison of additive and multi-
plicative bayesian models for longitudinal count data with overdispersion parameters:a simulation study. Communications in Statistics: Simulation and Computation.
(KUL-1 and UH)
Aregay, M., Shkedy, Z., Molenberghs, G., David, M. and Tibaldi, F. (2013). Model
based estimation of long–term persistence of induced HPV antibodies; a flexiblesubject–specific approach. Journal of Biopharmaceutical Statistics, 23(6), 1228–1248. (KUL-1 and UH)
Ceulemans, E., Hubert, M. and Rousseeuw, P.J. (2013). Robust Multilevel Simultaneous
Component Analysis, Chemometrics and Intelligent Laboratory Systems, 129, 33–39. (KUL-2 and KUL-1).
Croux, C., Haesbroeck, G. and Ruwet, C. (2013). Robust estimation for ordinal regres-
sion. Journal of Statistical Planning and Inference, 143, 1486–1499. (KUL-1 andULG)
Croux, C., Ronchetti, E., Salibian-Barrera, M. and Van Aelst, S. (2013). Special issue
on robust analysis of complex data of Computational Statistics & Data Analysis, 65,1–3. (KUL-1 and UG)
De Boever, P., Wens, B., Forcheh, Anyiawung C., Reynders, H., Nelen, V., Kleinjans, J.,
Van Larebeke, N., Verbeke, G., Valkenborg, D. and Schoeters, G. (2014). Charac-terization of the peripheral blood transcriptome in a repeated measures design usinga panel of healthy individuals. Genomics, 103, 1, 31–39. (KUL-1 and UH)
Del Fava, E., Shkedy, Z., Aregay, M. and Molenberghs, G. (2013). Modeling multivari-
ate, overdispersed binomial data with additive and multiplicative random effects.
Statistical modelling (KUL-1 and UH)
De Roover, K., Ceulemans, E., Timmerman, M.E., Nezlek, J.B. and Onghena, P. (2013).
Modeling differences in the dimensionality of multiblock data by means of clusterwisesimultaneous component analysis. Psychometrika, 78, 648–668. (KUL-2 and RUG)
De Roover, K., Timmerman, M.E., Mesquita, B. and Ceulemans, E. (2013). Common
and cluster-specific simultaneous component analysis. PLoS ONE, 8, e62280, 1–14.
(KUL-2 and RUG)
De Roover, K., Timmerman, M.E., Van Diest, I., Onghena, P. and Ceulemans, E. (2014).
Switching principal component analysis for modeling means and covariance changesover time. Psychological Methods, 19, 113–132. (KUL-2 and RUG)
De Roover, K., Timmerman, M.E., Van Mechelen, I. and Ceulemans, E. (2013). On the
added value of multiset methods for three-way data analysis. Chemometrics andIntelligent Laboratory Systems, 129, 98–107. (KUL-2 and RUG)
Donneau, A., Mauer, M., Lambert, P., Molenberghs, G. and Albert, A. (2013) Simulation-
based study comparing multiple imputation methods for non–monotone missing or-dinal data in longitudinal settings. Journal of Biopharmaceutical Statistics. (ULGand KUL-1/UH)
Efendi, A., Molenberghs, G., Njagi, E. and Dendale, P. (2013). A joint model for longi-
tudinal continuous and time to event outcomes with direct marginal interpretation.
Biometrical Journal, 551(4), 572–588. (KUL-1 and UH)
Forcheh, A. C., Verbeke, G., Kasim, A., Lin, D., Shkedy, Z., Talloen, W. Goehlmann, H.
and Clement, L. (2013). beadarrayFilter: An R package to filter beads. R Journal,5(1), 171–180. (KUL-1, UH and UG)
Geerdens, C., Claeskens, G. and Janssen, P. (2013). Goodness-of-fit tests for the frailty
distribution in proportional hazards models with shared frailty. Biostatistics, 14(3),433–444. (KUL-1 and UH)
Getachew, Y., Janssen, P., Yewhalaw, D., Speybroeck, N., Duchateau, L. (2013). Coping
with time and space in modelling malaria incidence: a comparison of survival andcount regression models. Statistics in Medicine, 32, 3224–3233. (UH and UG)
Gijbels, I., Herrmann, K. and Verhasselt, A. (2013). Discussion to the paper "Large
covariance estimation by thresholding principal orthogonal components", by Fan,J., Liao, Y. and Mincheva, M. Journal of the Royal Statistical Society, Series B, 75,603–680. Discussion on pages 662–663. (KUL-1 and UH).
Gijbels, I. and Omelka, M. (2013). Testing for homogeneity of multivariate dispersions
using dissimilarity measures. Biometrics, 69, 137–145. (KUL-1 and CU)
alez Montoro, A., Cao, R., Faes, C., Molenberghs, G., Espinosa, N., Cudeiro, J. and
Marino, J. (2014). Cross nearest–spike interval based method to measure synchronydynamics. Mathematical Biosciences and Engineering, 11, 27–48. (KUL-1/UH andUSC)
Ip, E., Molenberghs, G., Chen, S.H., Goegebeur, Y. and De Boeck, P. (2013). Function-
ally unidimensional item response models for multivariate binary data. MultivariateBehavioral Research, 48, 534–562. (KUL-2 and KUL-1/UH)
Jaspers, S., Aerts, M., Verbeke, G. and Beloeil, P.-A. (2013). A new semi-parametric
mixture model for interval censored data, with applications in the field of antimicro-bial resistance. Computational Statistics & Data Analysis. (KUL-1 and UH)
Jaspers, S., Aerts, M., Verbeke, G. and Beloeil, P.-A. (2014). Estimation of the wild–
type minimum inhibitory concentration value distribution. Statistics in Medicine,33, 289–303. (KUL-1 and UH)
Kassahun, W., Neyens, T., Molenberghs, G., Faes, C. and Verbeke, G. (2013). A joint
model for hierarchical continuous and zero-inflated overdispersed count data. Jour-nal of Statistical Computation and Simulation. (KUL-1 and UH)
Kassahun W., Neyens T., Faes C., Molenberghs G. and Verbeke G. (2014). A zero–
inflated overdispersed hierarchical Poisson model. Statistical Modelling. (KUL-1and UH)
Kenward, M. G. and Molenberghs, G.(2013). A Taxonomy of Mixing and Outcome
Distributions Based on Conjugacy and Bridging. Communications in Statistics –Theory and Methods. (LSHTM and KUL-1/UH)
Klingels, K., Feys, H., Molenaers, G., Verbeke, G., Van Daele, S., Hoskens, J., Desloovere,
K. and De Cock, P. (2013) Randomized Trial of Modified Constraint–Induced Move-ment Therapy With and Without an Intensive Therapy Program in Children WithUnilateral Cerebral Palsy. Neurorehabilitation and neural repair, 27(9), 799–807.
(KUL-1 and UH)
Mallinckrodt, C., Lin, Q., Carpenter, J., Kenward, M. and Molenberghs G. (2013). A
structured framework for assessing sensitivity to missing data assumptions in lon-gitudinal clinical trials. Pharmaceutical Statistics, 12(1), 1–6. (LSHTM and KUL-1/UH)
Milanzi, E., Molenberghs, G., Alonso, A., Kenward, M., Tsiatis, A., Davidian, M. and
Verbeke, G. (2014). Estimation after a group sequential trial. Statistics in Bio-Sciences. (UH, KUL-1 and LSHTM)
Molenberghs, G., Kenward, M. G., Verbeke, G., Iddi, S. and Efendi, A. (2013). On
the Connections Between Bridge Distributions, Marginalized Multilevel Models, andGeneralized Linear Mixed Models. International Journal of Statistics and Probabil-ity, 2(4), 1–21. (UH, KUL-1 and LSHTM)
Molenberghs, G., Kenward, M., Aerts, M., Verbeke, G., Tsiatis, A., Davidian, M. and
Rizopoulos, D. (2014). On random sample size, ignorability, ancillarity, complete-ness, separability, and degeneracy: sequential trials, random sample size, and missingdata. Statistical Methods in Medical Research, 23, 11–41. (UH, KUL-1 and LSHTM)
Njeru Njagi, E., Molenberghs, G., Rizopoulos, D., Verbeke, G., Kenward, M., Dendale, P.
and Willekens, K. (2013). A flexible joint–modelling framework for longitudinal andtime–to–event data with overdispersion. Statistical Methods in Medical Research.
(UH, KUL-1 and LSHTM)
Omelka, M., Veraverbeke, N. and Gijbels, I. (2013). Bootstrapping the conditional cop-
ula. Journal of Statistical Planning and Inference, 143, 1–23. (CU, UH and KUL-1)
Thilakarathne, P., Verbeke, G., Engelen, K., Marchal, K. and Lin, D. (2013). Identifying
Differentially Expressed genes in the Absence of Replication. International Journalof Bioinformatics Research and Applications, 9, 71–90. (KUL-1 and UH)
Timmerman, M.E., Ceulemans, E., De Roover, K. and Van Leeuwen, K. (2013). Subspace
K-means clustering. Behavior Research Methods, 45, 1011–1023. (RUG and KUL-2)
Veraverbeke, N., Gijbels, I. and Omelka, M. (2014). Pre-adjusted nonparametric estima-
tion of a conditional distribution function. Journal of the Royal Statistical Society,Series B, 76(2), 399–438. (UH, KUL-1 and CU)
Van Deun, K., Smilde, A.K., Thorrez, L., Kiers, H.A.L. and Van Mechelen, I. (2013).
Identifying common and distinctive processes underlying multiset data. Chemomet-rics and Intelligent Laboratory Systems, 129, 40–51. (KUL-2 and RUG)
Refereed publications (in press, accepted for publication).
ormann, S., Horv´
ath, L. and Huˇskov´
a, M. (2014). Dependent functional
linear models with applications to monitoring structural change. Statistica Sinica,to appear. (ULB and CU)
Bremhorst, V. and Lambert, P. (2014). Flexible estimation in cure survival models using
Bayesian P-splines. Computational Statistics and Data Analysis, to appear. (UCLand ULG)
Colling, B., Heuchenne, C., Samb, R. and Van Keilegom, I. (2013). Estimation of the
error density in a semiparametric transformation model. Annals of the Institute ofStatistical Mathematics, to appear. (ULG and UCL)
Donneau, A.F., Mauer M., Lambert, P., Molenberghs, G. and Albert, A. (2014). Simulation-
based study comparing multiple imputation methods for non-monotone missing ordi-nal data in longitudinal settings. Journal of Biopharmaceutical Statistics, to appear.
(ULG, KUL-1/UH).
Magis, D. and De Boeck, P. (2014). Type I error inflation in DIF identification with
Mantel-Haenszel: an explanation and a solution. Educational and PsychologicalMeasurement, to appear. (ULG and KUL-1)
Books & Book Chapters (in press).
Claeskens, G. and Jansen, M. (2014). Model selection (and model averaging). In Inter-
national encyclopedia of social and behavioral sciences, second edition. Editor J.D.
Wright, Elsevier, Oxford. To appear. (KUL-1 and ULB)
De Roover, K., Timmerman, M.E., De Leersnyder, J., Mesquita, B. and Ceulemans,
E. (in press). Whats hampering measurement invariance: Detecting non-invariantitems using clusterwise simultaneous component analysis. Frontiers in Psychology,to appear. (KUL-2 and RUG)
Source: https://iap-studys.be/documents/report-2014
The Exclusive Vitamin RECOMMENDED DIETARY INTAKE FOR μg vitamin B per day Vitamin B is found naturally in all foods of animal origin and a few foods that have been fortified.Symptoms of vitamin B deficiency can take several years to develop as liver stores may be large and vitamin B is recycled internally. In older people, however, the ability to absorb vitamin B from
Canti del salto e della tanca CANTI DEL SALTO E DELLA TANCA LiaIl focolareIl presente MUTTOSPrimaveraCuori lontaniCuori lontaniSaluto dal CampidanoIl mietitoreIl violentoLa luna neraSposaLa portatrice d'acquaLa surbileIl banditoIl nomadeLa madreSan FrancescoGonareNovembreAprileIl falcoL'aquilaAuguraleIl cacciatoreNuoro d'invernoA VindicinoAll'amataStella L'AUTOMOBILE PASSAIl villaggioLo stazzoLa tancaLa bardanaIl poeta