Yansong.org
The Power of Not Asking: How Do Generic Drug Substitution Laws Affect Patient's
Demand for Generic Drugs? 1
Yan Song2 Douglas Barthold 3
Current Version: Nov/9/2015
Substituting generic for brand drugs whenever possible has been proposed as an effective way to control pre-scription drug expenditure growth in the United States. This work investigates whether mandatory switchingand presumed consent laws are effective in increasing generic drug use. The analysis uses plausibly exoge-nous changes in states' drug substitution policies for identification. The Difference-in-Difference regressionresults indicate that mandatory switching laws have little effect. However, presumed consent laws, wherebythe pharmacists could assume patients' consent to switch to generic drugs, reduce consumers' probability ofpurchasing brand drugs by 4.1 percentage points. We construct and estimate a bounded rationality modelto explain why presumed consent laws work. In the model, consumers in states with presumed consent lawsincur a cost when asking pharmacists for brand drugs. We find that presumed consent laws' effect in de-creasing brand drug use is equivalent to increasing the brand drug price by 3 dollars. The average marginaleffect of the policy is to reduce the probability of purchasing brand drugs by 6 and 11 percent. A welfarecalculation indicates that consumers' surplus loss exceeds the insurances' gain by 7.5 dollars per person perpurchase when states switch from explicit to presumed consent laws.
1We would like to thank Fabian Lange, Erin Strumpf and Robert Clark for their continued guidance and support during this
project. We thank seminar participants at McGill University and HEC Montreal for helpful suggestions.
2Ph.D. Candidate, McGill University,
[email protected].
3Post-doctoral fellow, Schaeffer Center for Health Policy and Economics, University of Southern California, dou-
The rising costs of health care expenditures in the United States have captured the at-
tention of academics and policy makers. In 2013, national health expenditures totaled 2.9
trillion dollars, or 17.4 percent of US GDP.4 Ten percent of this spending went towards pre-
scription drugs. Finding an effective way to control drug expenditure has become a priority
for policy makers. A generic drug is usually priced at 10 to 20 percent of the brand name
counterpart. One possible way to control the drug expenditures is to dispense generic drugs
whenever a multi-source drug is prescribed. For instance, Kelton et al. (2013) estimates that
state Medicaid programs could have saved 220 million dollars when generic fluoxetine came
to market between 2001 and 2005. 5
However, it has been well documented that consumers have a high preference for partic-
ular brands, even when the alternatives are objectively similar. The majority of consumers
typically buy a single brand of beer, cola, or margarine even though relative prices vary
significantly over time. Other work finds substantial brand price premium in a wide range
of non-consumer package goods, such as automobiles (Sullivan et al. (1994)), index funds
(Hortaçsu and Syverson (2003)), and online books (Smith and Brynjolfsson (2001)). In the
prescription drug market, Ling et al. (2002) document that a large fraction of consumers
buys branded medications, even though generic substitutes are available at the same stores
for much lower prices. The preference for brand is also known to be persistent and difficult
to change. Beshears et al. (2013) document that short peer testimonials do not increase
the impact of a mailed communication on conversion rates to generic drugs, even when the
testimonial is presented as coming from socially proximate peers. Carrera and Villas-Boas
(2013) find that information on the comparability of generics has no effect on the generic
purchase rate relative to the national brand.
Given the potential savings of generic substitution and the well known consumers' prefer-
4http://www.cdc.gov/nchs/fastats/health-expenditures.htm. Last accessed Aug 16, 2015.
5A multi-source drug is defined as having at least one other drug rated as therapeutically equivalent under the Food and
Drug Administration's most recent publication of "Approved Drug Products with Therapeutic Equivalence Evaluations."
ence for brands, it is important to understand what type of policy could effectively increase
generic drug substitution rates. In this paper, we evaluate two types of policies employed by
states in the United States to increase generic drug use at the retail level. The first type of
law regulates whether it is mandatory or permissive for pharmacists to switch to a generic
drug. The second type of law mandates whether the patient consent is presumed or has to
be explicitly acquired. Presumed consent laws imply pharmacists assume that the patient
agrees with the generic substitution unless the patient explicitly rejects the substitution.
Explicit consent laws require that pharmacists must ask for patients' permission to switch
to a generic drug. A state can choose any combination of the two types of policy tools.
Intuitively, mandatory switching and presumed consent laws should both increase the use
of generic drugs. However, there has been no systematic study to quantify the impacts of
these laws. The only recent study by Shrank et al. (2010) analyzes the laws' impacts on
the generic substitution ratio for Zocor6. They find that states implementing policies that
require patient consent prior to generic substitution experienced 25 percent lower rates of
generic substitution. But more questions remain to be answered. For instance, does the
presumed patient consent law have the same level of impact on every type of drug? Why
does the presumed patient consent law work? What would happen if all states switch from
explicit consent law to presumed consent law?
This paper tries to answer these questions. We provide the first comprehensive analysis
of how state generic substitution laws impact the prescription drug market. We collect data
on state laws of generic substitution between 2006 and 2012. During the seven year period,
eleven states changed their policy on generic drug substitution. For example, Michigan
changed from not requiring to requiring consent, while Alabama chose not to require consent
in 2009. We exploit these changes to identify the impacts of generic substitution policies on
generic drug use.
We merge the law data with the Medical Expenditure Panel Survey (MEPS) data. The
6Zocor is used to lower cholesterol and triglycerides (types of fat) in the blood.
MEPS prescribed medicine file provides detailed information on household-reported pre-
scribed medicines for a nationally representative sample of the civilian noninstitutionalized
population of the United States. Each record represents one household-reported prescribed
medicine that was purchased in that year.
Our analysis includes two parts. In the first part, we empirically establish the impacts of
the policies using the difference in differences method. To capture the potential heterogene-
ity of the laws' effects, we look at nine drugs within three types of drug categories: statins,
antidepressants/SSRIs and proton pump inhibitors (PPIs).7 We find that mandatory switch-
ing laws have little impact on the generic drug use compared to permissive switching laws.
Mandatory switching laws do not decrease the probability of purchasing brand drugs more
than the permissive law for all nine drugs. In comparison, the presumed patient consent law
significantly decreases the probability of consumers buying a brand rather than a generic
drug for seven out of nine drugs. The magnitude of the impact depends on the molecule
under consideration, ranging from a 20 to 70 percent decrease in the probability of buy-
ing a brand name prescription drug. To verify the validity of these results, we implement
a falsification test. The intuition for the test is that consumers' preference of brand over
the counter (OTC) drugs should not be affected by the policies regulating the substitution
of prescription drugs. We find that these policies do not affect whether consumers choose
brand or generic OTC drugs. We also look at how pharmaceutical firms adjust their pricing
strategies in response to these policy changes. We find that the state generic drug substi-
tution laws have no significant impact on consumers' copayment and the total payment for
prescription drugs.
After establishing the impacts of the policies on generic substitution, we attempt to
understand the mechanisms that drive the effects of the presumed consent policy, and its
welfare implications. We model the presumed patient consent law as an extra cost for
patients living in states with such policy to buy brand name drugs. We estimate the size
7These drugs are used to reduce cholesterol levels, treat depression, and reduce gastric acid production, respectively.
of the cost parameter by modeling the consumer's choice of prescription drug as a discrete
choice problem. We find that the effect of the presumed consent law on the patient's decision
to buy prescription drugs is equivalent to a three dollar increase in the price of a brand name
drug. The average marginal effect of the policy is that the average probability of purchasing
brand name drugs decreases by six to eleven percent. A welfare calculation indicates that
the consumer's surplus loss exceeds the insurer's gain by 7.5 dollars per person when all
states switch from explicit to presumed consent.
The rest of the paper is organized as follows. Section 2 discusses the related literature and
this paper's contributions. Section 3 provides background information on the pharmaceutical
market and the state laws regulating generic substitution. Section 4 applies the difference
in differences method. Section 5 models the presumed consent law in a consumer's discrete
choice problem, and Section 6 concludes.
2. Literature Review
With the recent increase in pharmacy spending, pharmacy benefit managers and health
plans have adopted benefit changes designed to reduce pharmaceutical use or steer patients
to less-expensive alternatives. These cost sharing features of prescription drug benefits in-
clude tiered copayments, benefit caps, prior authorization, closed formularies, and reference
pricing. Goldman et al. (2007) reviews articles that study the associations between prescrip-
tion drug cost sharing and medication and medical utilization, spending, and health. These
studies show that increased cost sharing is associated with lower rates of drug treatment,
worse adherence among existing users, and more frequent discontinuation of therapy. For
each ten percent increase in cost sharing, prescription drug spending decreases by two to six
In comparison, much less attention has been paid to the impact of state drug substitution
laws on generic drug use. Shrank et al. (2010) evaluate the relationship between different
generic substitution policies and generic simvastatin use after patent expiration of Zocor.
They find that states implementing policies that require explicit consent prior to generic
substitution experienced 25 percent lower rates of generic substitution. This study suffers
from several limitations. By limiting themsevles to one type of drug, they could not capture
potential heterogeneous effects of the laws. Further, they do not discuss why the presumed
consent law was more effective than the mandatory substitution law. Kelton et al. (2013)
examine the movement to generic fluoxetine following patent expiration for Prozac, a widely
prescribed antidepressant. They find large differences in states' responses to generic avail-
ability. States take between two and ten calendar quarters to reach 90 percent use of generic
rather than brand-name fluoxetine. However, they do not explore the potential causes of
these variations.
This paper is related to the literature on the choice of prescription drugs. This literature
has largely focused on the role of physicians' preferences and learning. Hellerstein (1998)
examines the importance of physicians in the process by which patients receive either brand
name or generic drugs. Using a data set on physicians, their patients, and the multisource
drugs prescribed, she finds that very little of the prescription decision can be explained
by observable characteristics of individual patients, but all of the evidence indicates that
physicians are indeed an important agent in determining whether patients receive either
trade-name or generic drugs. Coscelli (2000) studies the contribution of doctor and patient
habits to persistence in market shares in prescription drug markets. He finds significant
evidence of time-dependence in prescription choices for both doctors and patients. Other
important work includes Coscelli and Shum (2004), Crawford and Shum (2005) and Lundin
The presumed consent law is widely applied in other scenarios. Abadie and Gay (2006)
study the impact of presumed consent legislation on cadaveric organ donation. Under pre-
sumed consent legislation, a deceased individual is classified as a potential donor in absence
of explicit opposition to donation before death. They construct a data set on organ donation
rates and potential factors affecting organ donation for 22 countries over a 10-year period.
They find that differences in other determinants of organ donation explain much of the varia-
tion in donation rates. Still, even after controlling for those determinants, presumed consent
legislation has a positive and sizable effect on organ donation rates. Another widely known
application of presumed consent rules is the default opt-in structure of the 401(k) savings
plans in the United States. Madrian and Shea (2000) find that automatic enrollment in
401(k) dramatically changes the savings behavior of employees. Specifically, they find that
401(k) participation is significantly higher under automatic enrollment. Thaler and Benartzi
(2004) and Chetty et al. (2012) study similar issues and come to the same conclusions. Car-
roll et al. (2005) model the 401(k) enrollment and derive conditions under which the optimal
enrollment regime is automatic enrollment (i.e., default enrollment), standard enrollment
(i.e., default non-enrollment), or active decisions. Thaler (2008) provides various other ex-
amples of presumed consent. Most of the existing literature on the presumed consent policy
uses a reduced form approach to quantify the effect of presumed consent. An exception is
Handel (2013). He investigates consumer inertia in health insurance markets. He leverages
a major change to insurance provision that occurred at a firm to identify substantial inertia.
He estimates a choice model, and uses these estimates to study the impact of policies that
nudge consumers toward better decisions by reducing inertia. Similar to Handel (2013),
we view the presumed consent as a policy that nudges consumers towards better decisions
by increasing inertia. We model the presumed consent policy as extra costs to buy brand
drugs. We contribute to the literature by developing a discrete consumer choice model, and
estimate the size of the cost incurred by the presumed consent policy.
3. Background Information of the Pharmaceutical Industry and Generic Substi-
tution Laws
3.1. Background Information of the Pharmaceutical Industry
The pharmaceutical industry is one of the most heavily regulated industries in the United
States. A firm has to file a New Drug Application (NDA) for FDA approval to be able
to market a new drug. Once approved, this drug is granted market monopoly under the
protection of a patent. A patent usually lasts about 20 years. After the drug's patent expires,
other firms can apply for FDA approval to produce a generic version of that drug. Before the
passage of the Hatch-Waxman act in 1984, generic producing firms had to go through the
same stage of clinical trials as the brand name firm to prove the drug's efficacy and safety.
After 1984, generic firms could simply demonstrate bio-equivalence with branded products
by showing that the active ingredient in their product diffused into the human bloodstream
in a manner similar to the original product. This act dramatically changed the landscape
of the pharmaceutical market (Boehm et al. (2013)). Generic drugs now enjoy about 70
percent of the market, compared to about 10 percent in the 1980s.
3.2. Background Information of the Generic Drug Substitution Laws
3.2.1. Generic Drug Substitution Laws in the US
At one time, laws in most states required the pharmacist to fill a prescription as written,
precluding generic dispensing when the physician had written the brand name, but the
last of these antisubstitution laws was repealed in 1984 (most were repealed in the mid- to
late-1970s). They were replaced by two types of laws regulating the substitution of generic
drugs. The first type of law can be classified into two categories, permissive or mandatory.
An example of the mandatory switching law is the legislation in Florida:
A pharmacist who receives a prescription for a brand name drug shall, un-
less requested otherwise by the purchaser, substitute a less expensive, generically
equivalent drug product.8
An example of the permissive switching law is the Illinois legislation:
A different brand name or non-brand name drug product of the same generic
name may be dispensed by the pharmacist, provided that the selected drug has a
unit price less than the drug product specified in the prescription.9
The second type of law determines the nature of consent, explicit or presumed, that is
0499/0465/Sections/0465.025.html. Last accessed Aug 14, 2015
Last accessed Aug 14, 2015
Table 1: Summary of Generic Drug Substitution Policy Regulating Environment.
Pharmacists must switch, and
Pharmacists can switch, and
need to acquire explicit patient
need to acquire explicit patient
Presumed Consent Pharmacists must switch, and
Pharmacists can switch, and
assume patient consent.
assume patient consent.
Note: These are the possible scenarios for the two types of generic drug substitution laws examined in thisstudy, for situations in which a brand name drug is prescribed, and a generic drug that has been rated asbioequivalent by the FDA is available. In all cases, the patient can overrule the substitution and opt fortheir preferred choice.
required for substitution. An example of the explicit consent law is the regulation in Penn-
Any pharmacist who substitutes any drug shall notify the person presenting
the prescription of such substitution together with the amount of the retail price
difference between the brand name and the drug substituted for it and shall inform
the person presenting the prescription that they may refuse the substitution.10
Compared to the explicit consent law, the presumed consent law presumes consent from the
consumer. It only indicates on the label of the drug that the substitution has taken place.
An example is the regulation in Massachusetts:
The pharmacist shall indicate on the label in the following manner the fact of
the interchange: "Interchange (name of exact drug product dispensed)"11
States can choose any combination of the two types of laws. Consumers can always refuse
the substitution and choose to pay more to get the branded drug. Table 1 summarizes the
possible generic drug substitution policy regulating environment.
3.2.2. Generic Drug Substitution Laws in Europe
Similar to the US, Europe has also adopted certain policies to increase the use of generic
drugs. Table 2 shows how different countries regulate generic drug substitution. Generic
Last accessed Aug 14, 2015
11https://malegislature.gov/Laws/GeneralLaws/PartI/TitleXVI/Chapter112/Section12D. Last accessed Aug 14, 2015
Table 2: Regulation of How Pharmacists Should Substitute Generic Drugs in Europe
Mandatory inpublic sector
Notes: * Mandatory substitution for antibiotics and antimycotics only. Sources : Vogler (2012)
substitution by pharmacists is mandatory in seven, permissive in fifteen, and disallowed in
five E.U. member countries. There is less information available on whether patients' consent
is required explicitly or can be presumed. In a cross country comparison, the generic drug
market share is usually higher in countries with mandatory substitution law. Countries which
disallow generic substitution usually have the lowest generic drug market shares.12 However,
since countries in Europe vary significantly in the reimbursement scheme for wholesalers and
pharmacies, economic development, and other variables associated with generic drug switch,
this evidence does not allow us to identify the effects of the mandatory substitution law.
3.2.3. Evolution of the US Generic Substitution Laws between 2006 and 2012
We take the data from Survey of Pharmacy Laws between 2006 and 2012. Figure 1 plots
12See Carone et al. (2012) for a more detailed generic drug market share among EU member countries.
Figure 1: State Generic Drug Substitution Laws Atlas
the distribution of how the US states choose the generic drug substitution laws.
From the figure, we see that the majority of states require explicit patient consent and
are permissive towards generic drug substitution, but all four possible combinations of the
two laws exist. Table 3 breaks down the distribution of the law types. In 2006, there were
three states in the permissive and presumed consent group, and there were also three states
in the mandatory and explicit consent group. Seven states belonged to the group that had
presumed consent and mandatory substitution. The majority of states were permissive and
required explicit patient consent for substitution, with 37 states in this group. During the
seven years period between 2006 and 2012, several states changed their generic substitution
laws. Panel B in 3 presents the breakdown of the state laws in 2012. In total, eleven states
changed these laws. Table 4 shows the details of these policy changes. Changes in both
directions exist. For example, Michigan switched from not requiring to requiring consent,
while Alabama chose not to require consent in 2009.
4. Empirical Analysis of the Impacts of the State Regulations of Generic Drug
We exploit exogenous variation from the law changes discussed above to identify the
impact of these laws. We merge the law data with the Medical Expenditure Panel Survey
(MEPS) data from 2006 to 2012. In this section, we will describe the data used and discuss
the empirical results.
Table 3: State Generic Substitution Laws
Presumed Consent 7
Presumed Consent 5
Note : The data of the state regulations of the generic drugsubstitution is taken from various years of Survey ofPharmaceutical Laws.
Table 4: US State Generic Drug Substitution Policies Changes between 2006 and 2012
Mandatory to Permissive Law
Minnesota, Mississippi, New
York, Pennsylvania, and West
Permissive to Mandatory Law
Oregon and Wyoming
Presumed to Explicit Consent
Explicit to Presumed Consent
Explicit to Presumed Consent
Permissive to Mandatory Law
Note: This table is based on Survey of Pharmacy Laws between years 2006 and 2012.
4.1. Data Description
We use two sections of the MEPS data, the household component and the prescribed
medicines file. The household component (HC) has a rich set of information on demographic
characteristics, health conditions, health status, and use of medical services. The prescribed
medicines file provides detailed information on household-reported prescribed medicines.
Each record represents one household-reported prescribed medicine that was purchased in
In the empirical work below, we focus on three types of drugs: statins, antidepressants,
and PPIs. These drugs are used to lower the level of cholesterol, treat depression, and
reduce gastric acid production, respectively. We select statins and antidepressants because
heart disease and mental disorder are among the top three most costly conditions according
to the MEPS data.13 We add PPIs to the sample to examine whether the laws impact
vary depending on the severity of the disease. Table 5 shows the brand name drugs, their
corresponding generic drug name, and the years that the brand and generic drug became
available. All nine drugs have generic counterparts between 2006 and 2012.
Table 6 breaks down the sample restriction process. The original sample has about two
million prescription drug purchase events. The sample shrinks to about eighteen thousand if
we keep only selected drugs. In the final sample, we have about sixteen thousand prescription
drug purchase events between 2006 and 2012.
In the MEPS prescribed medicines file, each record contains the information about the
drug name, quantity of the drug purchased, strength of the drug, copayment amount, and
total cost of the drug. We use the information of the quantity and strength of the drug
to create a variable indicating the number of months supplied in this purchase. Based on
these variables, we obtain the copayment and total cost of purchasing one month's supply of
the drug. Table 7 shows the average copayment, average total cost, and average copyament
by insurance status14 calculated from MEPS data between 2006 and 2007. Based on the
13MEPS Statistical brief #33114The total payments are defined as the sum of payments for care received, including out-of-pocket payments and payments
made by private insurance, Medicaid, Medicare, and other sources.
Figure 2: Evolution of the Average Copayment for One Month's Supply of Selected Brand
average copayment, the generic drugs are cheaper than the brand name drugs. Consumers
pay approximately 13 to 50 percent of the brand drug price to get generic drugs. Not only
is the copayment lower for generic drugs compared to brand name drugs, the total cost of
generic drugs is also lower. The combined payments from insurance and patient for generic
drugs range from 20 to 90 percent of the total costs of brand name drugs. The level of
copayment varies depending on the type of insurance the patient has. Patients covered with
private insurance always have the lowest out-of-pocket payment and uninsured patients pay
the most. This pattern is consistent regardless of the drug purchased. In figure 2 and 3, we
plot the copayment change for brand name and generic drugs over time. The copayments
for brand and generic drugs declined in this period.
In figure 4, we plot the share of generic drugs within each molecule between 2006 and
2012. The share is calculated based on the months' supplied for each drug in each year.
All generic drug market shares have an upward trend between 2006 and 2012, but the level
of the share varies depending on the molecule. The generics of Celexa, Zoloft, Paxil, and
Prozac have reached about 90 percent share at the end of the period, while other generics
have less than 50 percent.
Table 8 presents the demographic information for consumers who purchased antidepres-
sants, statins, or PPIs, grouped according to patient consent law regime. We can see that
Table 5: Drug Information by Disease Type
Year Brand Available
Year Generic Available 1996
Year Brand Available
Year Generic Available 2009
Year Brand Available
Year Generic Available 2006
Note : The year of drug availability is obtained from the date of FDA approval.
Table 6: Sample Restrictions of the MEPS data for Difference in Differences Analysis
Selection Criterion
Number ofObservations
Keep prescription drug purchase events for
antidepressants, PPIs, and statinsKeep if both generic and brand drugs are available in
Keep if drug strength variable has valid value
Drop if purchase quantity is less than one week or
more than one year supply
Table 7: Average Copayments and Total Payments for One Month's Supply of Selected Drugs
Brand/Generic Average
Citalopram Generic
Escitalopram Generic
Lansoprazole Generic
Omeprozole Generic
Pravastatin Generic
Simvastatin Generic
Note : The copay and total payment is calculated for a purchase of one month's supply of each drugwith MEPS data. The public insurance includes Medicare, Medicaid, and other public health insuranceprograms. The time frame is between 2006 and 2012. All copayments and payments are in dollars.
Figure 3: Evolution of the Average Copayment for One Month's Supply of Selected Generic
Figure 4: Generic Drug Share Evolution Between 2006 and 2012
Table 8: Demographic Characteristics of Patients
States with Explicit Consent
States with Presumed Consent
Private Insurance
States with Mandatory
States with Permissive
Private Insurance
Note: The data reported in this table is calculated using the sample for difference in differencesanalysis using MEPS data.
the two types of states are almost identical in terms of the demographic factors considered.
Further, we see that people who purchase these drugs are more likely to be female, white,
and covered by public health insurance.
4.2. State Regulations on the Probability of Purchasing Brand Drugs
We use the difference in differences method with the MEPS data and estimate the fol-
lowing regression.
where
P resumed_
Consentjt is an indicator for states with a presumed consent policy,
and
M andatoryjt is an indicator for states with a mandatory substitution law. Consumer
characteristics, including age, race, sex, and insurance status, are in
xit. State and year fixed
effects are included. The dependent variable is an indicator for whether the purchased drug
is brand name or generic.
First, we estimate this equation separately on all nine drugs in the three drug categories.
The estimation results are presented in Table 9. In the nine regressions estimated, the
coefficients for the presumed consent law are negatively significant in seven regressions. For
instance, the presumed consent law reduces the probability of purchasing Pravachol by about
nine percentage points. The magnitude of this effect varies, ranging from approximately a
one to nine percentage point decrease. In comparison, the coefficients for the mandatory law
are almost always not significantly different from zero. The only exception is for the drug
Then we run equation 1 with all nine drugs, and include interactions with the disease
type and the number of years the generic drug has been available. The results are presented
in Table 10. In column 1 of Table 10,
α1 is -0.041. This suggests that the presumed consent
law reduces the probability of purchasing a brand drug by 4.1 percentage points on average.
Consistent with findings in Table 10, the coefficient for the mandatory law is not significant.
This result is robust to clustered standard errors at the state level and block bootstrap
procedures suggested by Bertrand et al. (2002). In the second column, we report the results
for equation 1 with interactions between law types with the disease type, and how many
years the generic drug has been available. The results indicate that the presumed consent
law has larger impacts for antidepressants and PPIs than for statins.
4.3. Discussions and Robustness Checks
4.3.1. Interpretation of the Regression Results
At first glance, the regression results might seem counterintuitive. It is surprising that
the policy mandating substitution does not increase the sale of generic drugs more than
the permissive law. In order to understand this result, we need to explain the pharmacists'
incentives. Most insurance payments to pharmacies for prescription drugs are based on a
benchmark price called the average wholesale price (AWP). The AWP is reported by generic
producers themselves, and until recently has been subject to essentially no independent
Table 9: The Effect of State Regulations on Generic Drug Substitution by Drug
Number of Observations 13782
Number of Observations 9577
Number of Observations 14451
Note: All regressions include state and year fixed effects. Control variables includepatients' race, sex, age, and insurance status. Clustered standard errors are shown inparentheses. *p < 0.1, ** p < 0.05, *** p < 0.01.
Table 10: Difference in Differences Regression Results
Independent Variables
Falsification Test
PPI*Presumed Consent
Generic Available Years*Presumed Consent
Generic Available Years*Mandatory
Number of Observations
Note : State and year fixed effects are included. Other control variables include age, sex, race,wage in thousands, and insurance status. The omitted group is statins. Standard errors areclustered at state level, and are shown below the coefficient cells. *p < 0.1, ** p < 0.05, *** p <0.01
verification. As a result, generic producers have an incentive to compete for pharmacy
market share by reporting AWPs that exceed actual average wholesale prices. This "spread"
leads to larger pharmacy profits and make pharmacies more likely to store their products.
In general, gross profit dollars are approximately 50 percent higher for generic drugs than
for branded drugs.15 As a result, even under a permissive, rather than mandatory law,
pharmacists already have high incentives to substitute generics for brand name drugs. This
is why mandatory substitution laws do not outperform permissive laws in increasing generic
drug substitution. A natural follow up question is why pharmacists enjoy a much higher
markup for generics compared to brand drugs. The reason is that pharmacists have a much
higher bargaining power with generic drug producing firms than the brand name firms.
Pharmacists can choose among the same generic drug produced by several firms to store in
their pharmacies. However, they can only buy the branded drugs from the single firm who
has the patent. This power of choosing with generics leads to a higher markup in generics
for pharmacists.
In comparison, the presumed consent law is effective in pushing consumers to buy more
generic drugs. This result is surprising given that the literature has documented the diffi-
culty of changing consumers' preference for branded products. We will devote Section 5 to
understanding the channels of the presumed consent law's effect.
4.3.2. Endogeneity of the US Generic Drug Substitution Policy Changes
Our estimations about the impacts of the generic drug substitution policies rely on the
changes in these laws between 2006 and 2012. As Besley and Case (1994) pointed out, if
state policy making is purposeful action, responsive to economic and political conditions
within the state, then it may be necessary to identify and control for the forces that lead
policies to change if one wishes to obtain unbiased estimates of a policy's effects. This rules
applies to our estimates of the generic drug substitution policy effects. The difference in
differences estimator is not a panacea for the endogeneity problem. To alleviate concerns of
endogeneity issues, we discuss what might drive states to change the regulation on generic
drug substitution.
Due to the scarcity of media discussions of the state policies analyzed in this paper, we
look at media discussion of another type of generic drug substitution, substitution between
biologics and biosimilars. There are several reasons why the discussion of laws regulating
the substitution of biologics and biosimilars are more prevalent in the media. First, the
market for biologics and biosimilars are burgeoning. Second, the regulation of biosimilars is
still in the very nascent stages. 16Many of the proposed policies tools are similar to the ones
currently used to regulate generic drug substitution. As a result, we will discuss examples
of policy changes regulating the substitution of biosimilars for biologics to help understand
what might cause generic drug substitution policies to change.
Most of the media discussions about these policy changes show strong presence of lobbying
from pharmaceutical firms. For instance, in 2013, the Virginia House of Delegates passed
a bill restricting the ability of pharmacists to substitute generic versions of biological drugs
for brand name products.17 The Republican delegate who introduced the bill admitted that
Amgen18 reached out to him beforehand. Another example reveals the same pattern. In
North Dakota, a similar bill cleared a committee in the State Senate. The state senator who
introduced the bill said that Genentech19 had brought the bill to him.
From these examples, we can identify a common source of the changes in generic drug
substitution policies. Pharmaceutical companies producing brand name drugs often lobby
for laws favoring less generic substitution. In comparison, generic drug companies, insurance
firms, managed health care companies, and chain pharmacy stores lobby for polices that
encourage generic substitution. Though these examples are related to policies regulating
16Most biological products are more complex in structure and have larger molecules or mixtures of molecules
than conventional drugs (also called small molecule drugs).
Conventional drugs are made of pure chemi-
cal substances and their structures can be identified.
are complex mixtures that
are more difficult to identify or characterize.
Biosimilars are highly similar to the reference product they
were compared to,
but have allowable differences because they are made from living organisms.
17http://www.nytimes.com/2013/01/29/business/battle-in-states-on-generic-copies-of-biotech-drugs.html?_r=018Amgen is an American multinational biopharmaceutical company headquartered in Thousand Oaks, California.
19Genentech Inc., is a biotechnology corporation which became a subsidiary of Roche in 2009.
substitution between biologics and biosimilars, there is no reason to believe that the reasoning
would be different for policies regulating generic substitution. The lobbying power of brand
name drug firms, generic firms, insurance companies, and other related parties shape how
states regulate generic drug substitution.
The important implication for our empirical work is that pharmaceutical firms might also
lobby to change other policies that would influence the rate of generic substitution. For
example, they might lobby to change how physicians could forbid generic drug substitution,
or the way states regulate the drug formulary. Accordingly, we will examine these two policy
tools, and whether states changed them between 2006 and 2012.
States vary in how physicians can prevent generic drug substitution. Some states require
physician signature on appropriate line of the two line prescription. Others allow use of a
"Do not substitute" checkbox. And some states require handwritten "Dispense as written"
on the prescription. We checked the Survey of Pharmacy Laws between 2006 and 2012, and
found that three states changed this policy. In 2009, Illinois changed from "prescriber must
indicate: Dispense as Written in the designated box" to "prescriber must indicate may not
substitute in the designated box." Oregon changed from requiring the prescriber to write
in own handwriting "Brand Necessary," to the phrase "no substitution" or the notation
"N.S." in the practitioner's handwriting or by telephone communication. The last change
is by Montana in 2012, which changed the requirement from writing "Brand Necessary" to
"Brandname medically necessary."
These changes in state regulations of prescriber control of generic drug substitution should
not affect our estimation results, for two reasons. First, these semantic changes are minor,
and do not substantially change the way physicians can prevent generic substitution. The
effort to write "Dispense as written" is similar for to writing "May not substitute" on the
face of a prescription. A switch from handwritten messages to checking a box may have
more plausibly affected behavior, but this was not the case. Second, Shrank et al. (2011)
documents that physicians request "dispense as written" in only five percent of all prescrip-
tions. Combining the two points, we think the regression estimates should be unbiased by
confounding changes in how prescribers are regulated to prevent generic drug substitution.
Pharmaceutical firms might also lobby to change a state's formulary regulations. There
are "positive formulary" states, which identify generics that can be substituted, and there
are "negative formulary" states, which list drugs that cannot be substituted. There are also
states that do not refer to "Orange Book" standards, have neither a positive nor a negative
formulary, and allow pharmacists substitute generics as long as the drugs are pharmaceuti-
cally equivalent. In our sample period, two states changed their formulary regulations. In
2009, Delaware changed from a positive formulary to none. And in 2012, Missouri changed
from a negative formulary to none. Since all the drugs of this study are in the positive
formulary in Delaware, and not in the negative formulary in Missouri, our estimation results
should not be affected by these changes.
Another concern about the endogeneity issue is that pharmaceutical companies might
adjust their advertising budget in response to the changes in state generic drug substitution
laws. However, it is well documented in the literature that prescription drugs without patent
protection are rarely advertised by their manufacturers (Sinkinson and Starc (2015)) . This is
in contrast to over-the-counter medications, which are often advertised even through an exact
molecular substitute is available. Since all our brand name drugs have generic alternatives,
we do not think firms' responses in advertising expenditure would bias our estimation results.
4.3.3. Supply Side Pricing Responses to State Regulations of Generic Drugs
If state regulations on generic drug substitution influence consumers' choice of prescription
drugs, pharmaceutical firms are likely to adjust their pricing strategies to adapt to different
competition environments induced by these regulations. We examine firms' pricing responses
by looking at how the copayment and total payment are influenced by the state regulations.
We estimate equation 1 with copayment and total payment as dependent variables. The
regression results are presented in Table 11.
From this table, we can see that pharmaceutical pricing strategies do not respond to the
state law regulations. However, these results should be interpreted with care. Rebates from
pharmaceutical firms to insurance and health management companies are known to be an
Table 11: Pharmaceutical Firms' Pricing Response to the State Generic Drug Substitutions
Copayment Total Payment
Brand * Presumed Consent 0.131
Brand * Mandatory
Number of Observations
Note : The copayment and total payment are for one month's supply of eachdrug. State and year fixed effects are included. Standard errors are clusteredat state level, and are shown below the coefficients. *p < 0.1, ** p < 0.05,*** p < 0.01
important part of prescription drug prices. Since we have no information on the rebates,
we can not rule out the possibility that pharmaceutical firms respond to state generic drug
substitution laws by changing prescription drug prices.
4.3.4. Falsification Test of the Effect of Prescription Drug Substitution Policies on OTC
To check the robustness of our results, we implement a falsification test. The test is based
on the intuition that these laws apply only to prescription drug purchases. As a result, a
consumer's decision to buy brand name or generic OTC drugs should not be affected. We
estimate equation 1 with three OTC drugs: Claritin, Zantac, and Zyrtec.20 The regression
results are presented in column 3 of Table 10. The coefficients for both mandatory and
presumed consent law are not significantly different from zero.
20Claritin, Zantac, and Zyrtec are OTC drugs used to treat allergies, heartburn, and allergies, respectively. To make sure that
these drugs are indeed purchased over the counter, we restrict the sample to purchases for the standard OTC dosage, and forwhich the patient paid 100 percent of the cost of the drug. We considered over 50 types of OTC drugs in ten drug categories.
Only the three drugs discussed above have a meaningful number of observations in the MEPS data.
5. A Behavioral Model of the Presumed Consent Law
5.1. Consumer's Discrete Choice Model of Prescription Drugs
Reduced form estimations do not tell us why presumed consent law works and cannot
provide counterfactual experiments. To answer these questions, we build a discrete choice
model of prescription drug choice. The model is specified below.
We index our specifications by individual (
i), product (
j), and market (
m). The price of
a product varies over individuals and markets. The product price we observe in the data
is denoted
pimj. Other product attributes do not vary over markets. Their interactions
with consumer characteristics are denoted as
xij. Some attributes of the products are not
observable in the data. The utility of consumer
i living in market
m from product
j is
decomposed into observable and unobservable parts:
uij =
V (
pimj, xij) +
eij
where
xij include product characteristics, and product characteristics interacted with
observed individual characteristics.
eij is defined as the difference that makes the equation
an identity.
Our specification for
V (
.) and
eij is given as:
Uij =
α0
pimj +
βxij +
α1
∗ Brandj ∗ P resumed_
Consentim +
ϵij
There are several elements in this equation worth mentioning. First, the key element
of the model is the term
α1
∗ Brandj ∗ P resumed_
Consentim. It is an extra cost item
for consumers who would like to buy a brand name drug in a state with presumed patient
consent. The modeling decision is motivated by the intuition that a policy that mandates
generic drug substitution can be modeled as a positive infinite cost for patients buying a
brand name drug. In this case, a consumer would always choose a generic drug when facing
the infinite cost to buy a brand. Similarly a policy with no pharmacist intervention can be
modeled as no additional costs for a consumer to buy a brand name drug. The goal of the
model is to identify the cost difference between the presumed and explicit consent policies
when buying a brand name drug separately from consumer preferences.
This approach to identify the cost induced by presumed consent is in the spirit of Handel
(2013)'s method to document inertia in employer-provided health insurance. The challenge in
his work is to separately identify inertia from persistent unobserved preference heterogeneity.
He identifies the inertia by observing each family in the sample making both an "active"
and a "passive" choice from the same set of health plans over time. Similarly, we observe
consumers making decisions under presumed and explicit consent regulations. This allows
us to separately identify the cost parameter from unobserved preferences.
Another important issue, as noted by Villas-Boas and Winer (1999) and Petrin and Train
(2010), is that not accounting for endogeneity may result in a substantial bias in the param-
eter estimates of random utility models. In our model, the price
pimj variable is endogenous.
The econometric problem arises because
ϵij is not independent of
pimj, as maintained by
standard estimation techniques. We use the control function approach to deal with the
endogeneity problem. The idea behind the control function approach is to derive a proxy
variable, which conditions on the portion of
pimj that depends on
ϵij. If this can be done,
then the remaining variation in the endogenous variable will be independent of the error term
and standard estimation techniques will be consistent. In our context,
pimj can be written
as a function of all exogenous variables
xij, variables
zmj that do not enter consumer's utility
function but do impact
pimj, and a vector of unobserved terms
µmj. The key to the control
function approach is to note that, conditional on
µmj,
pimj is independent of
ϵij.
pimj =
W (
xij, zmj, µmj)
Decomposing
ϵmj into the portion that can be explained by a general function of
µmj and
the residual yields:
ϵmj =
CF (
µmj;
λ) + c
where
CF (
µmj;
λ) is the control function with parameters
λ. The simplest choice of the
control function would be a linear function in
µmj. In this case, the utility function is given
Uij =
V (
pimj, xij) +
λµmj + c
With this specification, the probability consumer
i chooses product
j is equal to
I(
Uij > Uil∀j ̸=
l)
f (
βi, c
In order to complete the specification, we make a distributional assumption for
f (
.). We
use normal and logit for the distributional assumption for
β and b
ϵ.
The expression of the likelihood for the model is
L(
β, α) = Π
I Π
j (
Pij )
yij
where
yij = 1 if person
i chooses
j, and zero otherwise.
The log-likelihood function is then
LL(
β, α) =
The specification is easily generalized to allow for repeated choices by each sampled de-
cision maker. The simplest specification treats the coefficients that enter utility as varying
over people but being constant over choice situations for each person. Utility from alterna-
tive
j in choice situation
t by person
i is
Uijt =
βiXijt +
ϵijt, with
ϵijt being IID extreme
value over time, people, and alternatives. Consider a sequence of alternatives, one for each
time period,
I =
{i1
, ., iT }. Conditional on
β the probability that the person makes this
sequence of choices is the product of logit formulas:
PI(
β) =
Π[
The unconditional probability is the integral of this product over all values of
β:
The only difference between mixed logit with repeated choices and with only one choice
per decision maker is that the integrand involves a product of logit formulas, one for each
time period, rather than just one logit formula. The probability is simulated similarly to
the probability with one choice period. A draw of
β is taken from its distribution. The logit
formula is calculated for each period, and the product of these logits is taken. This process
is repeated for many draws, and the results are averaged. In the estimation section below,
we show results with and without considering the panel feature of the data.
5.2. Model Estimation
5.2.1. Sample Construction
The estimation of the logit model uses a different sample constructed from the MEPS
dataset. There are several reasons for this. First, the multinomial logit model requires price
information for all possible product choices of consumers. However, in the dataset, we only
observe what the consumer pays out-of-pocket for their consumption choice. Therefore, we
need to impute the price they would have paid out-of-pocket for all other products. We
use the average price paid by the consumer in the same state, year, and insurance status
as the imputed price. Second, the multinomial model requires that consumer chooses the
product with the highest utility. However, we observe the same individuals making multiple
purchases of different classes of drugs in each interview round. Therefore, we keep only one
purchase event, from the highest quantity purchased. The sample restrictions and resulted
sample size are reported in Table 12. There are 19135 prescription drug choice scenarios in
the final sample.
5.2.2. Consumer Choice Model Estimation Results
The model is estimated in two steps. First, the endogenous variable is regressed on
observed choice characteristics and the instruments. The regression residuals are used to
Table 12: Sample Selection of the Prescribed Medicine Events for Logit Model Estimation
Selection Criterion
Number of Observations
Keep the ten antidepressants discussed in Table 5
Keep purchase events for treatment of major depression
Keep purchase events with valid quantity and strength information
Drop multiple purchase events for the same drug in the same interview round
Keep purchase events in the same interview round with the highest quantity
calculate the control function. Second, the choice model is estimated with the control func-
tion entering as extra variables.
We use a measure of competition in the market to instrument for price endogeneity. The
measure is the number of firms producing the drug in each market. The number of firms
producing the same drug would intensify competition and result in price decrease. The
MEPS prescribed medicine file reports the NDC code for each purchase21. We use the first
five digits to infer which company produces that drug. The first stage regression result
is shown below. We can see that increasing the number of firms producing a drug in one
market would result in the level of copayment in that market decreasing by 1.55 dollars. This
number is significant at a one percent level. For robustness checks, we tried the average price
of the product in other markets as an instrument, as suggested by Petrin and Train (2010).
The exclusion restriction requires that the price of the same product in other markets should
not be influenced by the demand side factors in this market. But it should be correlated to
the product's price in this market due to common costs of manufacturing. The regression
results are presented in the second column of Table 13. The coefficient for the number of
firms producing each drug in each market is no longer significant. But the coefficient for the
average price in all other markets is 0.98 and highly significant. The estimation results for
the choice model shown below are estimated using the number of firms producing each drug
Table 13: Instrument Regression Results
Level of Copayment
The Number of Firms Producing Each Drug in Each Market
Average Price in All Other Markets
Number of Observations
Note: Standard errors are clustered at state level, and are shown below the coefficients. *p < 0.1, ** p< 0.05, *** p < 0.01
in each market as an instrument. The other instruments yield similar results.
The consumer choice model estimation results are presented in Table 14. Columns 1 and
2 give the estimation results with and without instrumenting the endogenous variable. When
price is not instrumented, the coefficient for price is positive, which suggests an unreasonable
upward sloping demand curve. After instrumenting, the price coefficient changes to a more
reasonable estimate, -0.05. This result emphasizes the importance of instrumenting for price
when estimating a demand model. The coefficient for brand is 2.01, confirming the large
brand premium found in many other works. The estimation results in column 2 also suggest
that older and male customers are less likely to buy brand name drugs. The coefficient
for the interaction between brand name drug and presumed consent is -0.18. The ratio of
this interaction coefficient and the price coefficient indicates that the effect of the presumed
consent law on consumer choice of these antidepressants is equivalent to a 3.5 dollar increase
in the price of brand name drugs.
In column 3 of Table 14, we allow for repeated choices by each sampled decision maker
using otherwise the same specification in column 2. The first thing to notice is that there is an
improvement in the model fit, measured by the log-likelihood. The log-likelihood increased
from -35808 in column 2 to 33618 in column 3. Second, the standard deviations for the
random coefficient of price and brand dummies become highly significant. This suggest that
allowing repeated purchases for the same decision maker helps identify the random variation
in consumer preferences. The ratio of the interaction coefficient and the price coefficient
increases to about ten.
In column 4 of Table 14, we use a more flexible preference structure while continuing to
allow repeated purchases by the same decision maker. We allow consumers to have hetero-
geneous preferences in each active ingredient. We use this specification because consumers
have correlated preference for drugs with the same active ingredient. For instance, when
the generic version of Lexapro became available in 2012, it was more likely to draw con-
sumers who previously purchased Lexapro than those who purchased other antidepressants.
The flexible preference structure allows us to reproduce these more realistic substitution
patterns. The estimation results reveal several interesting points. First, the log-likelihood
increased significantly, from -33618 in column 3 to -19685 in column 4. Second, the alterna-
tive specific constants are highly significant and the standard deviations for these variables
are also significant. This indicates that consumers have different preferences for these an-
tidepressants and there are significant differences in how consumers value these products.
Third, the ratio of the coefficient for the interaction between brand and presumed consent
is around three, smaller compared to our estimates in column 2 and 3. For these reasons,
we choose this specification as our preferred specification. Our discussions below regarding
model fit, counterfactuals, and welfare evaluations are based on results estimated with this
Table 14: Consumer Choice Model Estimation Results
Heterogeneity for
Table 14: Consumer Choice Model Estimation Results
Heterogeneity for
Table 14: Consumer Choice Model Estimation Results
Heterogeneity for
Table 14: Consumer Choice Model Estimation Results
Heterogeneity for
Note : Standard errors are clustered at state level, and are shown below the coefficient. *p < 0.1, ** p < 0.05, ***
p < 0.01. In column 1, the model is estimated without instrumenting for price and without allowance for repeated
purchases by the same decision maker. In column 2, the specification uses instruments for price, without allowance
for repeated purchases by the same decision maker. Both specifications in column 3 and 4 instrument the price
and allow repeated purchases by the same decision maker.
5.2.3. Model Fit
We examine how well the model fits the data by comparing the predicted shares for all
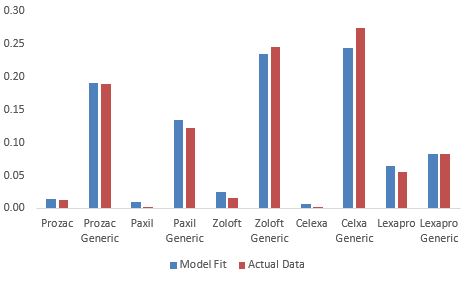
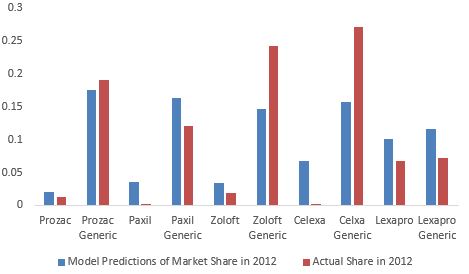
Figure 5: Predicted and Actual Shares for Antidepressants
Figure 6: Out-of-Sample Prediction and Actual Shares for Antidepressants
drugs to the actual shares. Figure 5 plots the shares in 2012 predicted by the model and
the actual shares in that year. We can see that the model does well in fitting the shares.
To further test the model's prediction power, we estimate the model on data between 2006
and 2011 and use the estimates to predict the shares in 2012. Figure 6 compares the out-of-
sample predictions to the actual data in 2012. The out-of-sample predictions track the data
reasonably well. Another way to measure the effect of the consent laws on consumer choice
of prescription drugs is to compare the prediction errors of the model when estimated with
and without the consent law variable. Therefore, we estimate the model separately with and
without the consent law variable and obtain the predicted share for all drugs. We find that
the model estimated with the consent law variable reduces the aggregate absolute prediction
error by two percentage points.
5.3. Discussion
5.3.1. Mechanisms of the Model
In the consumer choice model above, the presumed consent law is modeled as an extra
cost to buy brand name drugs. This captures the presumed consent law's impact in a par-
simonious way. There could be several behavioral channels underlying this cost parameter.
The first possibility is switching costs. The switching costs refer to the effort of the process
of asking the pharmacists to switch back to brand drugs.
The second possible channel stems from consumer's inattention. Under presumed consent
law, the switching to generic drug is indicated on the label of the prescribed drugs. If con-
sumers did not pay attention, they may not notice the switch. It has been well documented
that limited attention affects consumer decisions under various scenarios. DellaVigna and
Pollet (2009) compare the response to earnings announcements on Friday, when investor
inattention is more likely, to the response on other weekdays. They find that Friday an-
nouncements have a 15 percent lower immediate response and a 70 percent higher delayed
response. Chetty et al. (2007)show that consumers under-react to taxes that are not salient.
They use a field experiment in a grocery store and find that posting tax-inclusive price tags
reduces demand by eight percent. And they show that increases in taxes included in posted
prices reduce alcohol consumption more than increases in taxes applied at the register.
Other possible sources of the presumed consent law's impact include dynamic preference
inconsistency. For instance, a consumer might notice the switch but decide to go to the
pharmacist the next day to ask for a brand. The time inconsistency could lead consumers to
never make the change. The extra cost parameter in the choice model is meant to capture
the aggregate impact of these channels. The data does not allow us to separately identify
these models.
5.3.2. Product Substitution Patterns
It's critical that the estimated choice model predicts reasonable product substitution pat-
terns. The percentage change in the probability of choosing alternative j given a percentage
change in the price of another alternative k is:
Eijk = −pricek
Table 15: Substitution Elasticity For Selected Antidepressants
Note: In each column, we report the percentage change of market share for all drugs responding to a one percentincrease in the price of the drug indicated in the column header.
We report the substitution pattern in Table 15. There are two items worth noting. First,
the magnitude of the price elasticity is smaller compared to recent estimates found in the
literature. For instance, Simonsen et al. (2015) report the price elasticities for prescription
drugs in Denmark ranging from -0.09 to -0.25. We think this is caused by the model speci-
fication. We are calculating the price elasticity conditional on purchase. Adding an outside
option of not purchasing results in higher estimates of the price elasticities. Second, the
model exhibits significant within molecule substitution. When the price of a brand name
drug increases by one percent, the majority of the consumers who switch choose to buy the
generic drug of the same molecule.
5.3.3. Average Marginal Effects of the Presumed Consent Law
Since the model above is non-linear, we cannot directly interpret the impact of the pre-
sumed consent law based on the sign and magnitude of the coefficients reported above. To
Table 16: Average Marginal Effect of Change in Consent Law
Predicted Probability
Predicted Probability of
of Purchase under
Purchase under Presumed
Note: The predicted probabilities of purchasing drugs are calculated based on the mixed logitmodel specified in column 4 of Table 14.
ease interpretation, we calculate the average marginal effects of all states switching from an
explicit to presumed patient consent law. The results are presented in Table 16. We see that
on average, switching to presumed consent reduces the average probability of purchasing the
brand name version of a drug by approximately six to eleven percent.
5.3.4. Welfare Evaluations
From a policy maker's perspective, we would like to know the welfare implications of the
presumed consent law, in addition to understanding the impact of the policy. There are two
parties involved in the welfare calculation, insurance companies and patients. It is clear that
insurance companies gain from the presumed consent policy. From column 4 in Table 7, the
average insurance payment differs by 41 dollars between a brand and generic antidepressant.
Therefore after implementing the presumed consent policy, insurance companies gain by
paying less for drugs. Based on the predicted share of drugs in explicit and presumed
consent scenarios in Table 16 and the difference in insurance payments between a brand and
generic drug, we calculate that the insurance companies pay 0.9 dollars less per person on
On the other hand, consumer welfare would decrease because of the extra cost term for
buying a brand name drug. Under the logit assumptions, the consumer surplus associated
with a set of alternatives takes a closed form that is easy to calculate. By definition, a
person's consumer surplus is the utility, in dollar terms, that the person receives in the
choice situation. The decision maker chooses the alternative that provides the greatest
utility. Consumer surplus is therefore CSi = (1/α0)Maxj(Uij), where α0 is the marginal
utility of income. The division by α0 translates utility into dollars. We do not observe
Uij. But we observe Vij, and know the distribution of the remaining portion of the utility.
Therefore, we can calculate the expected consumer surplus.
E(CSi) =
E(maxj(Vij + ϵij))
Rosen and Small (1979) show that, if each ϵij is IID extreme value and utility is linear in
income, this expectation becomes:
E(CSi) =
eVij ) + C
where C is an unknown constant that represents the fact that absolute level of utility can not
be measured.22 The change in consumer surplus that results from a change in the consent
law can be calculated from equation 6. In particular, E(CSi) is calculated twice: first under
the conditions before the change, and again under the conditions after the change. The
difference between the two results is the change in consumer surplus:
E(CSi) =
ij − ln(
where the superscripts 0 and 1 refer to before and after the change. Since the unknown
constant C enters expected consumer surplus both before and after the change, it drops out
of the difference and can therefore be ignored when calculating changes in consumer surplus.
22We show how to derive equation 6 in the appendix.
Using equation 7, we find that the average consumer loss is about 9.17 dollars.
Combined with the welfare gain for insurance companies, this model suggests that switch-
ing from presumed consent to explicit consent would incur 7.48 dollars loss per person per
prescription. Some words of caution are necessary. First, this calculation does not consider
the supply side response and does not calculate the welfare change for drug suppliers. It is
not clear how the suppliers would react and their welfare would change. As a result, the
welfare discussion can only be a partial statement. Second, the welfare calculation considers
the psychological costs of switching away from default as a real cost. If we ignore this cost
and only consider the utility difference of choosing brand and generic drugs, the welfare loss
for consumers would be smaller.
Prescription drug spending in the US ballooned in 2014 to nearly $374 billion.23 The
ability to promote generic drug use for multi-source drugs will be key to controlling the
growth of spending on prescription drugs. State governments and insurance companies have
come up with various ways of incentivizing patients to buy generic drugs whenever possible.
These strategies include mandatory switching laws for pharmacists, presumed patient consent
laws, and tiered pricing in health insurance plans. Consumer's price elasticity for prescription
drugs has been widely studied, but there are limited studies of the effects of the state laws
on pharmacists' tendencies to switch to generic drugs.
This paper tries to fill that gap in the literature. We use changes in state laws to identify
their impacts on generic drug use. We find that the mandatory substitution law has little
effect, and the effect of presumed patient law on a patient's decision to buy prescription
drugs is equivalent to a 3 dollar increase in the brand name drug price. The average marginal
effect of the policy is that the average probability of purchasing brand name drugs decreases
between 6 and 11 percent. A welfare calculation indicates that the consumer surplus loss
exceeds the insurer's gain by 7.5 dollars per person when all states switch from explicit to
presumed consent.
Regarding the estimate of the size of the impact, some words of caution are in order.
We do not take supply side responses into account. It is possible that the brand name drug
producers decrease the price of their drugs in states with a presumed consent law. Therefore,
the impact of the presumed consent law would be smaller compared to our calculations.
Derivation of the Welfare Formula
This expected surplus is a very simple expression in the context of the logit model, which
is called the log-sum. We will demonstrate this fact in the context of two alternatives. With
two alternatives, the values ϵ1 of ϵ2 and can be depicted in a plane. This plane contains all
the possible combinations of (ϵ1, ϵ2). Some of them leads to the choice of alternative 1, and
others to the choice of alternative 2. More precisely, alternative 1 is chosen if ϵ2 < V1−V2+ϵ1,
and alternative 2 is chosen if ϵ1 < V2 − V1 + ϵ2.
We can rewrite the expected utility as the sum of the two terms E1and E2, with:
(V1 + ϵ1)f (ϵ1)f (ϵ2)dϵ1dϵ2
(V2 + ϵ2)f (ϵ1)f (ϵ2)dϵ1dϵ2
where f (z) = exp(−e−z) is the density of the Gumbell distribution.
We derive the expression for E1, and by symmetry we get the expression for E2. The
expected utility is obtained from summing E1and E2.
Abadie, A. and Gay, S.: 2006, The impact of presumed consent legislation on cadaveric
organ donation: a cross-country study, Journal of health economics 25(4), 599–620.
Bertrand, M., Duflo, E. and Mullainathan, S.: 2002, How much should we trust differences
in differences estimates?, Technical report, National Bureau of Economic Research.
Beshears, J., Choi, J. J., Laibson, D., Madrian, B. C. and Reynolds, G.: 2013, Testimoni-
als do not convert patients from brand to generic medication, The American journal of
managed care 19(9), e314.
Besley, T. and Case, A.: 1994, Unnatural experiments? estimating the incidence of endoge-
nous policies, Technical report, National Bureau of Economic Research.
Boehm, G., Yao, L., Han, L. and Zheng, Q.: 2013, Development of the generic drug industry
in the us after the hatch-waxman act of 1984, Acta Pharmaceutica Sinica B 3(5), 297–311.
Carone, G., Schwierz, C. and Xavier, A.: 2012, Cost-containment policies in public pharma-
ceutical spending in the eu, Available at SSRN 2161803 .
Carrera, M. and Villas-Boas, S.: 2013, Generic aversion and observational learning in the
over-thecounter drug market, Preliminary draft, April .
Carroll, G. D., Choi, J. J., Laibson, D., Madrian, B. and Metrick, A.: 2005, Optimal defaults
and active decisions, Technical report, National Bureau of Economic Research.
Chetty, R., Friedman, J. N., Leth-Petersen, S., Nielsen, T. and Olsen, T.: 2012, Active vs.
passive decisions and crowdout in retirement savings accounts: Evidence from denmark,
Technical report, National Bureau of Economic Research.
Chetty, R., Looney, A. and Kroft, K.: 2007, Salience and taxation: Theory and evidence,
Technical report, National Bureau of Economic Research.
Coscelli, A.: 2000, The importance of doctor and patients preferences in the prescription
decision, The Journal of Industrial Economics 48(3), 349–369.
Coscelli, A. and Shum, M.: 2004, An empirical model of learning and patient spillovers in
new drug entry, Journal of Econometrics 122(2), 213–246.
Crawford, G. S. and Shum, M.: 2005, Uncertainty and learning in pharmaceutical demand,
DellaVigna, S. and Pollet, J. M.: 2009, Investor inattention and friday earnings announce-
ments, The Journal of Finance 64(2), 709–749.
Goldman, D. P., Joyce, G. F. and Zheng, Y.: 2007, Prescription drug cost sharing: associa-
tions with medication and medical utilization and spending and health, Jama 298(1), 61–
Handel, B. R.: 2013, Adverse selection and inertia in health insurance markets: When
nudging hurts, The American Economic Review 103(7), 2643–2682.
Hellerstein, J. K.: 1998, The importance of the physician in the generic versus trade-name
prescription decision, The Rand journal of economics pp. 108–136.
Hortaçsu, A. and Syverson, C.: 2003, Product differentiation, search costs, and competition
in the mutual fund industry: a case study of the s&p 500 index funds, Technical report,
National Bureau of Economic Research.
Kelton, C. M., Chang, L. V. and Kreling, D. H.: 2013, State medicaid programs missed
220 million in uncaptured savings as generic fluoxetine came to market, Health Affairs
Ling, D. C., Berndt, E. R. and Kyle, M. K.: 2002, Deregulating direct-to-consumer marketing
of prescription drugs: Effects on prescription and over-the-counter product sales*, Journal
of Law and Economics 45(S2), 691–723.
Lundin, D.: 2000, Moral hazard in physician prescription behavior, Journal of health eco-
nomics 19(5), 639–662.
Madrian, B. C. and Shea, D. F.: 2000, The power of suggestion: Inertia in 401 (k) partici-
pation and savings behavior, Technical report, National bureau of economic research.
Petrin, A. and Train, K.: 2010, A control function approach to endogeneity in consumer
choice models, Journal of Marketing Research 47(1), 3–13.
Rosen, H. S. and Small, K. A.: 1979, Applied welfare economics with discrete choice models.
Shrank, W. H., Choudhry, N. K., Agnew-Blais, J., Federman, A. D., Liberman, J. N., Liu, J.,
Kesselheim, A. S., Brookhart, M. A. and Fischer, M. A.: 2010, State generic substitution
laws can lower drug outlays under medicaid, Health affairs 29(7), 1383–1390.
Shrank, W. H., Liberman, J. N., Fischer, M. A., Avorn, J., Kilabuk, E., Chang, A., Kessel-
heim, A. S., Brennan, T. A. and Choudhry, N. K.: 2011, The consequences of requesting
dispense as written, The American journal of medicine 124(4), 309–317.
Simonsen, M., Skipper, L. and Skipper, N.: 2015, Price sensitivity of demand for prescription
drugs: Exploiting a regression kink design, Journal of Applied Econometrics .
Sinkinson, M. and Starc, A.: 2015, Ask your doctor? direct-to-consumer advertising of
pharmaceuticals, Technical report, National Bureau of Economic Research.
Smith, M. D. and Brynjolfsson, E.: 2001, Consumer decision-making at an internet shopbot.
Sullivan, D. L., Birdwell, S. W. and Kucukarslan, S. N.: 1994, An assessment of consumer
purchasing behavior for private-label vs. brand-name over-the-counter products in chain
pharmacies, Journal of Pharmaceutical Marketing & Management 8(1), 85–108.
Thaler, G.: 2008, Nudge: Improving decisions about health, wealth, and happiness: By
richard h. thaler, cass r. sunstein.
Thaler, R. H. and Benartzi, S.: 2004, Save more tomorrow: Using behavioral economics to
increase employee saving, Journal of political Economy 112(S1), S164–S187.
Villas-Boas, J. M. and Winer, R. S.: 1999, Endogeneity in brand choice models, Management
Science 45(10), 1324–1338.
Vogler, S.: 2012, The impact of pharmaceutical pricing and reimbursement policies on gener-
ics uptake: implementation of policy options on generics in 29 european countries–an
overview, Generics and Biosimilars Initiative Journal 1(2), 44–51.
Source: http://yansong.org/pdf/YanSong-JMP_Nov9.pdf
8722 • The Journal of Neuroscience, September 24, 2003 • 23(25):8722– 8732 Brain-Derived Neurotrophic Factor Modulation of GABAergic Synapses by Postsynaptic Regulation of Rinda A. Wardle1,2 and Mu-ming Poo11Division of Neurobiology, Department of Molecular and Cell Biology, University of California, Berkeley, California 94720-3200, and 2Division of Biology,University of California at San Diego, La Jolla, California 92093-0357
Pal iative Medicine 2004; 18: 195¡/201 Haloperidol in palliative careJane Vella-Brincat and AD (Sandy) Macleod Nurse Maude Hospice, Christchurch Haloperidol is one of 20 'essential‘ medications in palliative care. Its use is widespread inpalliative care patients. The pharmacology of haloperidol is complex and the extent andseverity of some of its adverse effects, particularly extrapyramidal adverse effects (EPS),may be related to the route of administration. Indications for the use of haloperidol inpalliative care are nausea and vomiting and delirium. Adverse effects include EPS and QTprolongation. Sedation is not a common adverse effect of haloperidol. It is important thatpalliative care practitioners have a comprehensive understanding of the indications, doses,adverse effects and pharmacology of haloperidol. This review is intended to address theseissues. Pal iative Medicine 2004; 18: 195¡/201